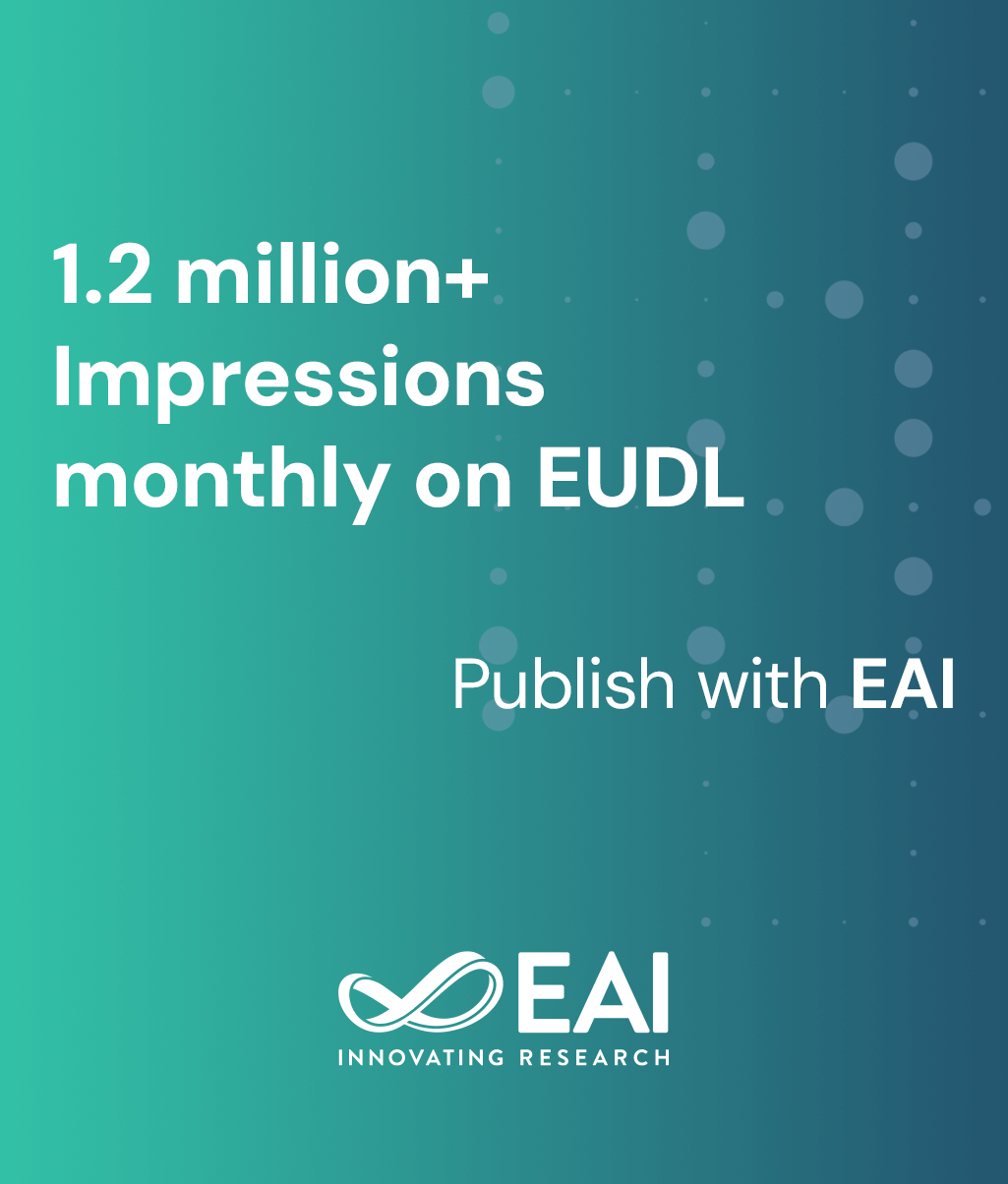
Research Article
Making Distributed Rate Control using Lyapunov Drifts a Reality in Wireless Sensor Networks
@INPROCEEDINGS{10.4108/ICST.WIOPT2008.3205, author={Avinash Sridharan and Scott Moeller and Bhaskar Krishnamachari}, title={Making Distributed Rate Control using Lyapunov Drifts a Reality in Wireless Sensor Networks}, proceedings={6th International ICST Symposium on Modeling and Optimization}, publisher={IEEE}, proceedings_a={WIOPT}, year={2008}, month={8}, keywords={Algorithm design and analysis Communication system control Distributed control Multiaccess communication Pressure control Protocols Stability Stochastic processes Testing Wireless sensor networks}, doi={10.4108/ICST.WIOPT2008.3205} }
- Avinash Sridharan
Scott Moeller
Bhaskar Krishnamachari
Year: 2008
Making Distributed Rate Control using Lyapunov Drifts a Reality in Wireless Sensor Networks
WIOPT
IEEE
DOI: 10.4108/ICST.WIOPT2008.3205
Abstract
We take a top-down approach of formulating the rate control problem, over a collection tree, in a wireless sensor network as a generic convex optimization problem and propose a distributed back pressure algorithm using Lyapunov drift based optimization techniques. Primarily, we show that existing theoretical results in the field of stochastic network optimization can be directly applied to a CSMA based wireless sensor network using our novel receiver capacity model. We back this claim by implementing our algorithm on the Tmote sky class devices. Our experimental evaluation on a 5 node testbed shows that the empirically observed rate allocation on a real sensor network testbed that uses our back pressure algorithm is close to the analytically predicted values, justifying our claims.