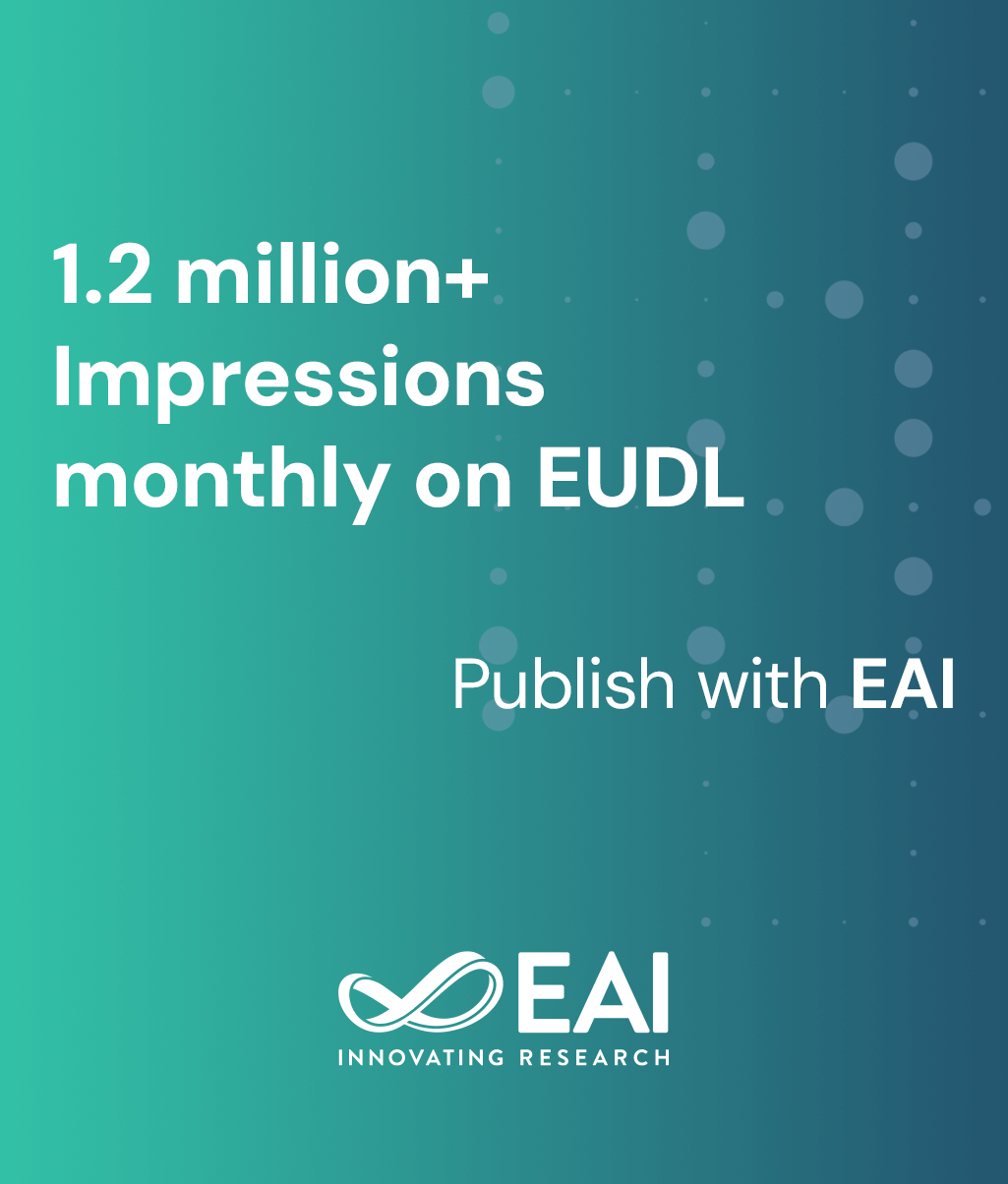
Research Article
Trajectory Enabled Service Support Platform for Mobile Users’ Behavior Pattern Mining
@INPROCEEDINGS{10.4108/ICST.MOBIQUITOUS2009.6768, author={Yanfeng Zhu and Yibo Zhang and Weixiong Shang and Jin Zhou and Chun Ying}, title={Trajectory Enabled Service Support Platform for Mobile Users’ Behavior Pattern Mining}, proceedings={6th Annual International Conference on Mobile and Ubiquitous Systems: Computing, Networking and Services}, publisher={IEEE}, proceedings_a={MOBIQUITOUS}, year={2009}, month={11}, keywords={Communications technology Discrete event simulation Distributed computing Fires Medical services Microelectronics Monitoring Shape Wireless communication Wireless sensor networks}, doi={10.4108/ICST.MOBIQUITOUS2009.6768} }
- Yanfeng Zhu
Yibo Zhang
Weixiong Shang
Jin Zhou
Chun Ying
Year: 2009
Trajectory Enabled Service Support Platform for Mobile Users’ Behavior Pattern Mining
MOBIQUITOUS
IEEE
DOI: 10.4108/ICST.MOBIQUITOUS2009.6768
Abstract
Existing operational support systems of GSM service providers focus on collecting and maintaining massive rough Cell- ID based location data, which cannot satisfy the requirement of new trajectory based services in identifying the behaviors of mobile users. In this paper, we introduce a trajectory enabled service support platform to convert the location data into limited meaningful mobile user’s behavior patterns, which benefit the trajectory based services by simplifying the behavior detection. The core technologies of the platform are the pattern selection, which requires to cover the information included in the raw location data as more as possible, and the run-time mining algorithm, which requires less storage space. We propose a new concept, transient entropy, to identify the moving speed of users, and based on which we define and mine four types of behavior patterns: frequent locations, frequent trajectory, meaningful location, and moving mode. By analyzing the sojourn distribution, we find that the sojourn time in each location follows a Zipf distribution, based on which we present a runtime algorithm to mine the behavior patterns with less storage space. A realistic experiments is given to validate the proposed platform and algorithms.