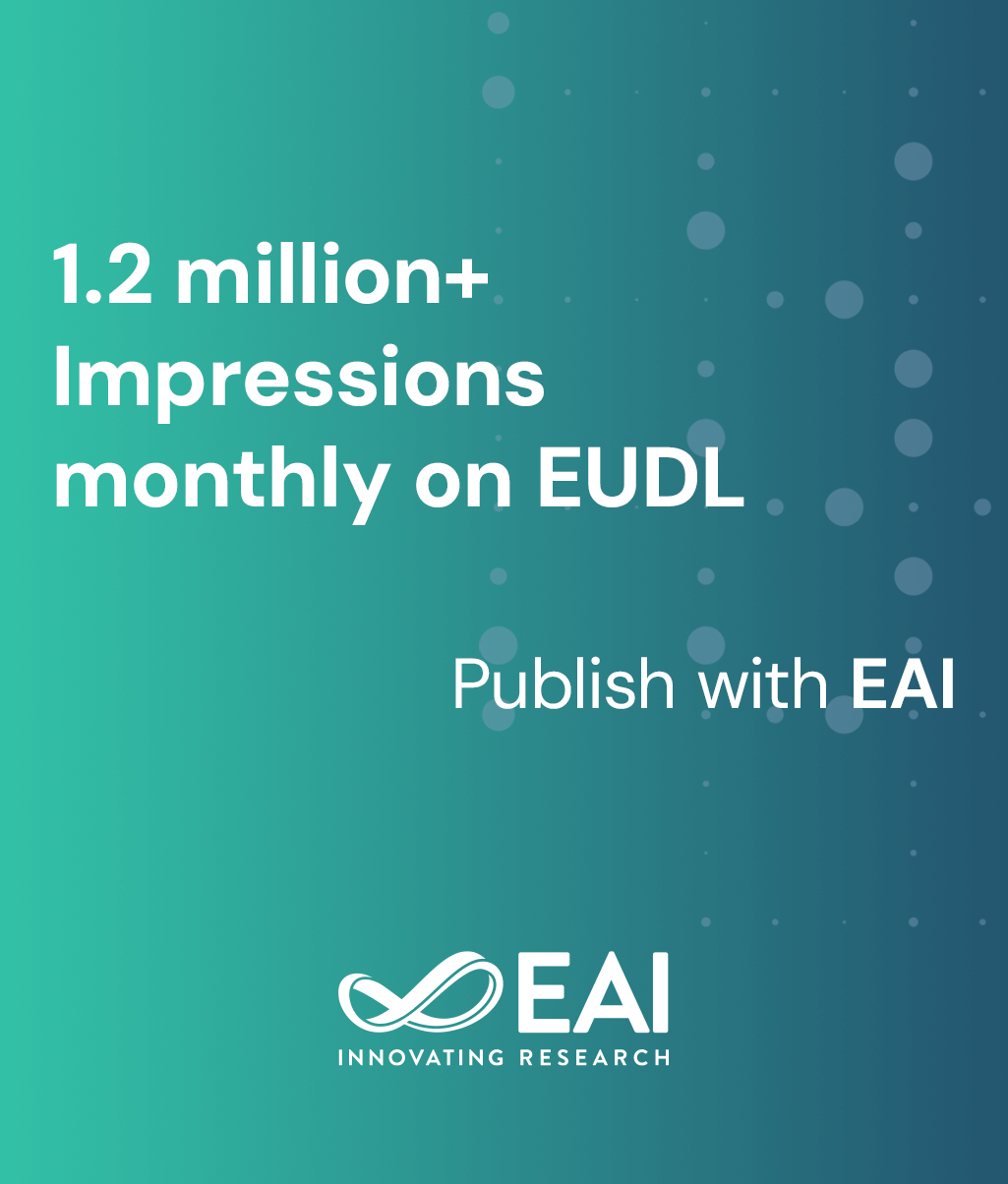
Research Article
Financial Risks Identification Model based on Reinforcement Learning
@INPROCEEDINGS{10.4108/eai.1-9-2023.2338766, author={Shu Chen}, title={Financial Risks Identification Model based on Reinforcement Learning}, proceedings={Proceedings of the 2nd International Conference on Public Management, Digital Economy and Internet Technology, ICPDI 2023, September 1--3, 2023, Chongqing, China}, publisher={EAI}, proceedings_a={ICPDI}, year={2023}, month={11}, keywords={financial risks identification risk feature extraction reinforcament learning classification accuracy}, doi={10.4108/eai.1-9-2023.2338766} }
- Shu Chen
Year: 2023
Financial Risks Identification Model based on Reinforcement Learning
ICPDI
EAI
DOI: 10.4108/eai.1-9-2023.2338766
Abstract
At present, financial risks have become an important problem for companies due to the large amount of transcation data and the uncontrolled transcation behaviours. Therefore, identifying the risks is essential for companies to know the possible crisis and provide corrsponding measures, which also plays an irreplaceable component for marketing and economic development. However, existing identification methods are concentrated on using mathematical models and analyzing the correlations among risk factors, which can not satisfy large amount of financial data and complex risks relationships. In this work, we initally attempt to establish a learning model to extract the features of financial risks. Subsequently, a reinforcement learning model is trained to classify risks and non-risks data with enough training iterations. Finally, we evaluate the proposed model with real-life data-set and compare with existing risk identification models to measure the performance of proposed model with multi-levels metrics. From our experimental results, we can observe that our model can achieve the identification of risks data with acceptable accuracy and reasonable computation costs.