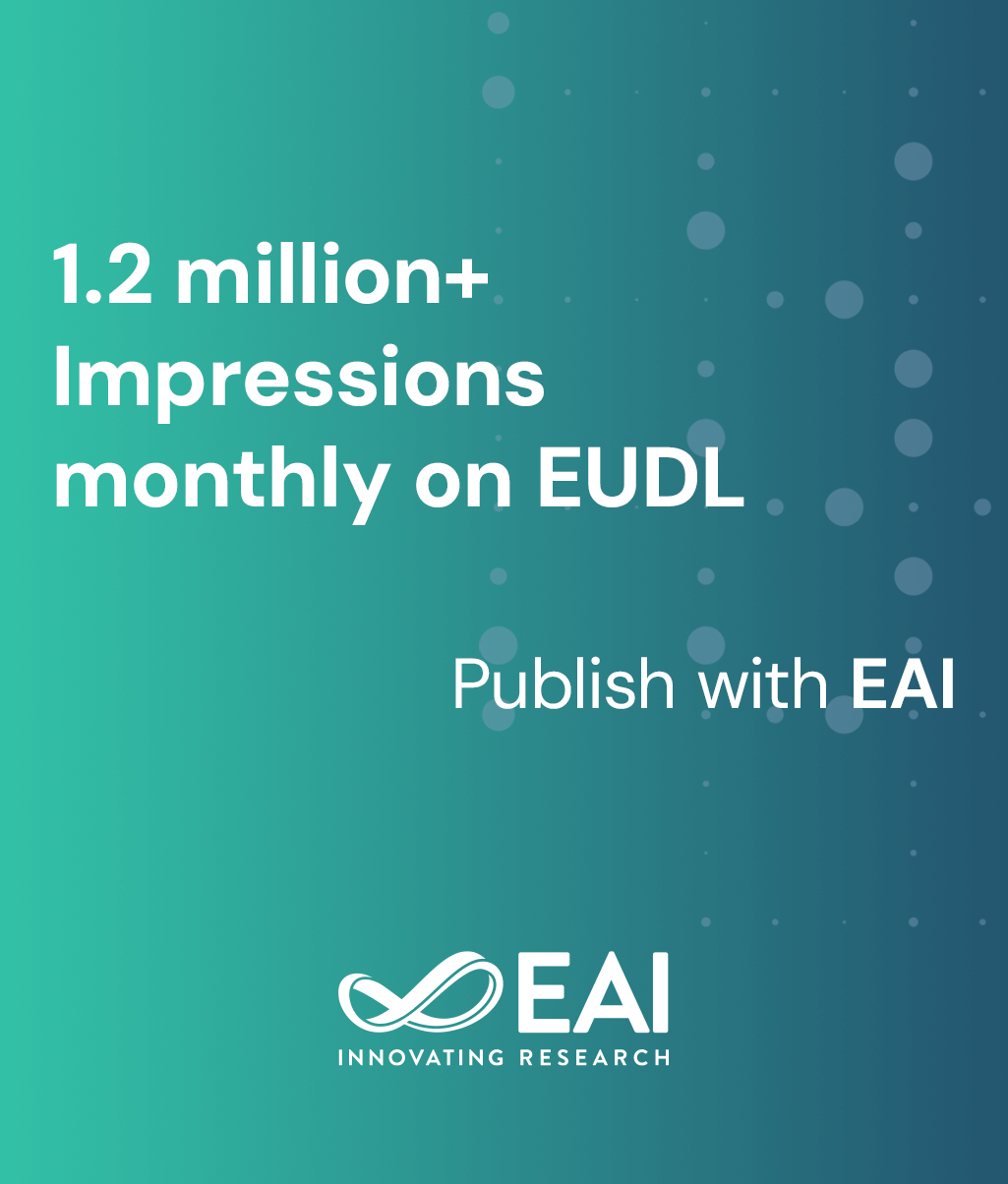
Research Article
Global Green GDP Forecasting Model Based on BP Neural Network
@INPROCEEDINGS{10.4108/eai.1-9-2023.2338703, author={Wei Zhang and Yimou Wang and Xinyu Li and Jiajun Lei}, title={Global Green GDP Forecasting Model Based on BP Neural Network}, proceedings={Proceedings of the 2nd International Conference on Public Management, Digital Economy and Internet Technology, ICPDI 2023, September 1--3, 2023, Chongqing, China}, publisher={EAI}, proceedings_a={ICPDI}, year={2023}, month={11}, keywords={combination weight leslie model neural network climate change}, doi={10.4108/eai.1-9-2023.2338703} }
- Wei Zhang
Yimou Wang
Xinyu Li
Jiajun Lei
Year: 2023
Global Green GDP Forecasting Model Based on BP Neural Network
ICPDI
EAI
DOI: 10.4108/eai.1-9-2023.2338703
Abstract
Multilateral developments would present a challenge to the global harmonization of green GDP as the fundamental criterion of economic health. Green GDP, on the other hand, considered environmental costs and sustainability and contributed to effective global climate crisis. mitigation in contrast to GDP, which was currently used to gauge national economic performance.We built a model of expected global climate mitigation based on BP neural networks. Secondly, to create climate evaluation indicators based on CO2 emissions and temperature variations, seven variables from four typical nations were integrated with GDP. The GGDP data for the USA was added to the model for validation utilizing the test set to examine the generalization capability of the BP neural network. It was discovered that the changing trend was essentially consistent, illustrating that the model had stability.