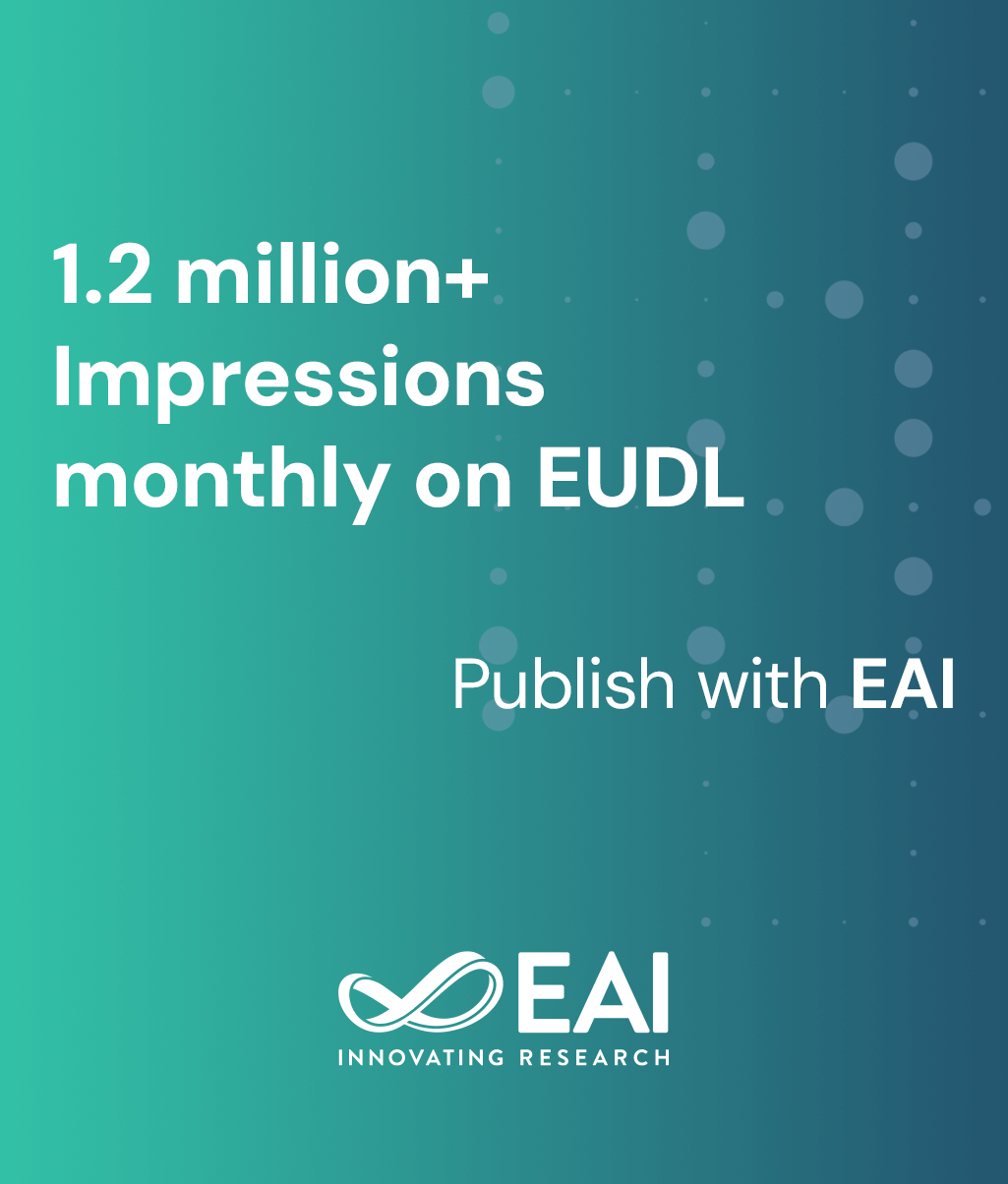
Research Article
SMASH: A Distributed Sensing and Processing Garment for the Classification of Upper Body Postures
@INPROCEEDINGS{10.4108/ICST.BODYNETS2008.2955, author={Holger Harm and Oliver Amft and Daniel Roggen and Gerhard Tr\o{}ster}, title={SMASH: A Distributed Sensing and Processing Garment for the Classification of Upper Body Postures}, proceedings={3rd International ICST Conference on Body Area Networks}, publisher={ICST}, proceedings_a={BODYNETS}, year={2010}, month={5}, keywords={Smart Shirt SMASH Smart Textiles Posture Classification}, doi={10.4108/ICST.BODYNETS2008.2955} }
- Holger Harm
Oliver Amft
Daniel Roggen
Gerhard Tröster
Year: 2010
SMASH: A Distributed Sensing and Processing Garment for the Classification of Upper Body Postures
BODYNETS
ICST
DOI: 10.4108/ICST.BODYNETS2008.2955
Abstract
This paper introduces a smart textile for posture classification. A distributed sensing and processing architecture is implemented into a loose fitting long sleeve shirt. Standardized interfaces to remote periphery support the variable placement of different sensor modalities at any location of the textile. The shirt is equipped with acceleration sensors in order to determine the postural resolution and the systems feasibility for applications in movement rehabilitation. For the garment characterization an arm posture measurement method is proposed and applied in a study with 5 users. The classification performance is analyzed on data from overall 8 users, conducting 12 posture types, relevant for shoulder and elbow joint rehabilitation. We present results for different user-modes, with classification rates of 89% for a user-independent evaluation. Moreover, the relation of body dimensions on the posture classification performance are analyzed.