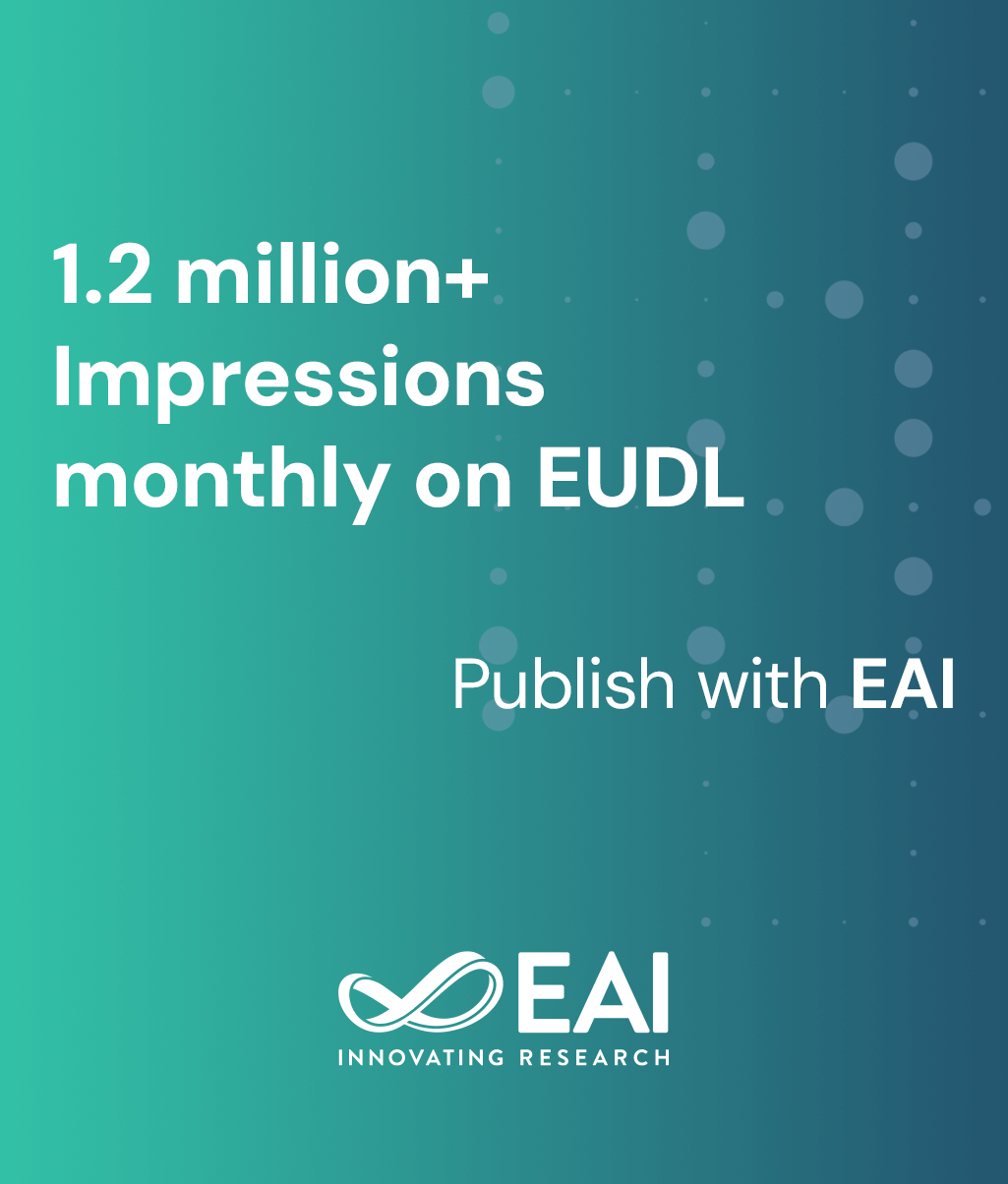
Research Article
Automated Speech-based Screening for Alzheimer’s Disease in a Care Service Scenario
@INPROCEEDINGS{10.1145/3154862.3154915, author={Johannes Tr\o{}ger and Nicklas Linz and Jan Alexandersson and Alexandra K\o{}nig and Philippe Robert}, title={Automated Speech-based Screening for Alzheimer’s Disease in a Care Service Scenario}, proceedings={11th EAI International Conference on Pervasive Computing Technologies for Healthcare}, publisher={ACM}, proceedings_a={PERVASIVEHEALTH}, year={2018}, month={1}, keywords={dementia screening vocal features classification speech analysis clinical phonetics}, doi={10.1145/3154862.3154915} }
- Johannes Tröger
Nicklas Linz
Jan Alexandersson
Alexandra König
Philippe Robert
Year: 2018
Automated Speech-based Screening for Alzheimer’s Disease in a Care Service Scenario
PERVASIVEHEALTH
ACM
DOI: 10.1145/3154862.3154915
Abstract
This paper describes a benchmark study for a lightweight and low-cost dementia screening tool. The tool is easy to administer, requires no additional experimentation material, and automatically evaluates and indicates potential subjects with dementia. The protocol foresees that subjects answer four distinct tasks, three of which are ordinary questions and one is a counting prompt. In our care use case, older people are assessed remotely via the tool, potentially even via tele- phone or within a daily care service routine. The assessment results are subsequently sent to professionals who initiate fur- ther steps. A machine learning classifier was trained on the French Dem@Care corpus. Solely utilizing vocal features, the classifier reaches 89% accuracy. Implications for the use case and further steps are discussed.