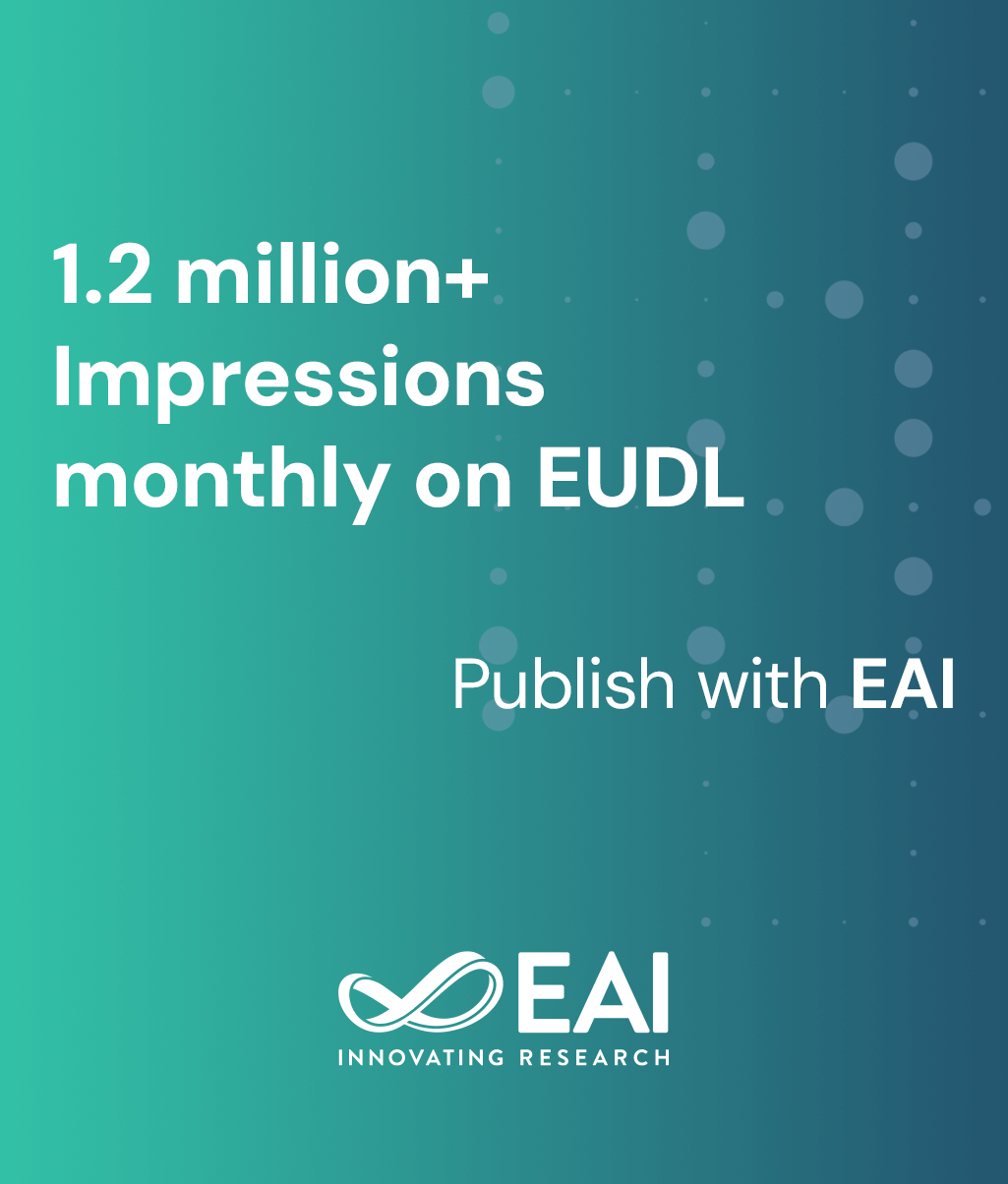
Research Article
Exploring Stroke-Associated Hemiparesis Assessment with Support Vector Machines
@INPROCEEDINGS{10.1145/3154862.3154894, author={Vishwajith Ramesh and Kunal Agrawal and Brett Meyer and Gert Cauwenberghs and Nadir Weibel}, title={Exploring Stroke-Associated Hemiparesis Assessment with Support Vector Machines}, proceedings={11th EAI International Conference on Pervasive Computing Technologies for Healthcare}, publisher={ACM}, proceedings_a={PERVASIVEHEALTH}, year={2018}, month={1}, keywords={stroke hemiparesis machine learning support vector machines kinect body-tracking posture}, doi={10.1145/3154862.3154894} }
- Vishwajith Ramesh
Kunal Agrawal
Brett Meyer
Gert Cauwenberghs
Nadir Weibel
Year: 2018
Exploring Stroke-Associated Hemiparesis Assessment with Support Vector Machines
PERVASIVEHEALTH
ACM
DOI: 10.1145/3154862.3154894
Abstract
Hemiparesis, the weakness of one side of the body, affects the ability of stroke survivors to move and walk. With prevalence in 80% of survivors, hemiparesis is an important measure for stroke severity. It is generally diagnosed through motor tests performed as part of the National Institute of Health Stroke Scale (NIHSS). Here we report on initial work for an alternate way of identifying hemiparesis that leverages body joint position data captured by the Microsoft Kinect v2 of people resting while waiting for the neurological examination. We employ support vector machines with 10 stroke patients and 9 healthy controls to characterize hemiparesis based on the lower core body angles of the participants, and compare our results to neurologists’ diagnoses. We were able to identify left-side hemiparesis, right-side hemiparesis, or no hemiparesis with > 89% accuracy when looking at the lower body angles and observing the patients for 1 minute.