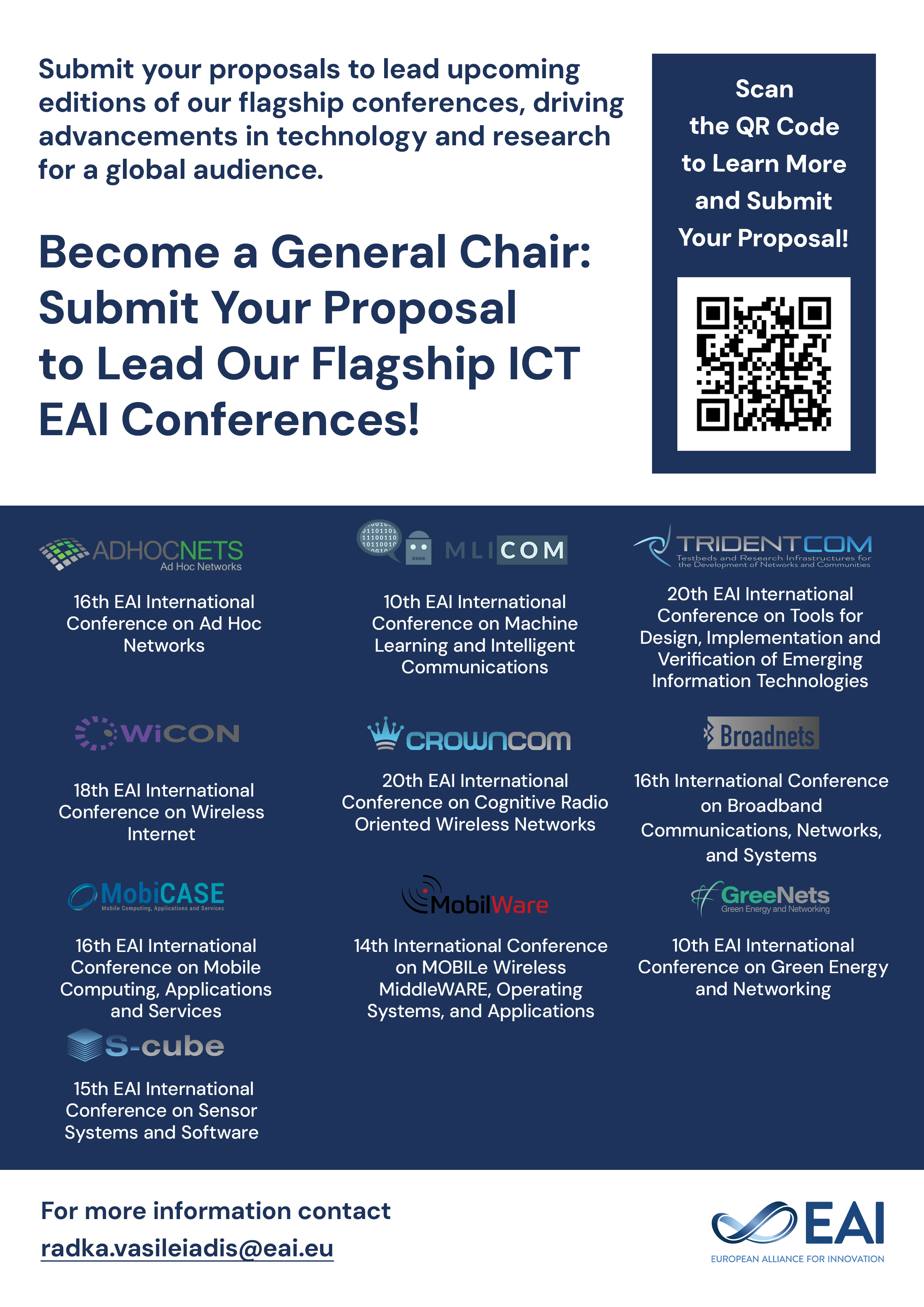
Research Article
KinRes: Depth Sensor Noise Reduction in Contactless Respiratory Monitoring
@INPROCEEDINGS{10.1145/3154862.3154896, author={Kaveh Bakhtiyari and J\'{y}rgen Ziegler and Hafizah Husain}, title={KinRes: Depth Sensor Noise Reduction in Contactless Respiratory Monitoring}, proceedings={11th EAI International Conference on Pervasive Computing Technologies for Healthcare}, publisher={ACM}, proceedings_a={PERVASIVEHEALTH}, year={2018}, month={1}, keywords={microsoft kinect signal processing greedy algorithm}, doi={10.1145/3154862.3154896} }
- Kaveh Bakhtiyari
Jürgen Ziegler
Hafizah Husain
Year: 2018
KinRes: Depth Sensor Noise Reduction in Contactless Respiratory Monitoring
PERVASIVEHEALTH
ACM
DOI: 10.1145/3154862.3154896
Abstract
This paper proposes a novel reliable solution, named KinRes, to extract contactless respiratory signal via an IR-3D Depth sensor (Microsoft Kinect 2) on human subjects interacting with a computer. The depth sensor is very sensitive to the minor changes so that the body movements impose noise in the depth values. Previous studies on contactless respiratory concentrated solely on the still laid subjects on a surface to minimize the possible artifacts. To overcome these limitations, we low-pass filter the extracted signal. Then, a greedy self-correction algorithm is developed to correct the false detected peaks & troughs. The processed signal is validated with a simultaneous signal from a respiratory belt. This framework improved the accuracy of the signal by 24% for the subjects in a normal sitting position.