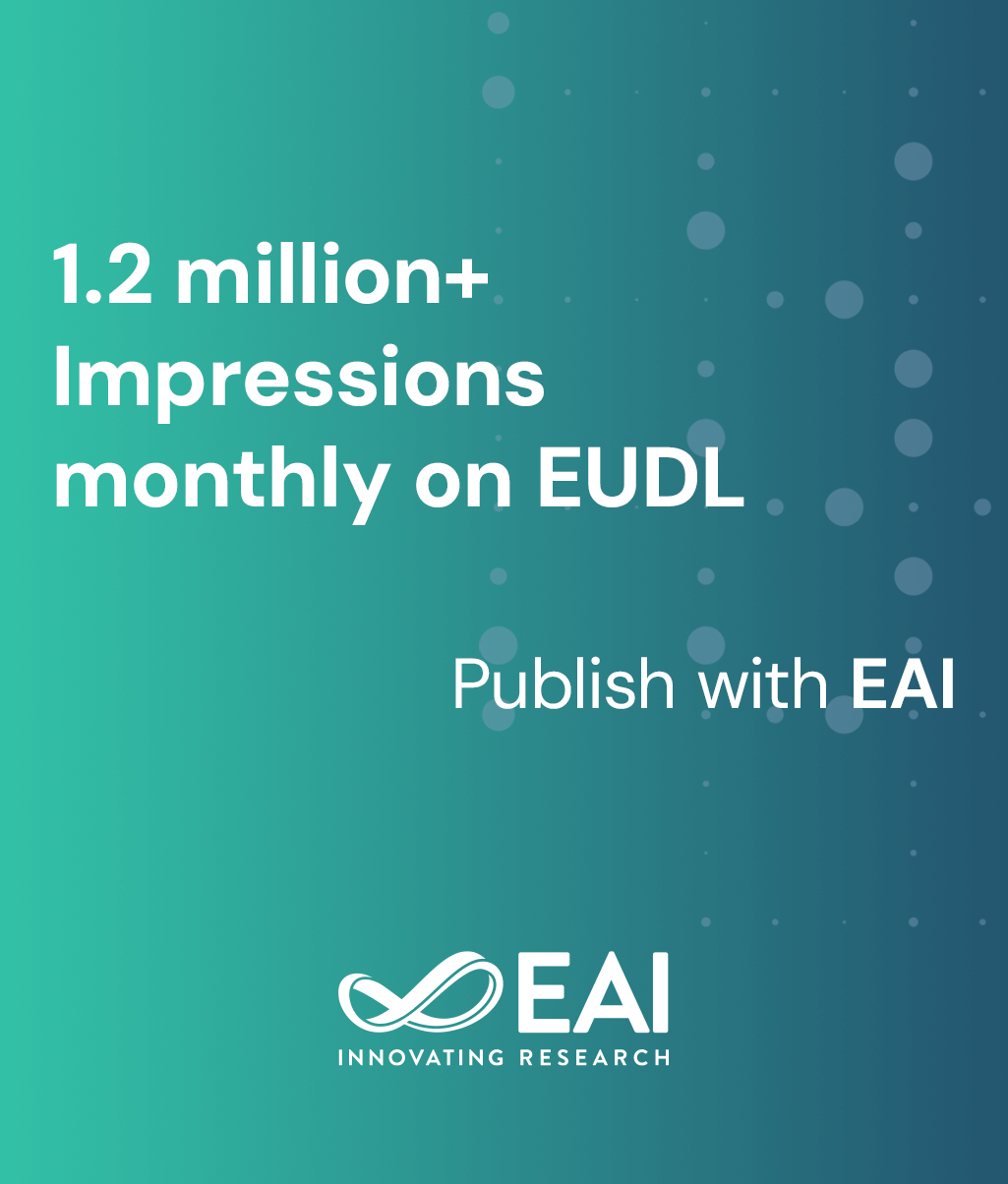
Research Article
Stochastic learning solution for constrained Nash Equilibrium throughput in non saturated wireless collision channels
@INPROCEEDINGS{10.4108/ICST.VALUETOOLS2009.7764, author={Essaid Sabir and Rachid El-Azouzi and Veeraruna Kavitha and Yezekael Hayel and El-Houssine Bouyakhf}, title={Stochastic learning solution for constrained Nash Equilibrium throughput in non saturated wireless collision channels}, proceedings={3rd Workshop on Game Theory in Communication Networks}, publisher={ACM}, proceedings_a={GAMECOMM}, year={2010}, month={5}, keywords={QoS Collision channel Constrained Nash equilibrium Stability Throughput.}, doi={10.4108/ICST.VALUETOOLS2009.7764} }
- Essaid Sabir
Rachid El-Azouzi
Veeraruna Kavitha
Yezekael Hayel
El-Houssine Bouyakhf
Year: 2010
Stochastic learning solution for constrained Nash Equilibrium throughput in non saturated wireless collision channels
GAMECOMM
ICST
DOI: 10.4108/ICST.VALUETOOLS2009.7764
Abstract
We consider finite number of users, with infinite buffer storage, sharing a single channel using the aloha medium access protocol. This is an interesting example of a non saturated collision channel. We investigate the uplink case of a cellular system where each user will select a desired throughput. The users then participate in a non cooperative game wherein they adjust their transmit rate to attain their desired throughput. We show that this game, in contrast to the saturated case, either has no Nash Equilibrium or has infinitely many Nash Equilibria. Further, we show that the region of NE coincides with an appropriate 'stability region'. We also discuss the efficiency of the equilibria in term of energy consumption and congestion rate. Next, we propose two learning algorithms using a stochastic iterative procedure that converges to the best Nash equilibrium. For instance, the first one needs partial information (transmit rates of other users during the last slot) whereas the second is an information less and fully distributed scheme. We approximate the control iterations by an equivalent ordinary differential equation in order to prove that the proposed stochastic learning algorithm converges to a Nash equilibrium even in the absence of any coordination or extra information. Extensive numerical examples and simulations are provided to validate our results.