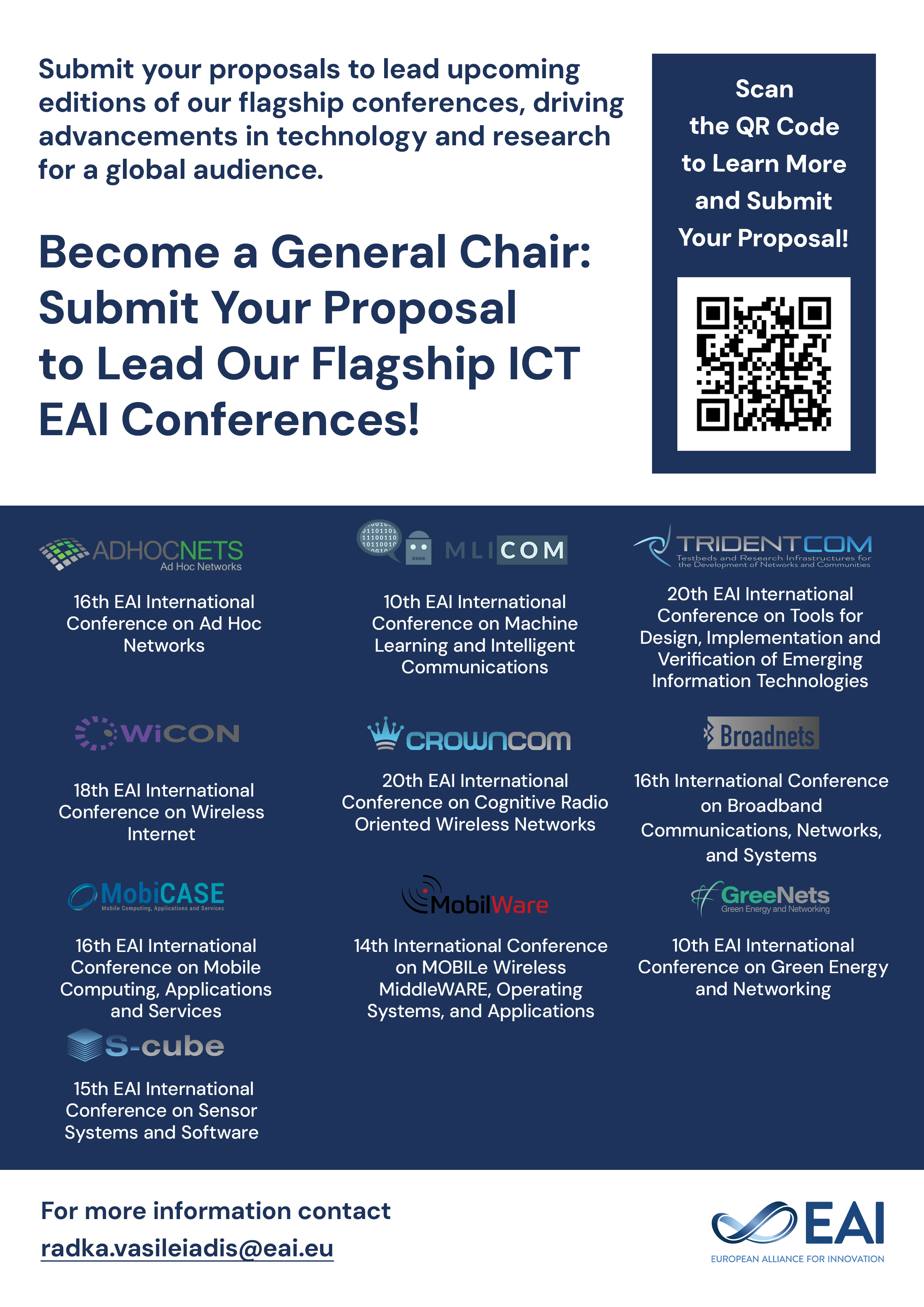
Research Article
Classifying Wheelchair Propulsion Patterns with a Wrist Mounted Accelerometer
@INPROCEEDINGS{10.4108/ICST.BODYNETS2008.2963, author={Brian French and Asim Smailagic and Dan Siewiorek and Vishnu Ambur and Divya Tyamagundlu}, title={Classifying Wheelchair Propulsion Patterns with a Wrist Mounted Accelerometer}, proceedings={3rd International ICST Conference on Body Area Networks}, publisher={ICST}, proceedings_a={BODYNETS}, year={2010}, month={5}, keywords={eWatch wearable sensors machine learning manual wheelchair propulsion patterns}, doi={10.4108/ICST.BODYNETS2008.2963} }
- Brian French
Asim Smailagic
Dan Siewiorek
Vishnu Ambur
Divya Tyamagundlu
Year: 2010
Classifying Wheelchair Propulsion Patterns with a Wrist Mounted Accelerometer
BODYNETS
ICST
DOI: 10.4108/ICST.BODYNETS2008.2963
Abstract
In this paper, we describe a manual wheelchair propulsion classification system which recognizes different patterns using a wrist mounted accelerometer. Four distinct propulsion patterns have been identified in a limited user study. This study is the first attempt at classifying wheelchair propulsion patterns using low-fidelity, body-worn sensors. Data was collected using all four propulsion patterns on a variety of surface types. The results of two machine learning algorithms are compared. Accuracies of over 90% were achievable even with a simple classifier such as k-Nearest Neighbor (kNN). Being able to identify current propulsion patterns and provide real-time feedback to novice and expert wheelchair users is potentially useful in preventing future repetitive use injuries.