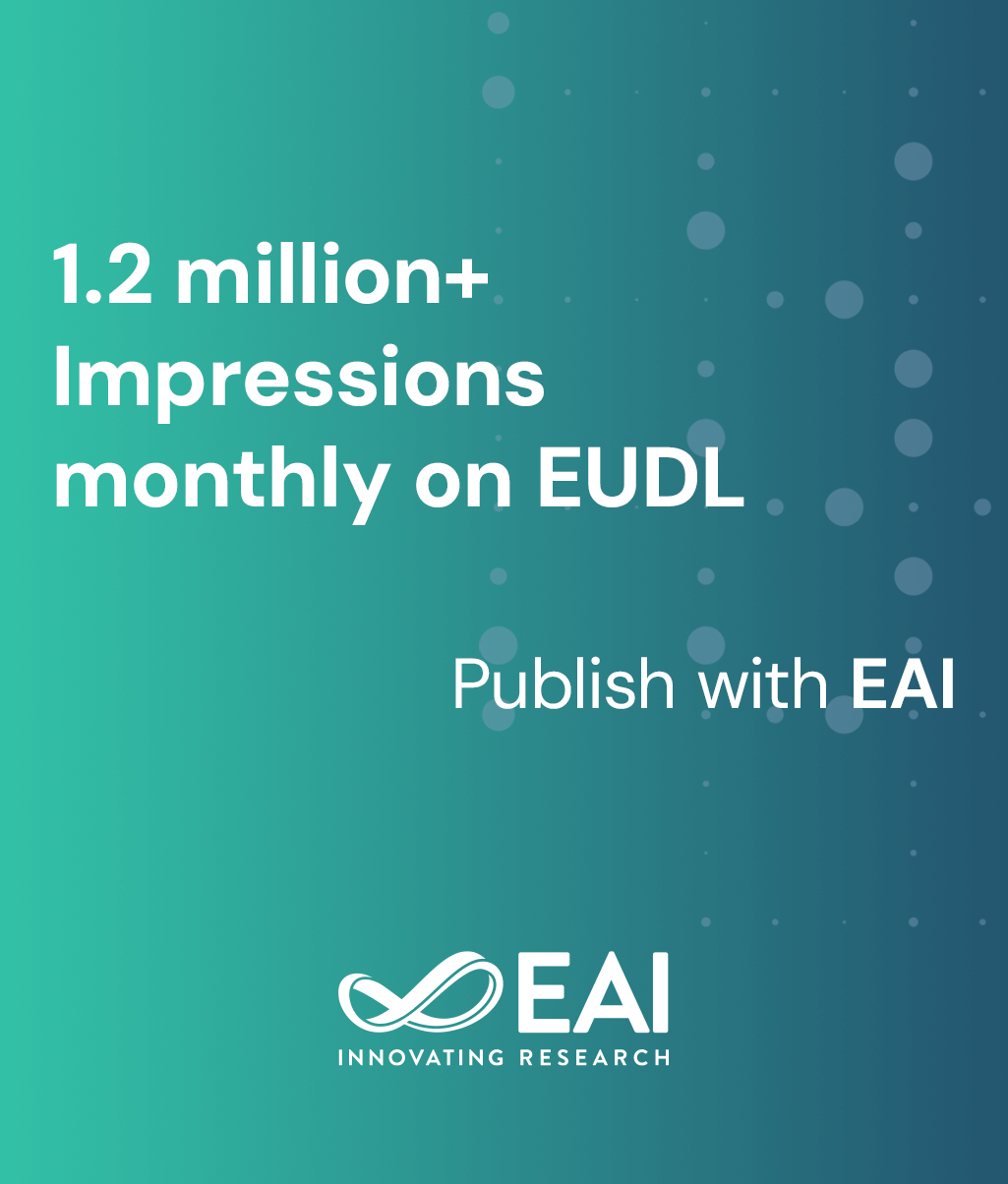
Research Article
Exponential bounds and stopping rules for MCMC and general Markov chains
@INPROCEEDINGS{10.1145/1190095.1190152, author={I. Kontoyiannis and L.A. Lastras-Montano and S.P. Meyn}, title={Exponential bounds and stopping rules for MCMC and general Markov chains}, proceedings={1st International ICST Conference on Performance Evaluation Methodologies and Tools}, publisher={ACM}, proceedings_a={VALUETOOLS}, year={2012}, month={4}, keywords={}, doi={10.1145/1190095.1190152} }
- I. Kontoyiannis
L.A. Lastras-Montano
S.P. Meyn
Year: 2012
Exponential bounds and stopping rules for MCMC and general Markov chains
VALUETOOLS
ACM
DOI: 10.1145/1190095.1190152
Abstract
We develop explicit, general bounds for the probability that the empirical sample averages of a function of a Markov chain on a general alphabet will exceed the steady-state mean of that function by a given amount. Our bounds combine simple information-theoretic ideas together with techniques from optimization and some fairly elementary tools from analysis. In one direction, motivated by central problems in simulation, we develop bounds for the general class of "geometrically ergodic" Markov chains. These bounds take a form that is particularly suited to simulation problems, and they naturally lead to a new class of sampling criteria. These are illustrated by several examples. In another direction, we obtain a new bound for the important special class of Doeblin chains; this bound is optimal, in the sense that in the special case of independent and identically distributed random variables it essentially reduces to the classical Hoeffding bound.