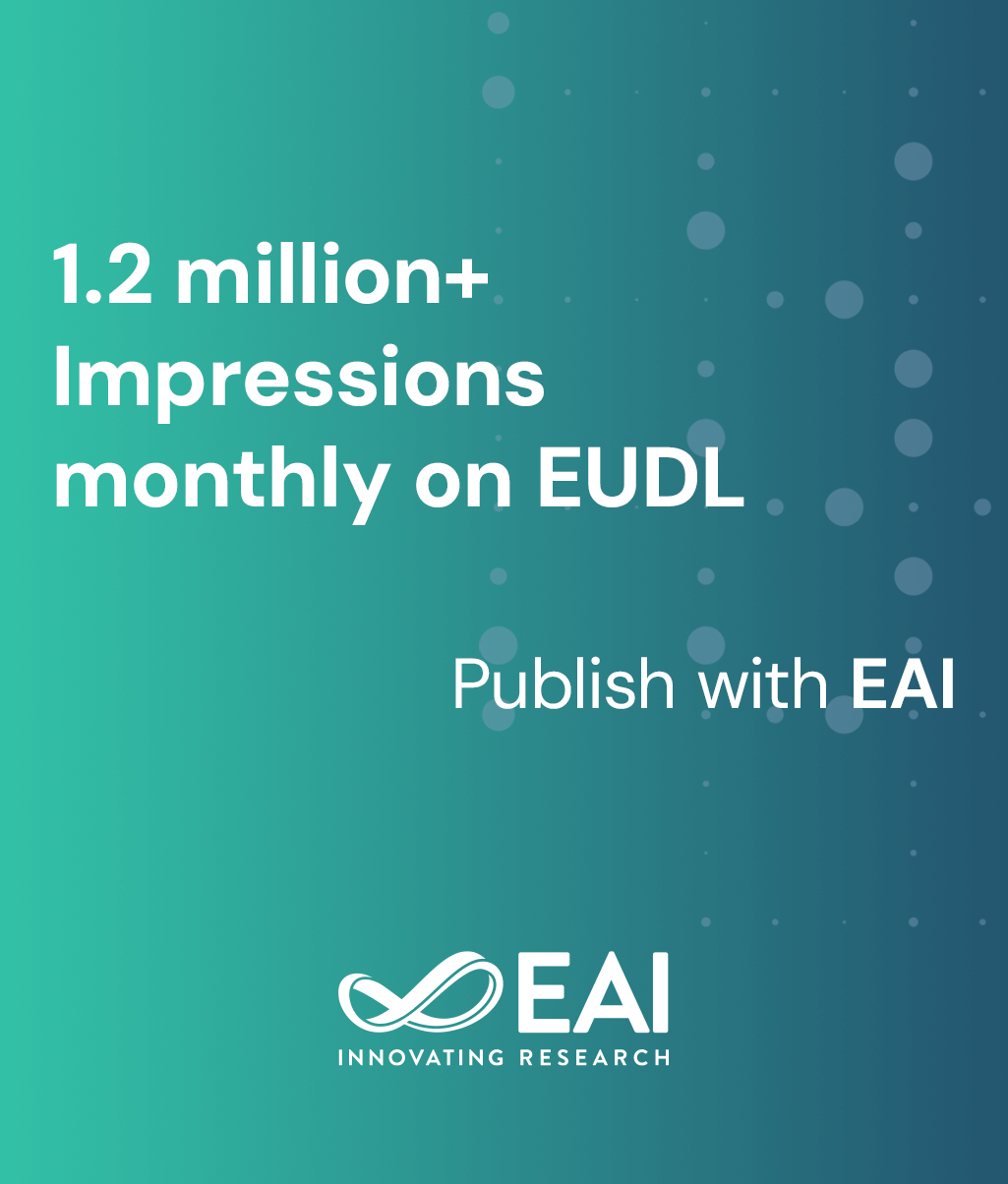
Research Article
Evaluation and Prediction of Enterprise Safety Management Efficiency Based on DEA-BP Neural Networks
@INPROCEEDINGS{10.4108/eai.1-9-2023.2338749, author={Lixing Zhu and Jie Xiao and Tiancheng Wang and Jinhai Tang and Chengyifan Li and Junjie Yan}, title={Evaluation and Prediction of Enterprise Safety Management Efficiency Based on DEA-BP Neural Networks}, proceedings={Proceedings of the 2nd International Conference on Public Management, Digital Economy and Internet Technology, ICPDI 2023, September 1--3, 2023, Chongqing, China}, publisher={EAI}, proceedings_a={ICPDI}, year={2023}, month={11}, keywords={enterprise security management efficiency evaluation;data envelopment analysis (dea);back propagation neural network (bpnn)}, doi={10.4108/eai.1-9-2023.2338749} }
- Lixing Zhu
Jie Xiao
Tiancheng Wang
Jinhai Tang
Chengyifan Li
Junjie Yan
Year: 2023
Evaluation and Prediction of Enterprise Safety Management Efficiency Based on DEA-BP Neural Networks
ICPDI
EAI
DOI: 10.4108/eai.1-9-2023.2338749
Abstract
The evaluation and prediction of enterprise security management efficiency is an important and challenging topic. In this study, we use a hybrid model of Data Envelopment Analysis (DEA) and Back Propagation Neural Network (BPNN) to evaluate and predict the efficiency of enterprise safety management. This model considers multiple input variables, including security inputs, number of employees, and enterprise size, and is trained and tested on a virtual enterprise dataset. The results show that all the input variables affect the efficiency of an enterprise's security management to varying degrees, with the effects of security inputs and number of employees being particularly significant. Through principal component analysis, we further visualized the relationship between input variables and efficiency values in a two-dimensional space. The results of this study have important theoretical and practical implications for understanding and improving enterprise safety management, as well as providing a new perspective to explore the complexity of this field.