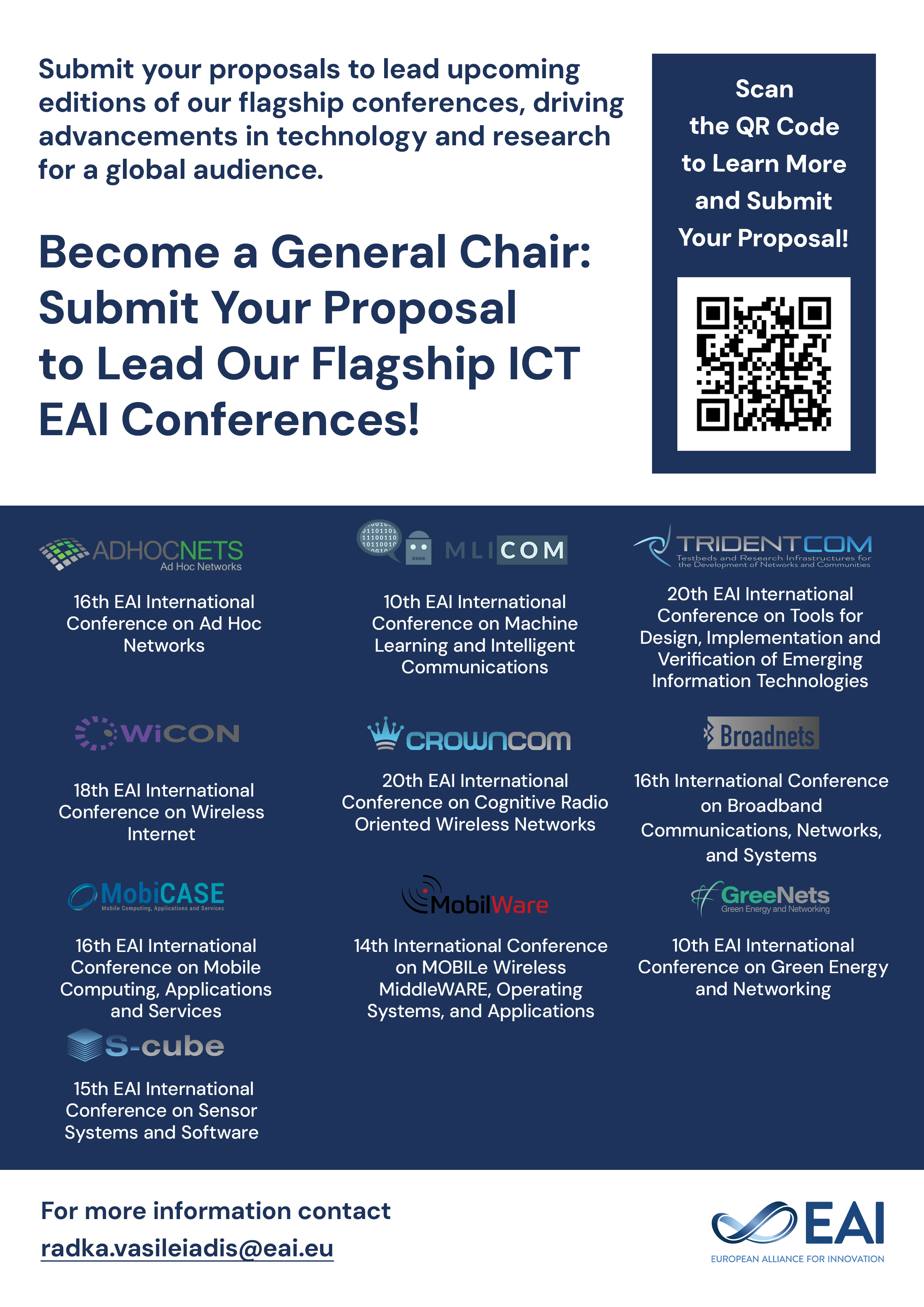
Research Article
On real-time capacity of event-driven data-gathering sensor networks
@INPROCEEDINGS{10.4108/ICST.MOBIQUITOUS2009.6813, author={Bo Jiang and Binoy Ravindran and Hyeonjoong Cho}, title={On real-time capacity of event-driven data-gathering sensor networks}, proceedings={6th Annual International Conference on Mobile and Ubiquitous Systems: Computing, Networking and Services}, publisher={IEEE}, proceedings_a={MOBIQUITOUS}, year={2009}, month={11}, keywords={Capacitive sensors Capacity planning Delay estimation Distributed computing Estimation theory Queueing analysis Telecommunication traffic Throughput Traffic control Wireless sensor networks}, doi={10.4108/ICST.MOBIQUITOUS2009.6813} }
- Bo Jiang
Binoy Ravindran
Hyeonjoong Cho
Year: 2009
On real-time capacity of event-driven data-gathering sensor networks
MOBIQUITOUS
IEEE
DOI: 10.4108/ICST.MOBIQUITOUS2009.6813
Abstract
Network capacity is a critical feature of wireless ad hoc and sensor networks. It is particularly challenging to determine network capacity when combined with other performance objectives such as timeliness. This paper investigates real-time capacity for event-driven data-gathering sensor networks with the unbalanced many-to-one traffic pattern. First, we compute the average allowable throughputs of nodes for a given event distribution, based on which we then leverage results of queuing theory to estimate the per-hop delays. We develop a new slack time distribution scheme for the unbalanced many-to-one traffic pattern, and prove it optimal in terms of the per-hop success probability. Here the per-hop success probability is defined as the probability for a packet to meet its sub-deadlines at each hop. Finally, we define the network-wide real-time capacity, i.e., given a threshold for the per-hop success probability, how much data (in bit per second) can be delivered to the sink node meeting their deadlines. For these research results, we provide some application scenarios, including configuring packet deadlines or verifying a specific deadline configuration, setting a packet's priority for dynamic scheduling, and trading the reliability of real-time data delivery for capacity efficiency etc. We also study two special cases of WSNs, the chain model and the continuous model. Our slack distribution scheme yields consistent or similar results for these two special cases as that of past works, but is more adaptive by supporting more generic cases.