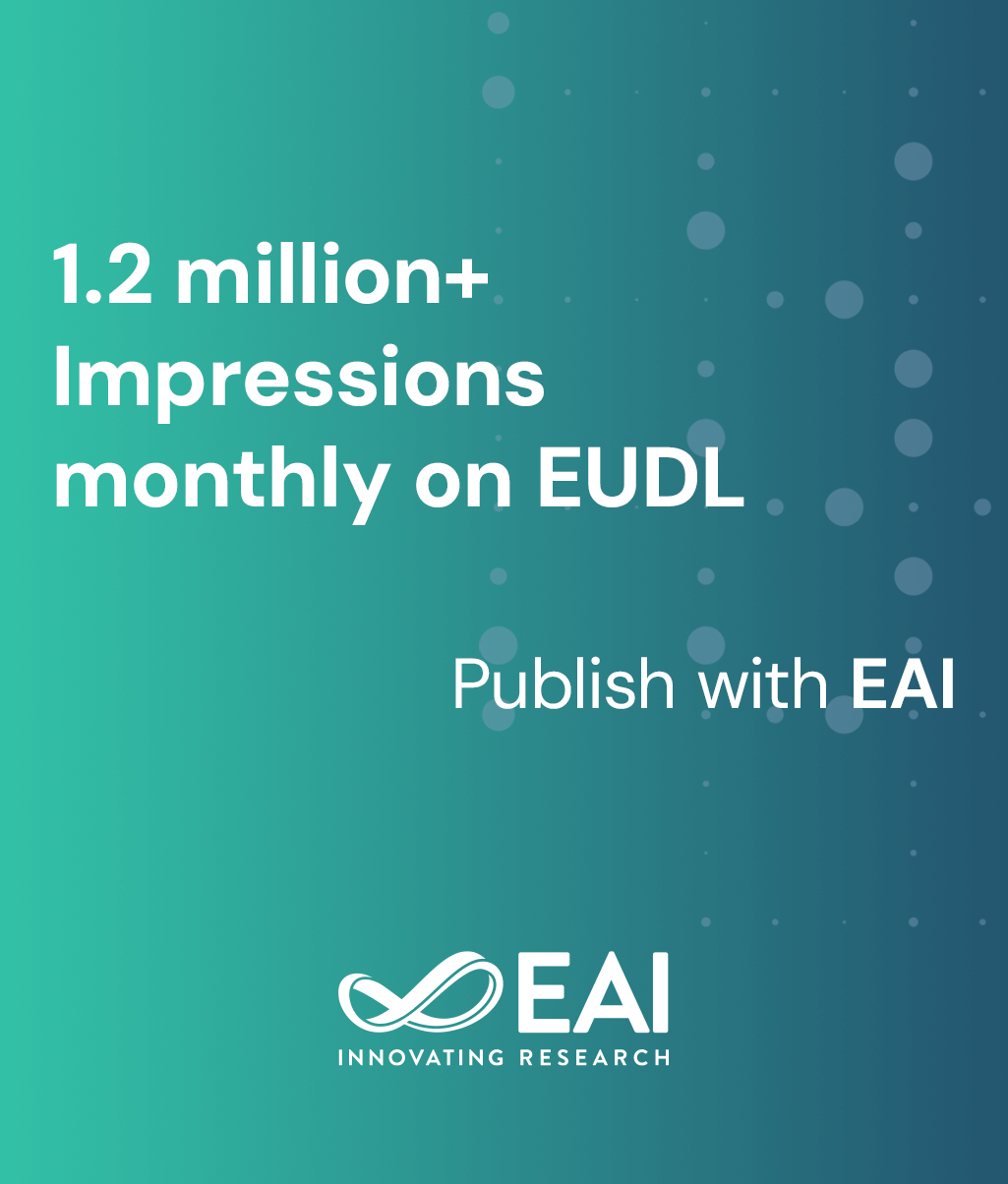
Research Article
From Social Media Reactions to Grades: A Machine Learning-Based SocialNet Analysis for Academic Performance Prediction
@ARTICLE{10.4108/airo.8171, author={Muhammad Ramzan and Naeem Ahmed}, title={From Social Media Reactions to Grades: A Machine Learning-Based SocialNet Analysis for Academic Performance Prediction}, journal={EAI Endorsed Transactions on AI and Robotics}, volume={4}, number={1}, publisher={EAI}, journal_a={AIRO}, year={2025}, month={3}, keywords={SocialNet Analysis, Machine Learning, Student Performance, Education, Learning Technologies}, doi={10.4108/airo.8171} }
- Muhammad Ramzan
Naeem Ahmed
Year: 2025
From Social Media Reactions to Grades: A Machine Learning-Based SocialNet Analysis for Academic Performance Prediction
AIRO
EAI
DOI: 10.4108/airo.8171
Abstract
The impact of social media on student academic performance has garnered significant research interest in recent years. The pervasive use of social networking sites (SNS) among college and university students, both in and outside classrooms, has raised concerns about its potential effects on academic achievement. This study investigates the relationship between social media usage and academic performance through a dataset of 550 participants. Machine learning models, including Random Forest, Decision Trees, and Long Short-Term Memory (LSTM), were employed to analyze and predict the impact of social media on students' academic outcomes. The models were trained using clean and well-engineered data. The results indicate a moderate influence of social media usage on academic performance, with the LSTM model outperforming traditional approaches in predictive accuracy. These findings highlight the importance of considering sequential usage patterns in understanding the academic implications of social media.
Copyright © 2025 M. Ramzan et al., licensed to EAI. This is an open access article distributed under the terms of the CC BY-NC-SA 4.0, which permits copying, redistributing, remixing, transformation, and building upon the material in any medium so long as the original work is properly cited.