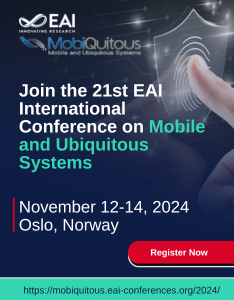
Research Article
Threat Modeling for Cloud Infrastructures
@ARTICLE{10.4108/eai.10-1-2019.156246, author={Nawaf Alhebaishi and Lingyu Wang and Anoop Singhal}, title={Threat Modeling for Cloud Infrastructures}, journal={EAI Endorsed Transactions on Security and Safety}, volume={5}, number={17}, publisher={EAI}, journal_a={SESA}, year={2018}, month={12}, keywords={Threat Modeling, Cloud Infrastructure, Attack Surface, Attack Tree, Attack Graph, Security Metrics, Network Hardening}, doi={10.4108/eai.10-1-2019.156246} }
- Nawaf Alhebaishi
Lingyu Wang
Anoop Singhal
Year: 2018
Threat Modeling for Cloud Infrastructures
SESA
EAI
DOI: 10.4108/eai.10-1-2019.156246
Abstract
Today’s businesses are increasingly relying on the cloud as an alternative IT solution due to its flexibility and lower cost. Compared to traditional enterprise networks, a cloud infrastructure is typically much larger and more complex. Understanding the potential security threats in such infrastructures is naturally more challenging than in traditional networks. This is evidenced by the fact that there are limited efforts on threat modeling for cloud infrastructures. In this paper, we conduct comprehensive threat modeling exercises based on two representative cloud infrastructures using several popular threat modeling methods, including attack surface, attack trees, attack graphs, and security metrics based on attack trees and attack graphs, respectively. Those threat modeling efforts may provide cloud providers useful lessons toward better understanding and improving the security of their cloud infrastructures. In addition, we show how hardening solution can be applied based on the threat models and security metrics through extended exercises. Such results may not only benefit the cloud provider but also embed more confidence in cloud tenants by providing them a clearer picture of the potential threats and mitigation solutions.
Copyright © 2018 Nawaf Alhebaishi et al., licensed to EAI. This is an open access article distributed under the terms of the Creative Commons Attribution license (http://creativecommons.org/licenses/by/3.0/), which permits unlimited use, distribution and reproduction in any medium so long as the original work is properly cited.