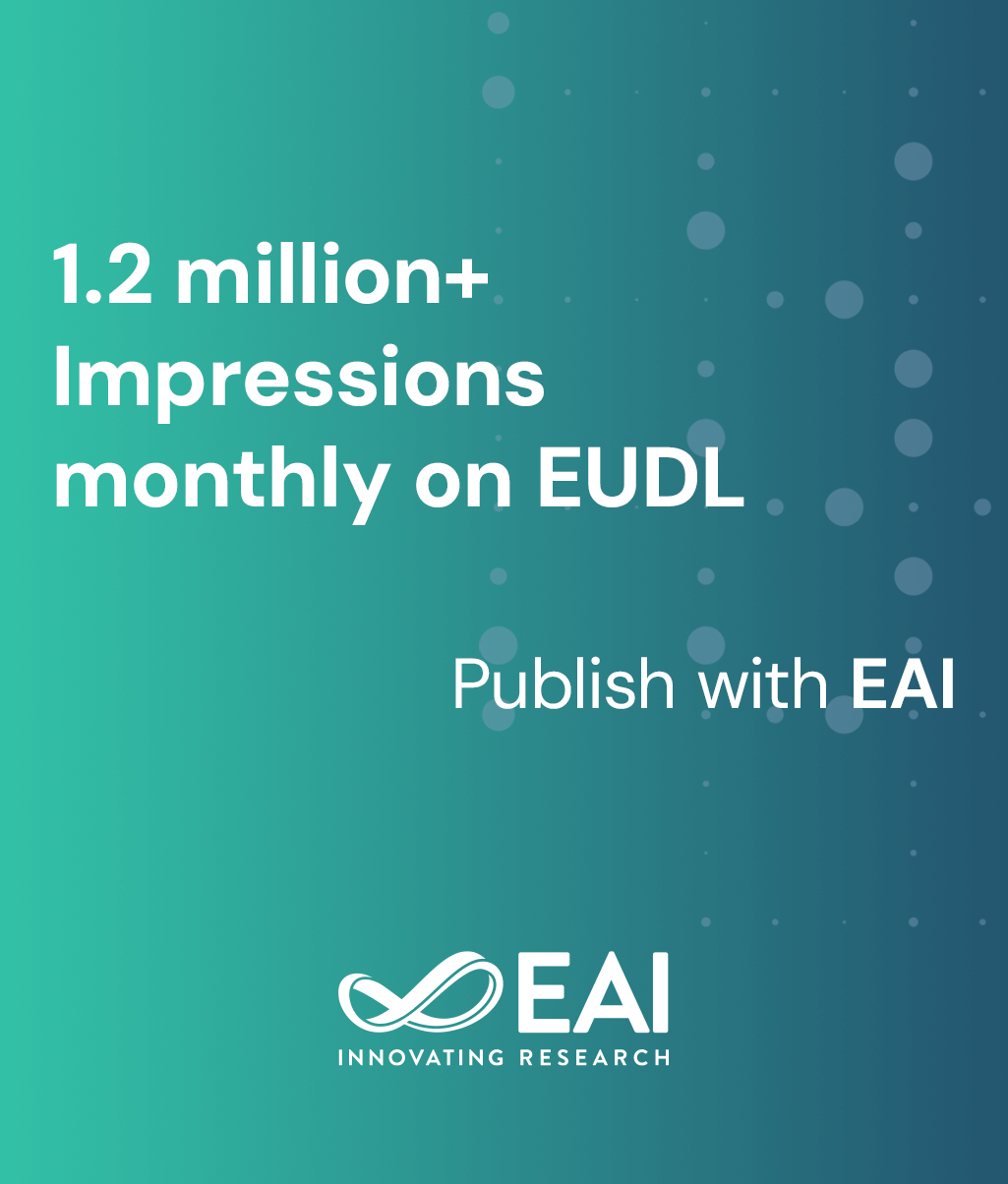
Research Article
Recognising Activities in Real Time Using Body Worn Passive Sensors With Sparse Data Streams: To Interpolate or Not To Interpolate?
@INPROCEEDINGS{10.4108/eai.11-8-2015.151111, author={Asanga Wickramasinghe and Damith C. Ranasinghe}, title={Recognising Activities in Real Time Using Body Worn Passive Sensors With Sparse Data Streams: To Interpolate or Not To Interpolate?}, proceedings={12th EAI International Conference on Mobile and Ubiquitous Systems: Computing, Networking and Services}, publisher={ICST}, proceedings_a={MOBIQUITOUS}, year={2016}, month={3}, keywords={Experimentation Algorithms}, doi={10.4108/eai.11-8-2015.151111} }
- Asanga Wickramasinghe
Damith C. Ranasinghe
Year: 2016
Recognising Activities in Real Time Using Body Worn Passive Sensors With Sparse Data Streams: To Interpolate or Not To Interpolate?
MOBIQUITOUS
ICST
DOI: 10.4108/eai.11-8-2015.151111
Abstract
Recent emergence of small, lightweight, batteryless (passive), and therefore maintenance free, wearable computing platforms such as sensor enabled RFID (Radio Frequency Identication) tags provide new opportunities for low cost and unobtrusive activity monitoring. Unfortunately, data streams from passive sensors are uniquely characterised by sparsity and noise. Consequently, readily extracting features that require a data stream with a regular sampling rate, such as those based on frequency domain transformations and auto regressive coecients are challenging. In this study, we propose an approach that reduces online interpolation errors to facilitate interpolating sparse acceleration data streams from a passive RFID tag with an on-board accelerometer sensor. We demonstrate using two datasets from older people that incorporating features extracted from interpolated acceleration data depicts a signicant performance improvement compared with incorporating features possible from only raw acceleration data. However, this is achieved at the expense of a real-time prediction delay (>400%) due to preprocessing involved. Furthermore, we demonstrate that for these types of sensors, features readily available from a typical RFID platform can be successfully used instead of features extracted from an interpolated data stream to achieve similar or better activity recognition performance without preprocessing and, whilst, using signicantly less number of features.