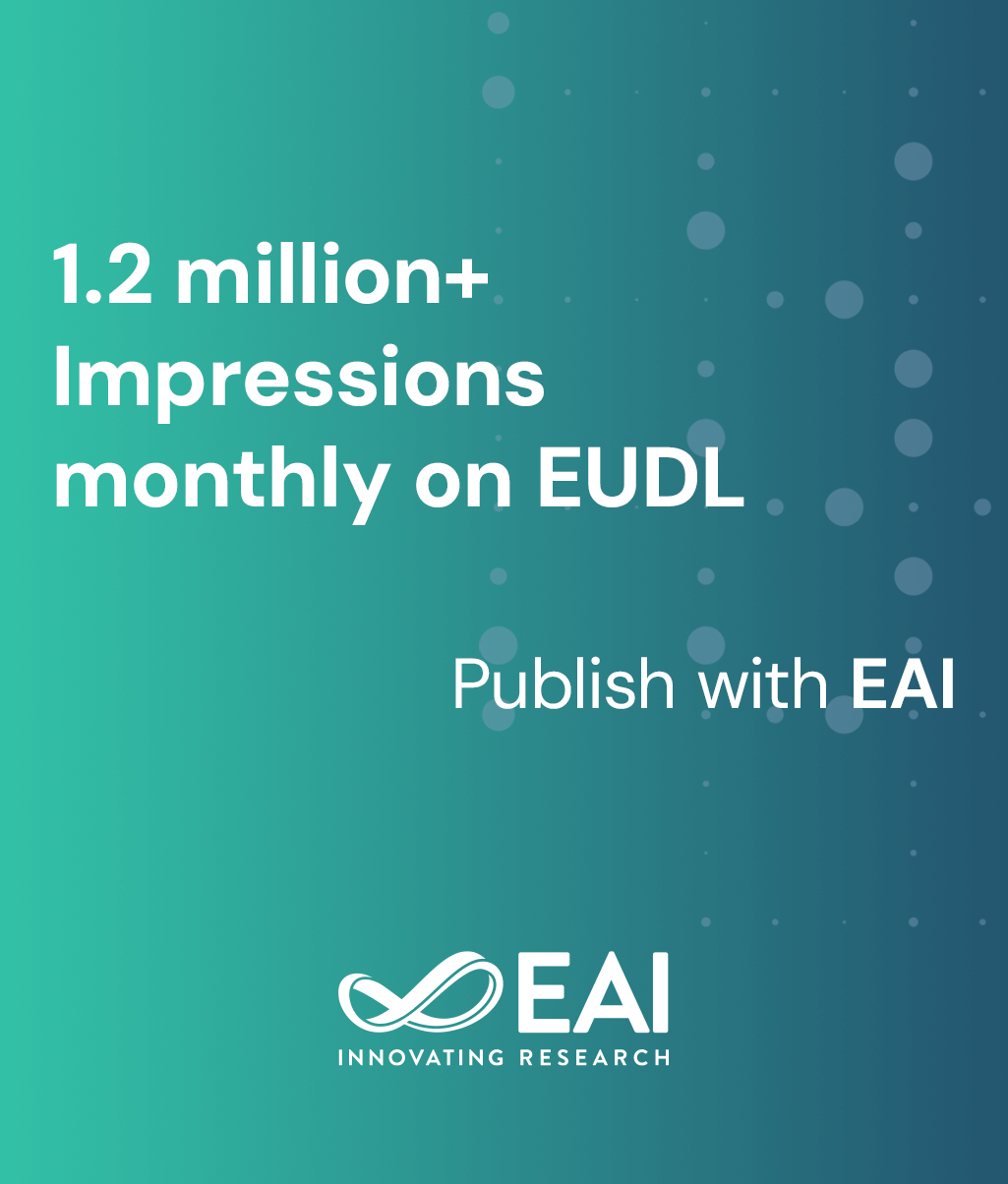
Research Article
Deep Learning Based Optical Character Recognition Model for Old Turkic
@ARTICLE{10.4108/airo.8460, author={Seyed Hossein Taheri and Houman Kosarirad and Isabel Adrover Gallego and Nedasadat Taheri}, title={Deep Learning Based Optical Character Recognition Model for Old Turkic}, journal={EAI Endorsed Transactions on AI and Robotics}, volume={4}, number={1}, publisher={EAI}, journal_a={AIRO}, year={2025}, month={4}, keywords={Deep learning, optical character recognition, convolutional neural network}, doi={10.4108/airo.8460} }
- Seyed Hossein Taheri
Houman Kosarirad
Isabel Adrover Gallego
Nedasadat Taheri
Year: 2025
Deep Learning Based Optical Character Recognition Model for Old Turkic
AIRO
EAI
DOI: 10.4108/airo.8460
Abstract
This study presents the development and evaluation of a deep learning-based optical character recognition (OCR) model specifically designed for recognizing Old Turkic script. Utilizing a convolutional neural network (CNN), the project aimed to achieve high classification accuracy across a dataset comprising 38 distinct Old Turkic characters. To enhance the model’s robustness and generalization capabilities, sophisticated data augmentation techniques were employed, generating 760 augmented images from the original 38 characters. The model was rigorously trained and validated, achieving an overall ac- curacy of 96.34%. Evaluation metrics such as precision, recall, and F1-scores were systematically analyzed, showing superior performance in most classes while identifying areas for further optimization. The results underscore the effectiveness of CNN architectures in specialized OCR tasks, demonstrating their potential in preserving and digitizing historical scripts. This study not only advances the field of document analysis and OCR but also contributes to the digital preservation and accessibility of ancient scripts.
Copyright © 2025 S. H. Taheri et al., licensed to EAI. This is an open access article distributed under the terms of the CC BY-NC-SA 4.0, which permits copying, redistributing, remixing, transformation, and building upon the material in any medium so long as the original work is properly cited.