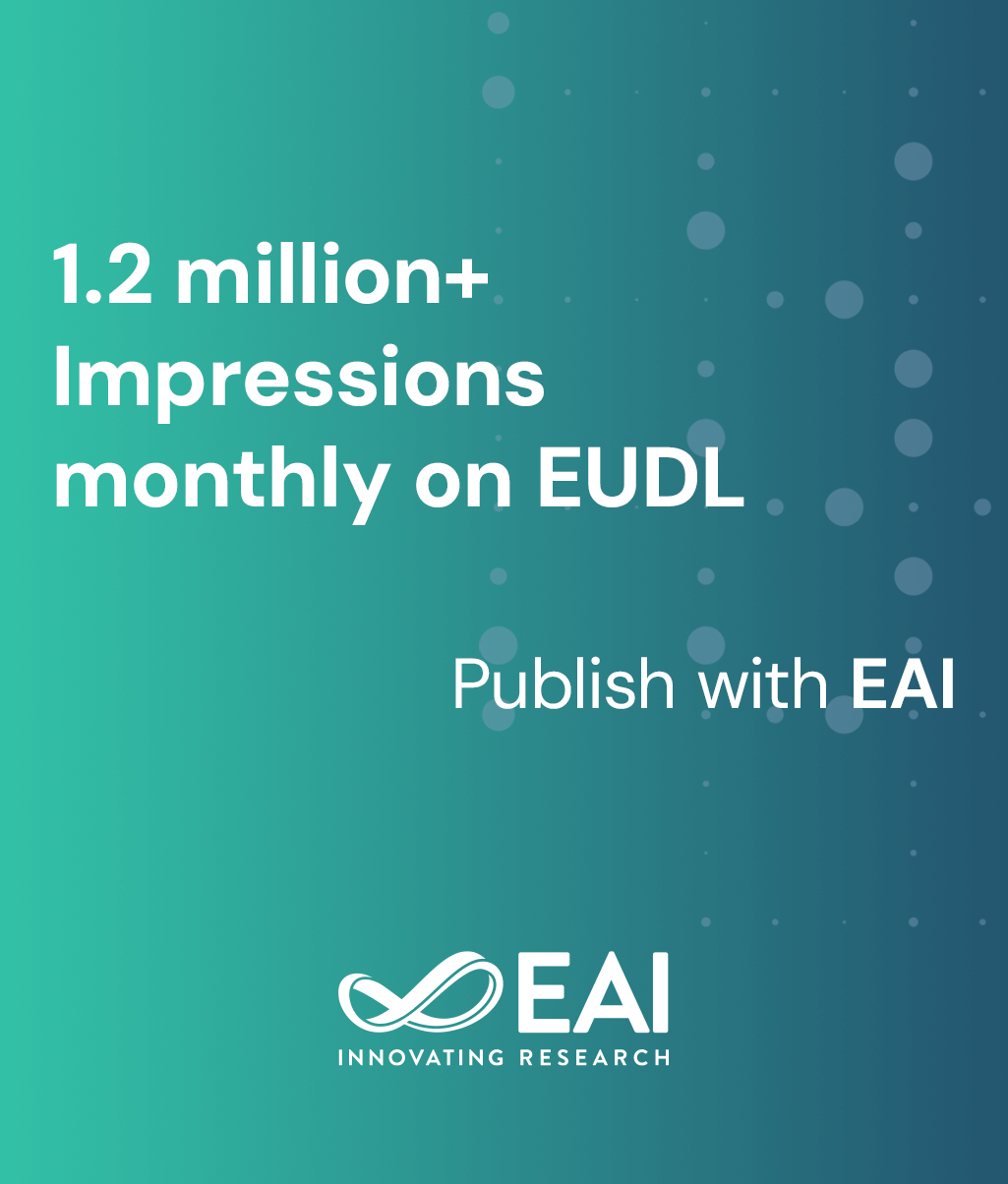
Research Article
A Comprehensive Survey of Text Encoders for Text-to-Image Diffusion Models
@ARTICLE{10.4108/airo.5566, author={Shun Fang}, title={A Comprehensive Survey of Text Encoders for Text-to-Image Diffusion Models}, journal={EAI Endorsed Transactions on AI and Robotics}, volume={3}, number={1}, publisher={EAI}, journal_a={AIRO}, year={2024}, month={12}, keywords={NLP, CLIP, T5-XXL, BERT, Text Encoder}, doi={10.4108/airo.5566} }
- Shun Fang
Year: 2024
A Comprehensive Survey of Text Encoders for Text-to-Image Diffusion Models
AIRO
EAI
DOI: 10.4108/airo.5566
Abstract
In this comprehensive survey, we delve into the realm of text encoders for text-to-image diffusion models, focusing on the principles, challenges, and opportunities associated with these encoders. We explore the state-of-the-art models, including BERT, T5-XXL, and CLIP, that have revolutionized the way we approach language understanding and cross-modal interactions. These models, with their unique architectures and training techniques, enable remarkable capabilities in generating images from textual descriptions. However, they also face limitations and challenges, such as computational complexity and data scarcity. We discuss these issues and highlight potential opportunities for further research. By providing a comprehensive overview, this survey aims to contribute to the ongoing development of text-to-image diffusion models, enabling more accurate and efficient image generation from textual inputs.
Copyright © 2024 Fang et al., licensed to EAI. This is an open access article distributed under the terms of the CC BY-NC-SA 4.0, which permits copying, redistributing, remixing, transformation, and building upon the material in any medium so long as the original work is properly cited.