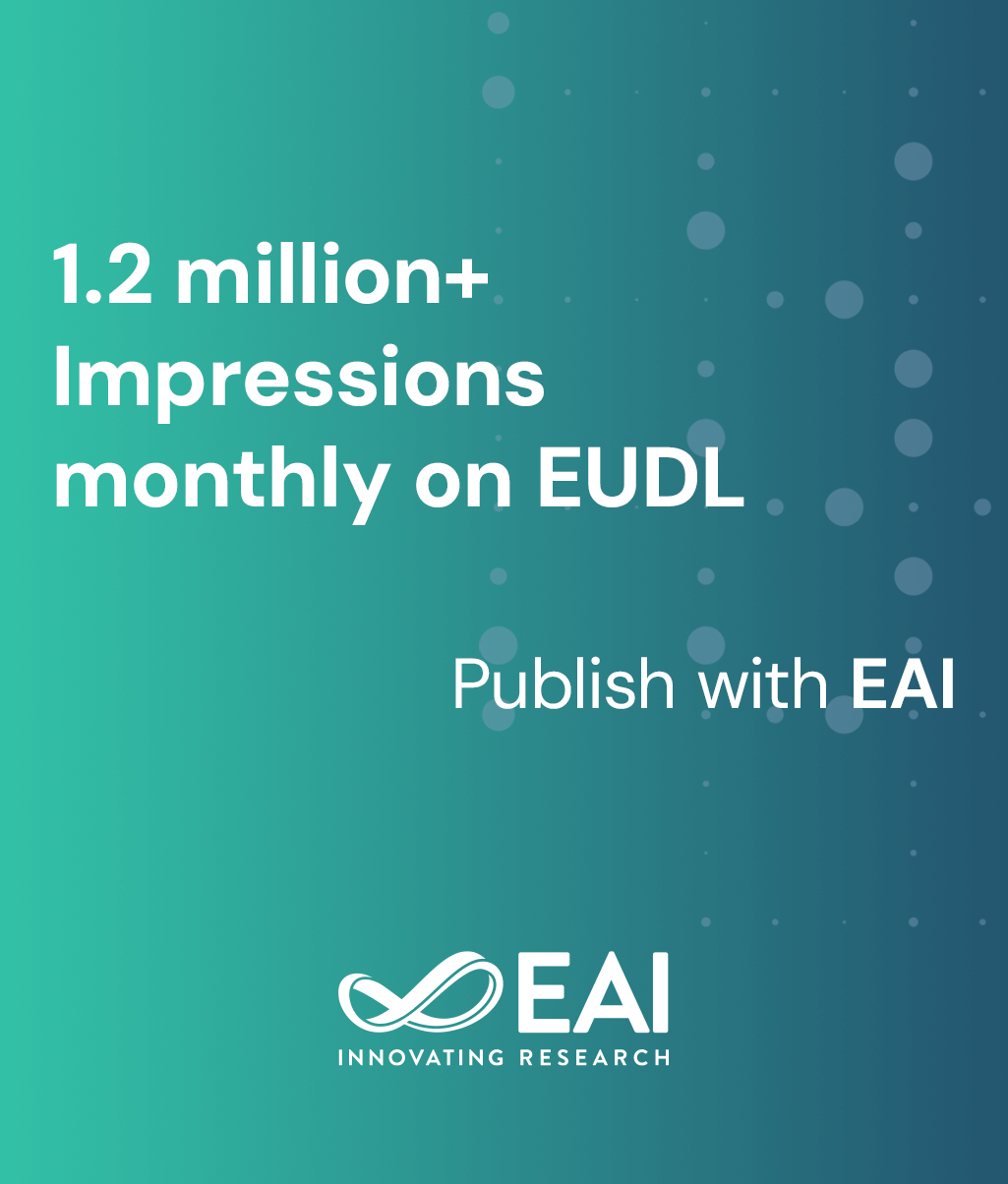
Research Article
A Hybrid Approach for Robust Object Detection: Integrating Template Matching and Faster R-CNN
@ARTICLE{10.4108/airo.6858, author={Hewa Majeed Zangana and Firas Mahmood Mustafa and Marwan Omar}, title={A Hybrid Approach for Robust Object Detection: Integrating Template Matching and Faster R-CNN}, journal={EAI Endorsed Transactions on AI and Robotics}, volume={3}, number={1}, publisher={EAI}, journal_a={AIRO}, year={2024}, month={10}, keywords={Computer Vision, Deep Learning, Hybrid Model, Object Detection, Template Matching.}, doi={10.4108/airo.6858} }
- Hewa Majeed Zangana
Firas Mahmood Mustafa
Marwan Omar
Year: 2024
A Hybrid Approach for Robust Object Detection: Integrating Template Matching and Faster R-CNN
AIRO
EAI
DOI: 10.4108/airo.6858
Abstract
Object detection is a critical task in computer vision, with applications ranging from autonomous vehicles to medical imaging. Traditional methods like template matching offer precise localization but struggle with variations in object appearance, while deep learning approaches such as Faster R-CNN excel in handling diverse and complex datasets but often require extensive computational resources and large amounts of labeled data. This paper proposes a hybrid approach that integrates template matching with Faster R-CNN to leverage the strengths of both techniques. By combining the accuracy of template matching with the robustness and generalization capabilities of Faster R-CNN, our method achieves superior performance in challenging scenarios, including objects with occlusions, varying scales, and complex backgrounds. Extensive experiments demonstrate that the hybrid model not only enhances detection accuracy but also reduces computational load, making it a practical solution for real-world applications.
Copyright © 2024 Zangana et al., licensed to EAI. This is an open access article distributed under the terms of the CC BY-NC-SA 4.0, which permits copying, redistributing, remixing, transformation, and building upon the material in any medium so long as the original work is properly cited.