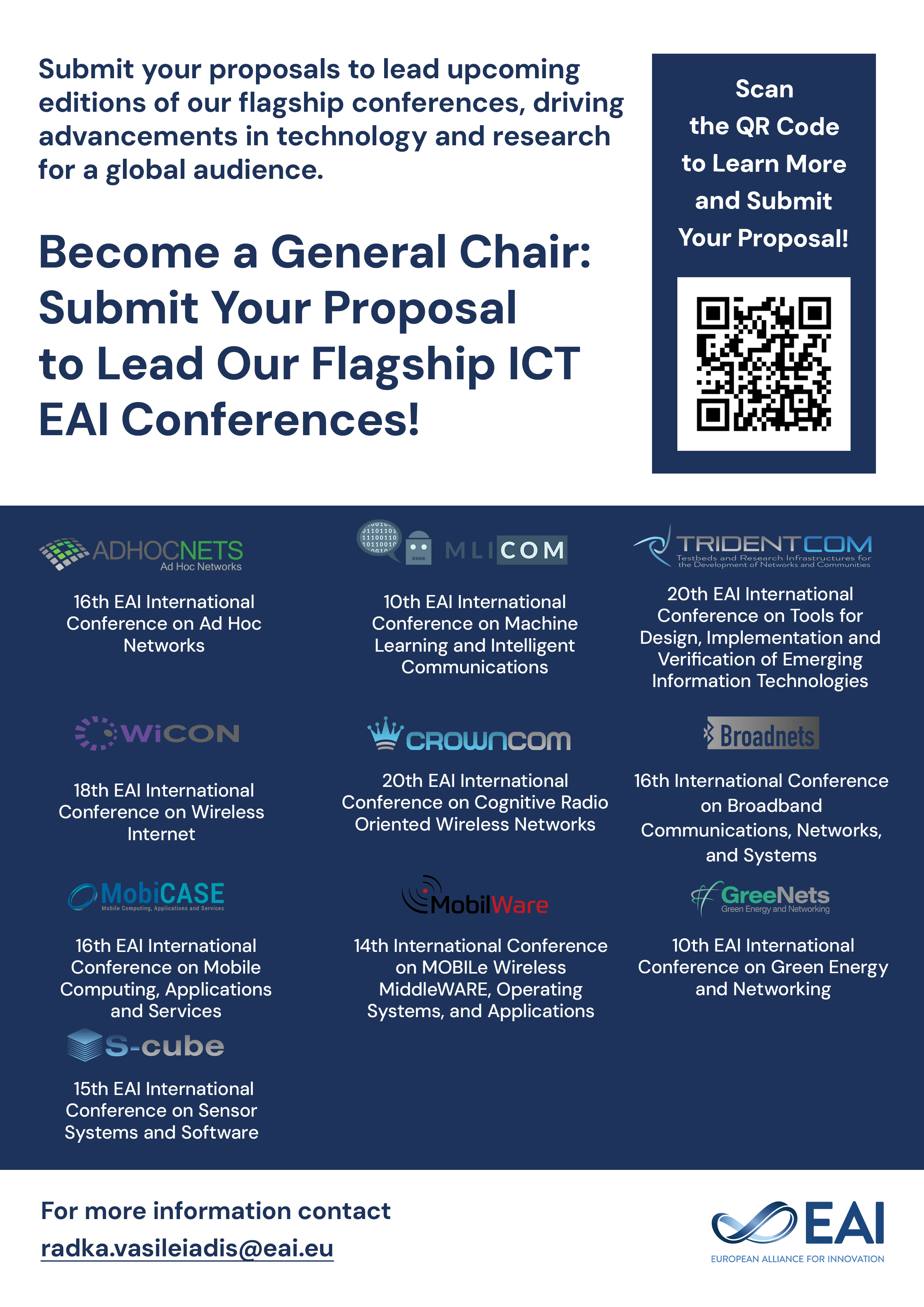
Research Article
DarSens: A Framework for Distributed Activity Recognition from Body-Worn Sensors
@INPROCEEDINGS{10.1145/2221924.2221969, author={Michael Haslgruebler and Clemens Holzmann}, title={DarSens: A Framework for Distributed Activity Recognition from Body-Worn Sensors}, proceedings={5th International ICST Conference on Body Area Networks}, publisher={ACM}, proceedings_a={BODYNETS}, year={2012}, month={6}, keywords={Body sensor network context-awareness activity recognition self-organization}, doi={10.1145/2221924.2221969} }
- Michael Haslgruebler
Clemens Holzmann
Year: 2012
DarSens: A Framework for Distributed Activity Recognition from Body-Worn Sensors
BODYNETS
ACM
DOI: 10.1145/2221924.2221969
Abstract
With the increasing amount of sensors in our environment, the desire to reuse existing sensors for dierent applications grows. However, most appliances do not provide access to their sensors in a cross-application manner, but rather use them for a specic purpose only. In this paper, we describe a framework which provides a way to access not only the data, but also the processing capabilities of a sensor system in a reusable way, without the need for a-priori knowledge about the availability of sensors in the environment. In particular, the presented framework is able to run on an embedded system platform, and is used for the recognition of human activities in a body sensor network. Notably, both the feature extraction and classication are performed within the network. Hence, we can use the processing power of sensor nodes, and do not have to revert to the processing capabilities of a client device which is using the sensor network. Two experiments have been conducted to show the feasibility and performance of our approach in typical activity recognition scenarios.