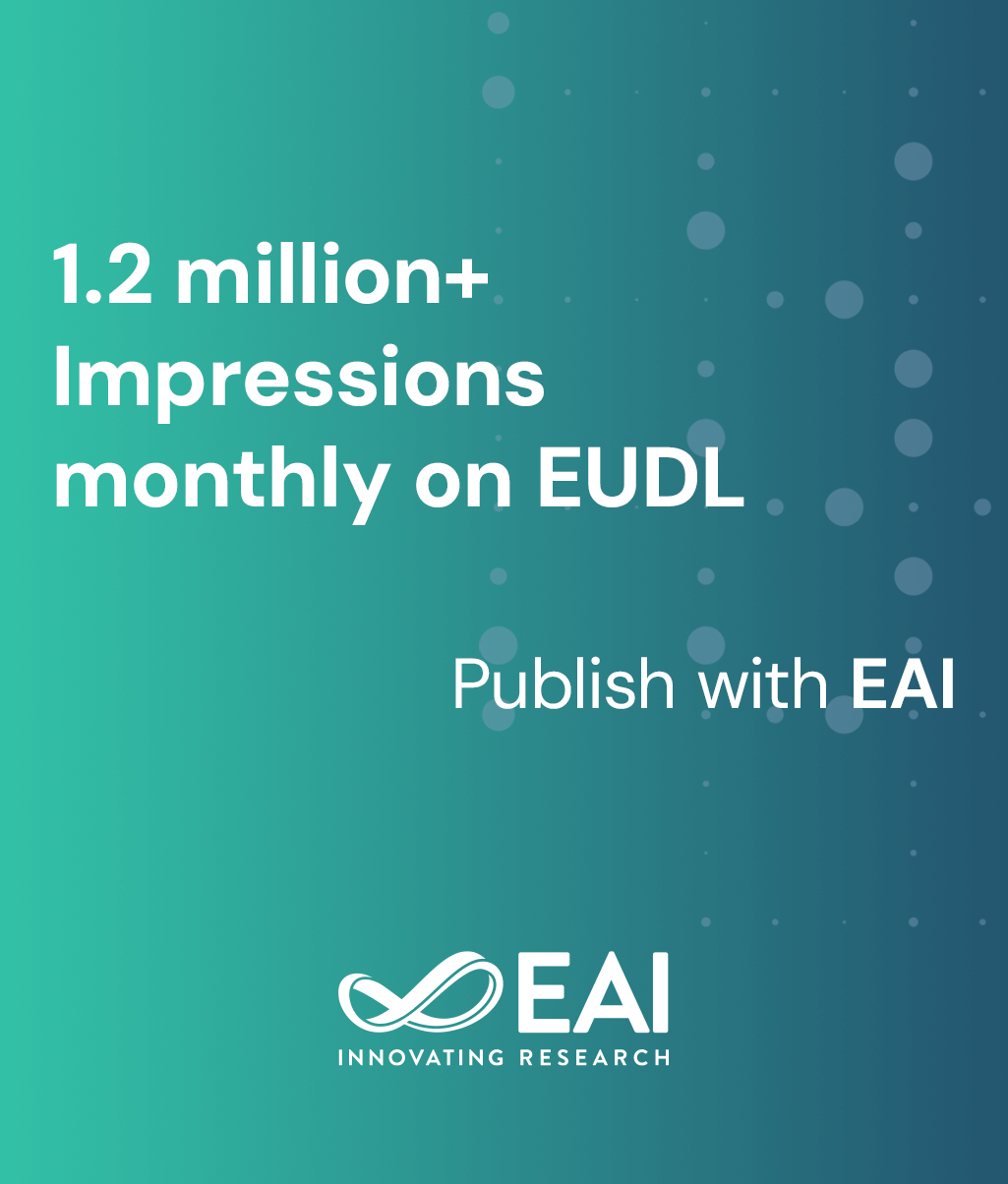
Research Article
Harnessing Context for Vandalism Detection in Wikipedia
@ARTICLE{10.4108/cc.1.1.e7, author={Lakshmish Ramaswamy and Raga Sowmya Tummalapenta and Deepika Sethi and Kang Li and Calton Pu}, title={Harnessing Context for Vandalism Detection in Wikipedia}, journal={EAI Endorsed Transactions on Collaborative Computing}, volume={1}, number={1}, publisher={ICST}, journal_a={CC}, year={2014}, month={5}, keywords={Collaborative Social Media, Vandalism, Content-context, Contributor-context}, doi={10.4108/cc.1.1.e7} }
- Lakshmish Ramaswamy
Raga Sowmya Tummalapenta
Deepika Sethi
Kang Li
Calton Pu
Year: 2014
Harnessing Context for Vandalism Detection in Wikipedia
CC
ICST
DOI: 10.4108/cc.1.1.e7
Abstract
The importance of collaborative social media (CSM) applications such as Wikipedia to modern free societies can hardly be overemphasized. By allowing end users to freely create and edit content, Wikipedia has greatly facilitated democratization of information. However, over the past several years, Wikipedia has also become susceptible to vandalism, which has adversely affected its information quality. Traditional vandalism detection techniques that rely upon simple textual features such as spammy or abusive words have not been very effective in combating sophisticated vandal attacks that do not contain common vandalism markers. In this paper, we propose a context-based vandalism detection framework for Wikipedia. We first propose a contextenhanced finite state model for representing the context evolution ofWikipedia articles. This paper identifies two distinct types of context that are potentially valuable for vandalism detection, namely content-context and contributor-context. The distinguishing powers of these contexts are discussed by providing empirical results. We design two novel metrics for measuring how well the content-context of an incoming edit fits into the topic and the existing content of a Wikipedia article. We outline machine learning-based vandalism identification schemes that utilize these metrics. Our experiments indicate that utilizing context can substantially improve vandalism detection accuracy.
Copyright © 2014 L. Ramaswamy , licensed to ICST. This is an open access article distributed under the terms of the Creative Commons Attribution license (http://creativecommons.org/licenses/by/3.0/), which permits unlimited use, distribution and reproduction in any medium so long as the original work is properly cited.