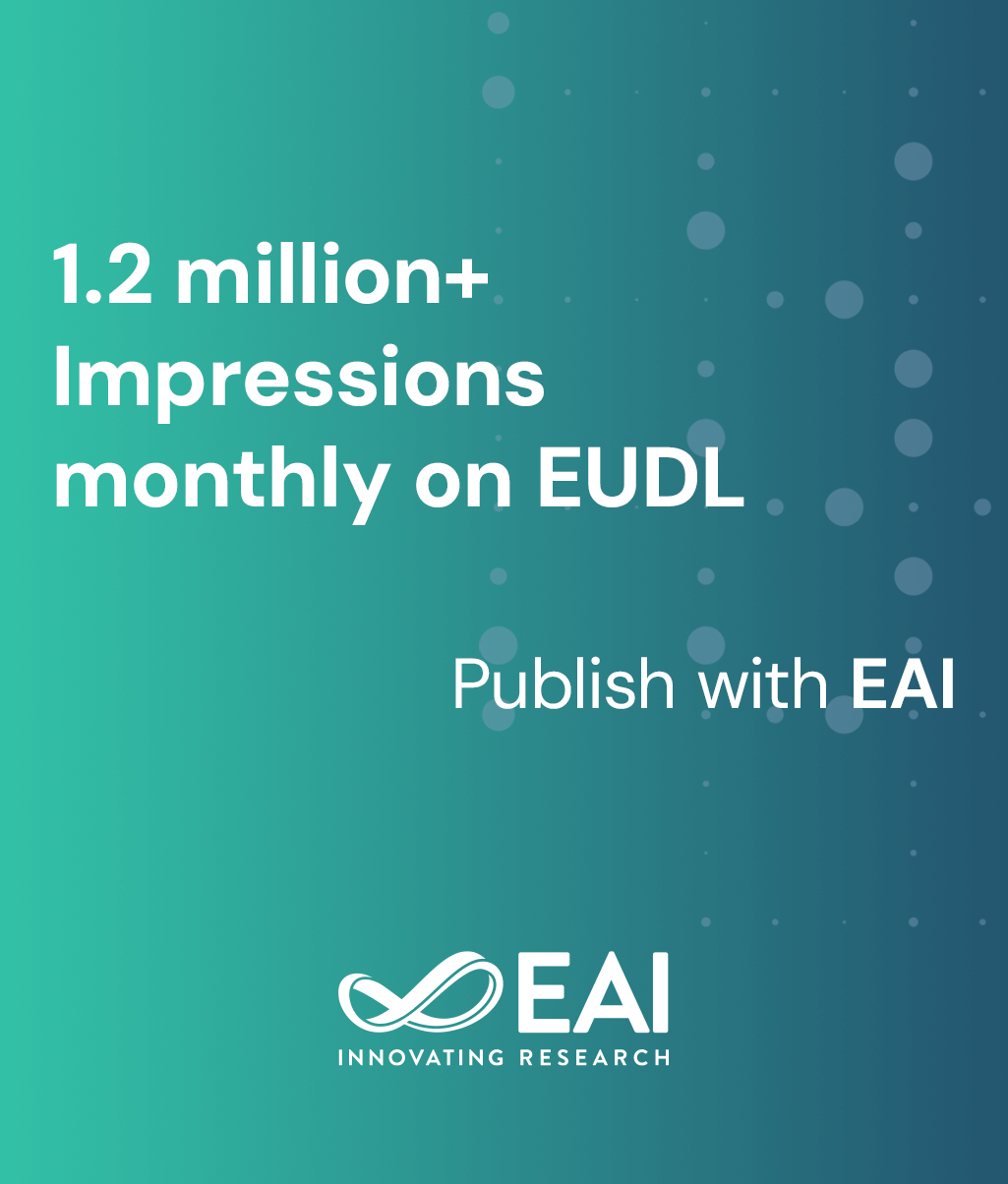
Research Article
A Fusion Approach for Non-invasive Detection of Coronary Artery Disease
@INPROCEEDINGS{10.1145/3154862.3154871, author={Anirban Dutta Choudhury and Rohan Banerjee and Arpan Pal and K M Mandana}, title={A Fusion Approach for Non-invasive Detection of Coronary Artery Disease}, proceedings={11th EAI International Conference on Pervasive Computing Technologies for Healthcare}, publisher={ACM}, proceedings_a={PERVASIVEHEALTH}, year={2018}, month={1}, keywords={preventive healthcare coronary artery disease non-invasive classification}, doi={10.1145/3154862.3154871} }
- Anirban Dutta Choudhury
Rohan Banerjee
Arpan Pal
K M Mandana
Year: 2018
A Fusion Approach for Non-invasive Detection of Coronary Artery Disease
PERVASIVEHEALTH
ACM
DOI: 10.1145/3154862.3154871
Abstract
Coronary Artery Disease (CAD) kills millions of people every year across the world. In this paper, we present a novel idea of a low cost, non-invasive screening system for early detection of CAD patients by fusion of phonocardiogram (PCG) and photoplethysmogram (PPG) signals. Two sets of time and frequency features are extracted from both the signals. Support Vector Machine (SVM) is used to classify each subject separately based on both the feature sets. Finally, the outcomes of the two classifiers are fused at the decision level, depending upon the maximum absolute distance of the test data-points form their respective SVM hyperplane. We created a corpus of 25 subjects, containing 10 CAD and 15 non CAD subjects using low cost non-medical grade devices. Results show that either of PCG or PPG based classifiers yields sensitivity and specificity scores close to 0.6 and 0.8 respectively in identifying CAD. Whereas, a significant improvement in both sensitivity (0.8) as well as specificity (0.93) can be simultaneously achieved by incorporating the proposed fusion approach.