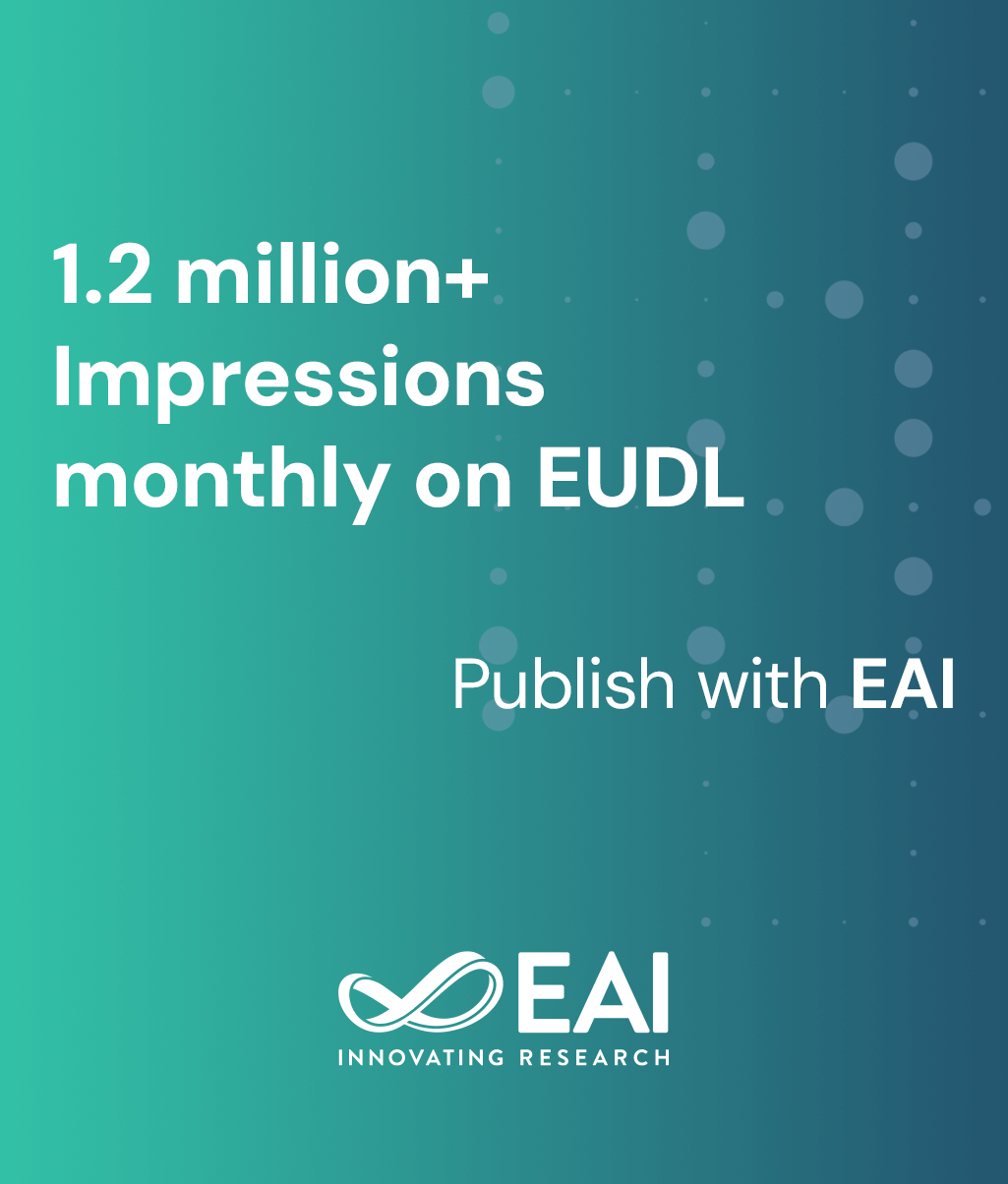
Research Article
Imbalanced Multiclass Medical Data Classification based on Learning Automata and Neural Network
@ARTICLE{10.4108/airo.3526, author={Masoumeh Soleimani and Zahra Forouzanfar and Morteza Soltani and Majid Jafari Harandi}, title={Imbalanced Multiclass Medical Data Classification based on Learning Automata and Neural Network}, journal={EAI Endorsed Transactions on AI and Robotics}, volume={2}, number={1}, publisher={EAI}, journal_a={AIRO}, year={2023}, month={7}, keywords={Classification, Imbalanced Data, Neural Network, Learning Automata}, doi={10.4108/airo.3526} }
- Masoumeh Soleimani
Zahra Forouzanfar
Morteza Soltani
Majid Jafari Harandi
Year: 2023
Imbalanced Multiclass Medical Data Classification based on Learning Automata and Neural Network
AIRO
EAI
DOI: 10.4108/airo.3526
Abstract
Data classification in the real world is often faced with the challenge of data imbalance, where there is a significant difference in the number of instances among different classes. Dealing with imbalanced data is recognized as a challenging problem in data mining, as it involves identifying minority-class data with a high number of errors. Therefore, the selection of unique and appropriate features for classifying data with smaller classes poses a fundamental challenge in this research. Nowadays, due to the widespread presence of imbalanced medical data in many real-world problems, the processing of such data has gained attention from researchers. The objective of this research is to propose a method for classifying imbalanced medical data. In this paper, the hypothyroidism dataset from the UCI repository is used. In the feature selection stage, a support vector machine algorithm is used as a cost function, and the wrapper algorithm is employed as a search strategy to achieve an optimal subset of features. The proposed method achieves high accuracy, reaching 99.6% accuracy for data classification through the optimization of a neural network using learning automata.
Copyright © 2023 Soleimani et al., licensed to EAI. This is an open access article distributed under the terms of the Creative Commons Attribution license (https://creativecommons.org/licenses/by-nc-sa/4.0/), which permits unlimited use, distribution and reproduction in any medium so long as the original work is properly cited.