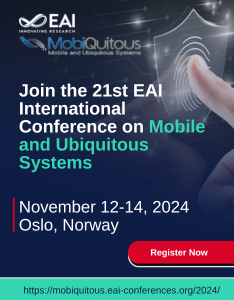
Research Article
IOTA Based Anomaly Detection Machine learning in Mobile Sensing
@ARTICLE{10.4108/eai.11-1-2022.172814, author={Muhammad Shoaib Akhtar and Tao Feng}, title={IOTA Based Anomaly Detection Machine learning in Mobile Sensing}, journal={EAI Endorsed Transactions on Creative Technologies}, volume={9}, number={30}, publisher={EAI}, journal_a={CT}, year={2022}, month={1}, keywords={machine learning, deep learning, deep neural network, anomaly detection}, doi={10.4108/eai.11-1-2022.172814} }
- Muhammad Shoaib Akhtar
Tao Feng
Year: 2022
IOTA Based Anomaly Detection Machine learning in Mobile Sensing
CT
EAI
DOI: 10.4108/eai.11-1-2022.172814
Abstract
In this proposed method, iMCS can detect and prevent fake sensing activities of mobile users using machine learning techniques. Our iMCS solution uses behavioral analysis based on participants' reliability scores to detect variation in behavior of users and introduces a new role in a distributed system of MCS architecture to validate the collected data. To evaluate the incentive based on the participant's sensory data and data quality, to properly distribute profit among the participants, we employ the Shapley Value approach. The evaluation results demonstrate that our method is effective in both quality estimations and incentive sharing.
Copyright © 2022 Muhammad Shoaib Akhtar et al., licensed to EAI. This is an open access article distributed under the terms of the Creative Commons Attribution license, which permits unlimited use, distribution and reproduction in any medium so long as the original work is properly cited.