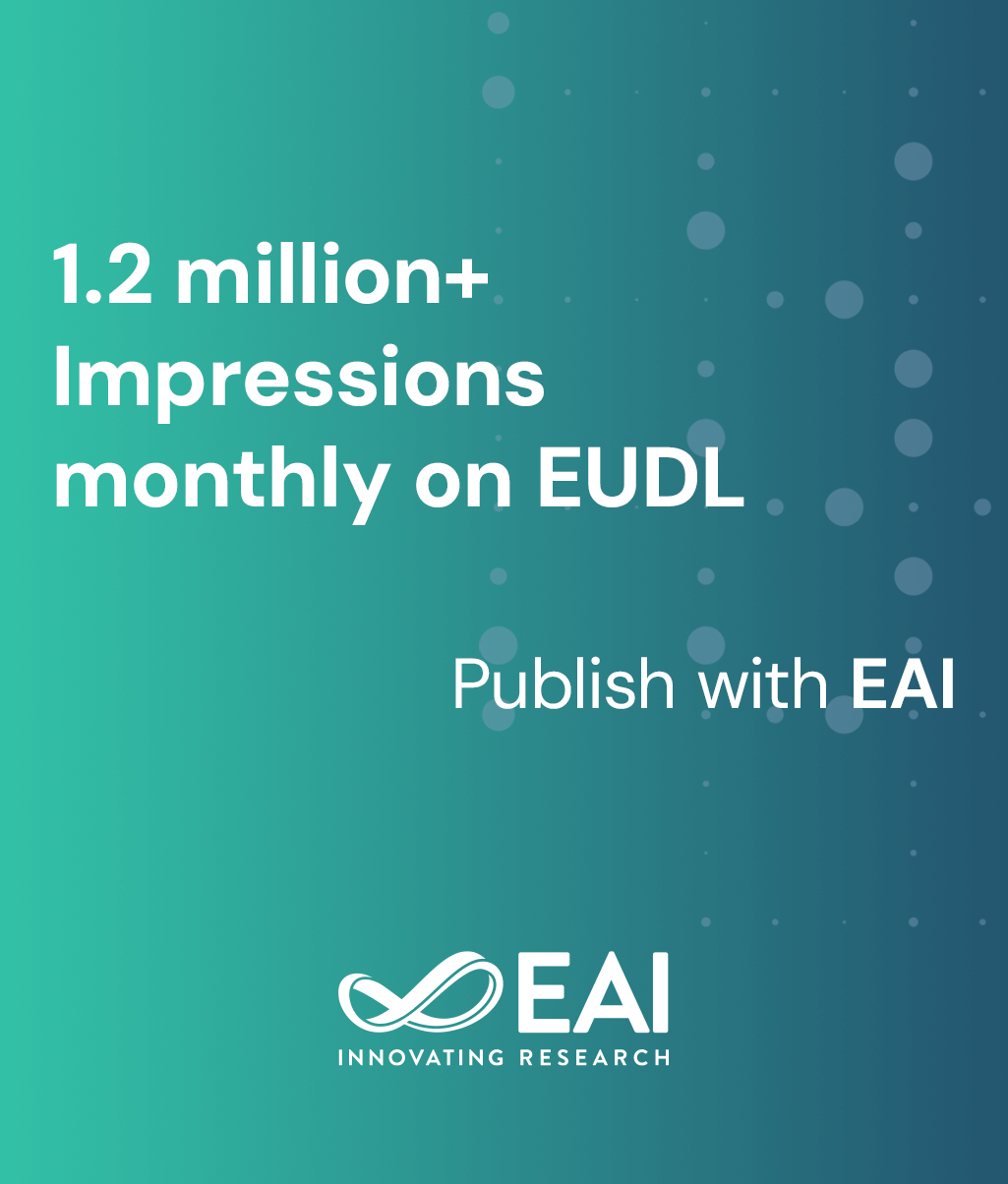
Research Article
Apple Disease Detection and Classification using Random Forest (One-vs-All)
@ARTICLE{10.4108/airo.8041, author={Zengming Wen and Hong Lan and Muhammad Asim Khan}, title={Apple Disease Detection and Classification using Random Forest (One-vs-All)}, journal={EAI Endorsed Transactions on AI and Robotics}, volume={4}, number={1}, publisher={EAI}, journal_a={AIRO}, year={2025}, month={4}, keywords={Fruit diseases detection, digital image processing, GLCM feature extraction, LDA, random forest}, doi={10.4108/airo.8041} }
- Zengming Wen
Hong Lan
Muhammad Asim Khan
Year: 2025
Apple Disease Detection and Classification using Random Forest (One-vs-All)
AIRO
EAI
DOI: 10.4108/airo.8041
Abstract
Fruit diseases detection and recognition are a common problem worldwide. Fruit Disease detection is a hot topic among researchers and is very complex due to structure and color similarity factors. In this research we proposed a new model to detect and classify the apple disease with the help of digital image processing and machine learning. First, image processing techniques were applied to enhance the image contrast and remove the noise, which helped to segment the region of interest accurately also help to extract feature without garbage data. Then K-means clustering technique with fuzzy C-mean method was implemented to segment the images. GLCM feature extraction was used after the segmentation. Real images of apple disease with multi-disease regions were used in the research method. These features were preprocessed with methods like LDA. K-Fold cross validation was used for training and testing, with combination of random forest machine learning method. The result showed high accuracy with comparison of existing techniques.
Copyright © 2025 Z. Wen et al., licensed to EAI. This is an open access article distributed under the terms of the CC BY-NC-SA 4.0, which permits copying, redistributing, remixing, transformation, and building upon the material in any medium so long as the original work is properly cited.