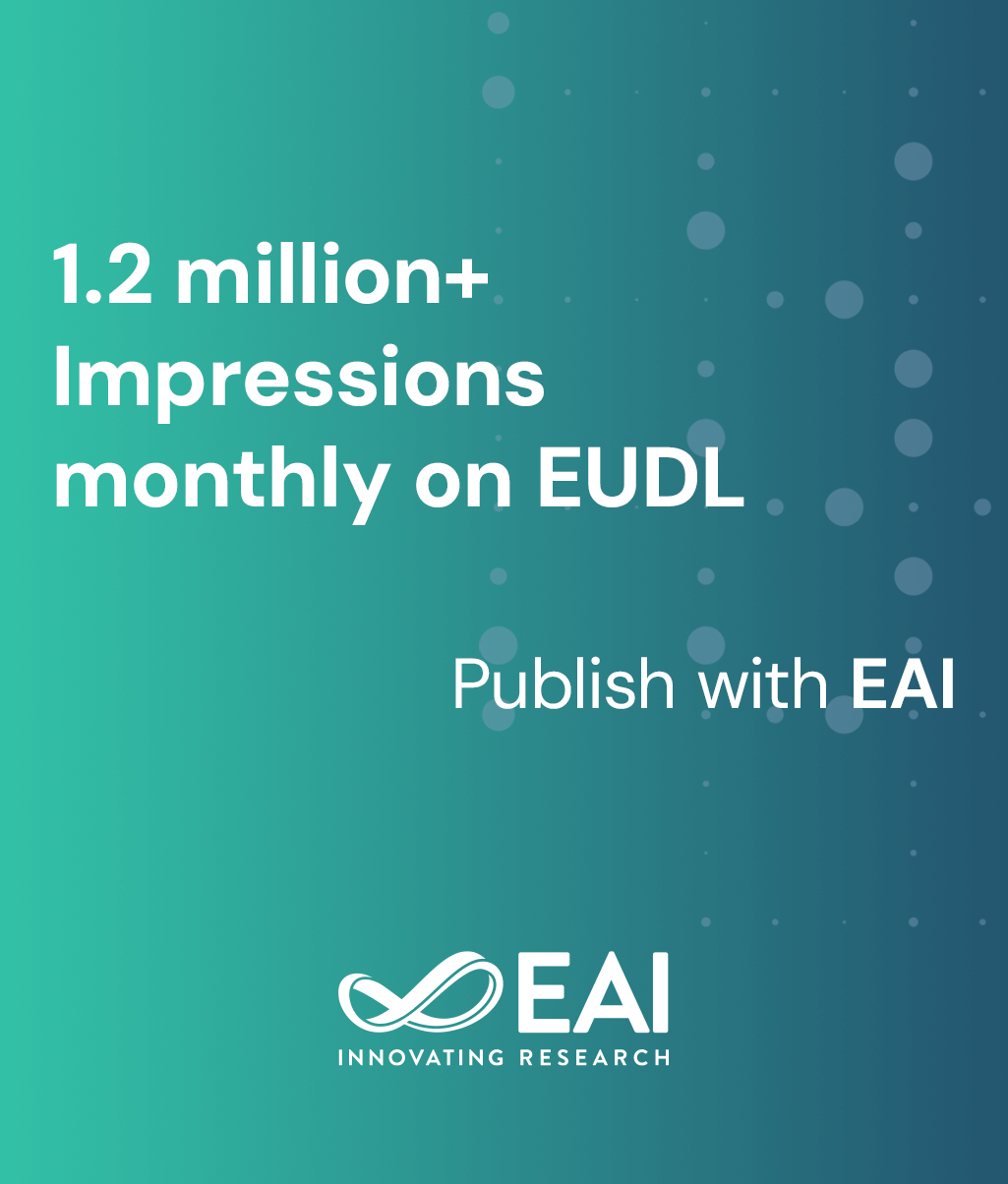
Research Article
Advancing Food Security through Precision Agriculture: YOLOv8’s Role in Efficient Pest Detection and Management
@ARTICLE{10.4108/airo.8049, author={Ameer Tamoor Khan and Sign Marie Jensen and Noman Khan}, title={Advancing Food Security through Precision Agriculture: YOLOv8’s Role in Efficient Pest Detection and Management}, journal={EAI Endorsed Transactions on AI and Robotics}, volume={4}, number={1}, publisher={EAI}, journal_a={AIRO}, year={2025}, month={4}, keywords={Sustainable Agriculture, Pest Detection, YOLOv8, Computer Vision, Object Detection, Crop Management, Deep Learning}, doi={10.4108/airo.8049} }
- Ameer Tamoor Khan
Sign Marie Jensen
Noman Khan
Year: 2025
Advancing Food Security through Precision Agriculture: YOLOv8’s Role in Efficient Pest Detection and Management
AIRO
EAI
DOI: 10.4108/airo.8049
Abstract
In response to the growing global population and the consequent need for sustainable food security, effective pest management is critical for enhancing agricultural productivity. This research presents YOLOv8, a state-of-the-art deep learning model optimized for pest detection in agricultural environments, contributing to modern food security efforts. Evaluated using the complex IP102 dataset, YOLOv8 demonstrated notable improvements in pest detection accuracy, achieving scores of 66.9 mAP@0.5 and 42.1 mAP@[0.5:0.95]. These results underscore YOLOv8’s robust performance across diverse detection scenarios, enabling more precise pest control and reducing crop loss. However, in-depth dataset analysis revealed a bias towards larger pests, likely due to bounding box size variations, which presents an opportunity for model improvement. Future work will focus on addressing data imbalances, enhancing sensitivity to smaller pests, and validating YOLOv8 in varied real-world agricultural settings. These advancements are expected to significantly improve pest management practices, ultimately boosting agricultural productivity and supporting global food security through the application of modern agricultural technologies.
Copyright © 2025 A. T. Khan et al., licensed to EAI. This is an open access article distributed under the terms of the CC BY-NC-SA 4.0, which permits copying, redistributing, remixing, transformation, and building upon the material in any medium so long as the original work is properly cited.