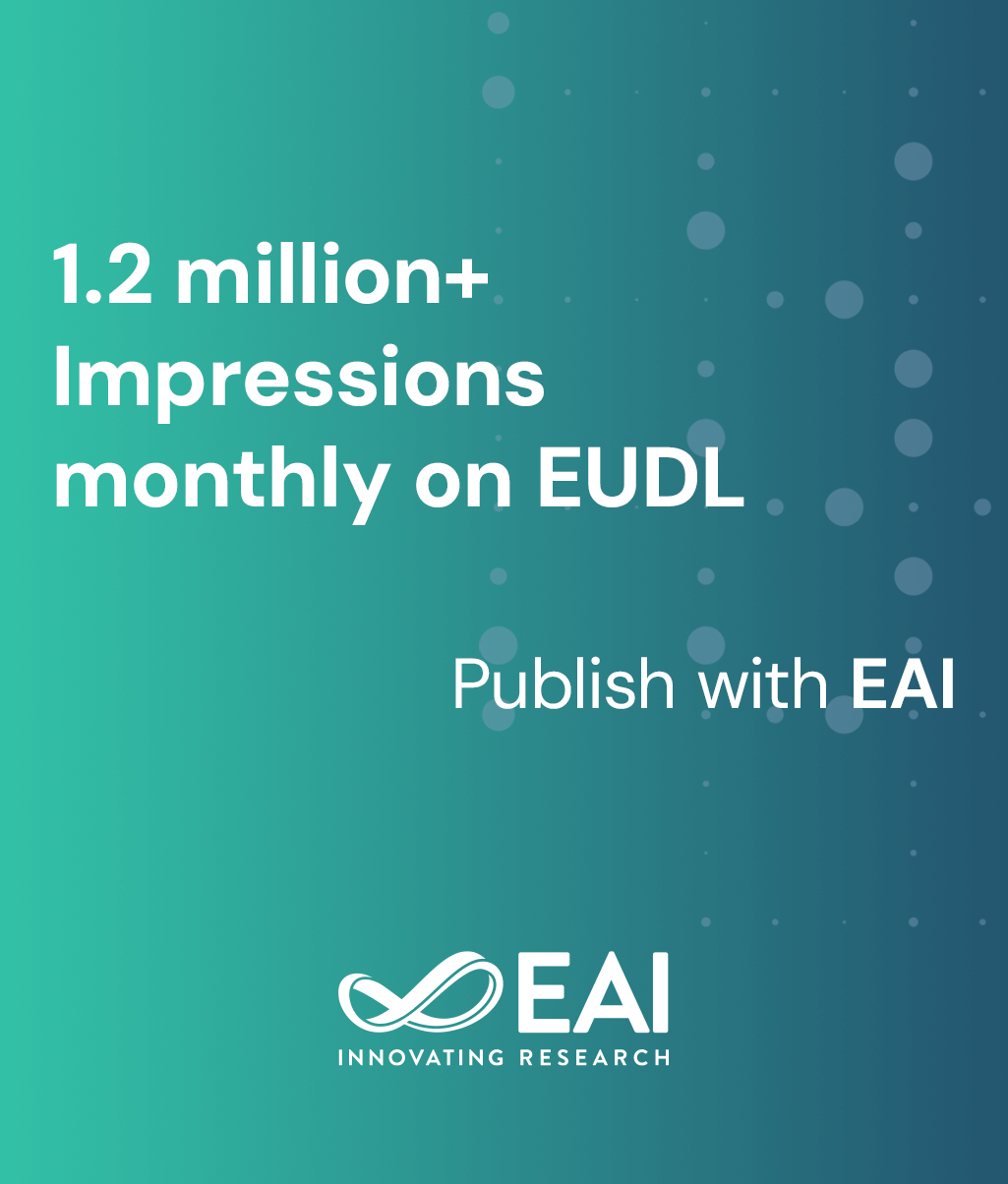
Research Article
Utilizing Fundamental Analysis to Predict Stock Prices
@ARTICLE{10.4108/airo.5140, author={Akshay Khanpuri and Narayana Darapaneni and Anwesh Reddy Paduri}, title={Utilizing Fundamental Analysis to Predict Stock Prices}, journal={EAI Endorsed Transactions on AI and Robotics}, volume={3}, number={1}, publisher={EAI}, journal_a={AIRO}, year={2024}, month={3}, keywords={Machine Learning, RNN, Long Short-Term Memory, LSTM, National Stock Exchange, NSE, Stock Prediction, Stock Selection, Performance Measures}, doi={10.4108/airo.5140} }
- Akshay Khanpuri
Narayana Darapaneni
Anwesh Reddy Paduri
Year: 2024
Utilizing Fundamental Analysis to Predict Stock Prices
AIRO
EAI
DOI: 10.4108/airo.5140
Abstract
Portfolio management involves the critical task of determining the optimal times to enter or exit a stock in order to maximize profits in the stock market. Unfortunately, many retail investors struggle with this task due to unclear investment objectives and a lack of a structured decision-making process. With the vast number of stocks available in the market, it can be difficult for investors to determine which stocks to invest in. As a result, there is a growing need for the development of effective investment decision support systems to assist investors in making informed decisions. Researchers have explored various approaches to building such systems, including predicting stock prices using sentiment analysis of news, articles, and social media, as well as historical trends and patterns. However, the impact of financial reports filed by companies on stock prices has not been extensively studied. This paper aims to address this gap by using machine learning techniques to develop a more accurate stock prediction model based on financial reports from companies in the Nifty 50. The financial reports considered include quarterly reports, annual reports, cash flow statements, and ratios.
Copyright © 2024 A. Khanapuri et al., licensed to EAI. This is an open access article distributed under the terms of the Creative Commons Attribution license (http://creativecommons.org/licenses/by/3.0/), which permits unlimited use, distribution and reproduction in any medium so long as the original work is properly cited.