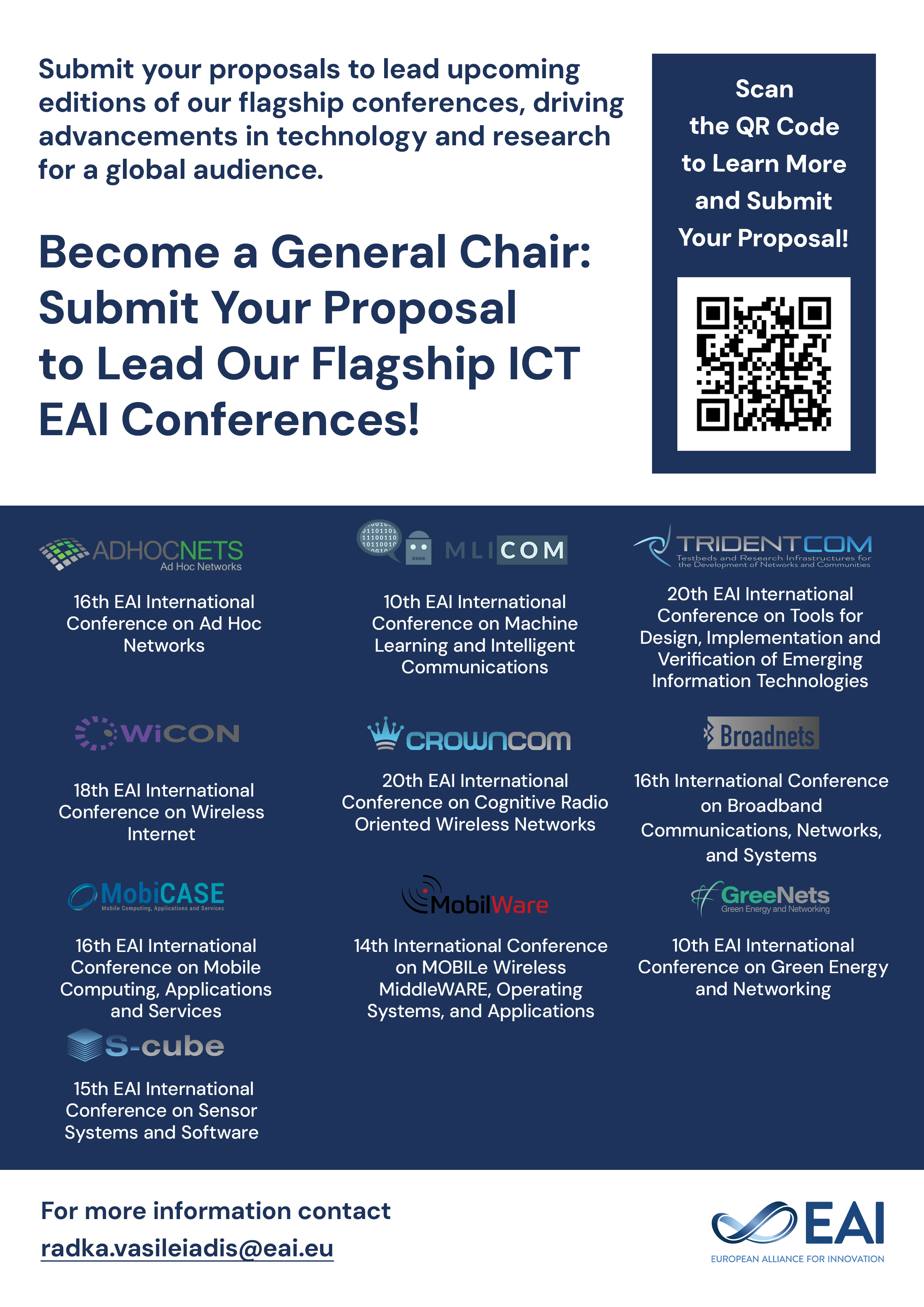
Research Article
Fairness Guarantees Under Demographic Shift
@INPROCEEDINGS{10.4108/eai.12-1-2024.2347147, author={Shengxin Zhang and Ruiyang Wang and Yushan Li and Jiarui Yang and Ruichao Zhuang}, title={Fairness Guarantees Under Demographic Shift}, proceedings={Proceedings of the 3rd International Conference on Big Data Economy and Digital Management, BDEDM 2024, January 12--14, 2024, Ningbo, China}, publisher={EAI}, proceedings_a={BDEDM}, year={2024}, month={6}, keywords={machine learning fairness demographic parity sensitive}, doi={10.4108/eai.12-1-2024.2347147} }
- Shengxin Zhang
Ruiyang Wang
Yushan Li
Jiarui Yang
Ruichao Zhuang
Year: 2024
Fairness Guarantees Under Demographic Shift
BDEDM
EAI
DOI: 10.4108/eai.12-1-2024.2347147
Abstract
Contemporary research has established that machine learning applications, especially in social contexts, can inadvertently lead to unfair model predictions that manifest as racism, sexism, or discrimination. Such biases stem from factors such as population growth and economic changes. Typically, a model is trained and later deployed to predict relevant problems. However, this approach usually assumes that the training dataset is reflective of the data expected in real-world deployment. As the distribution shift caused by demographic changes remains unknown, Giguere, Metevier et al. utilized a student t-test to compute the upper confidence bound. However, we identified several areas of potential improvement within this algorithm. In this paper, we propose methods to optimize the Shifty Algorithm by enhancing its robustness and refining its loss function. To evaluate the performance of the modified Shifty Algorithm, we used the UCI Adult Census dataset and a real-world dataset on university admissions exams and subsequent student achievement. Through these experiments, we demonstrate that models trained using our method successfully mitigate bias when faced with demographic shifts. Our experimental results validate the robust fairness assurances of our algorithm under real-world conditions. Moreover, they highlight the ability of the new Shifty Algorithm to train models effectively, ensuring fairness in the event of demographic shifts, while making fewer assumptions.