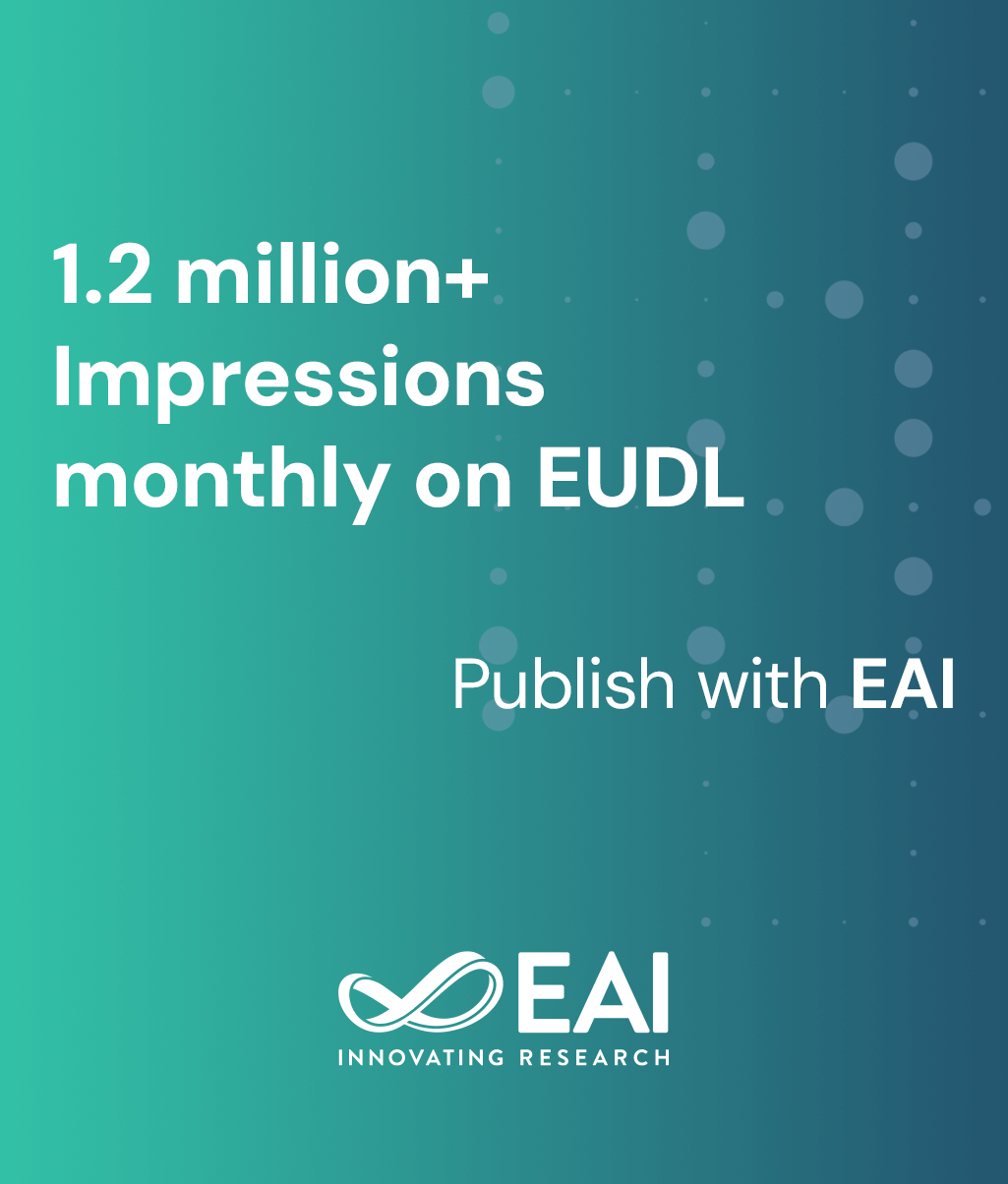
Research Article
Anomaly-Based Behavior Analysis of Wireless Network Security
@INPROCEEDINGS{10.1109/MOBIQ.2007.4451054, author={Samer Fayssal and Salim Hariri and Youssif Al-Nashif}, title={Anomaly-Based Behavior Analysis of Wireless Network Security}, proceedings={2nd International ICST Workshop on Personalized Networks}, publisher={IEEE}, proceedings_a={PERNETS}, year={2008}, month={2}, keywords={Communication system security Computer network management Computer networks Computer security Condition monitoring Intrusion detection Protection Signal analysis Wireless LAN Wireless networks}, doi={10.1109/MOBIQ.2007.4451054} }
- Samer Fayssal
Salim Hariri
Youssif Al-Nashif
Year: 2008
Anomaly-Based Behavior Analysis of Wireless Network Security
PERNETS
IEEE
DOI: 10.1109/MOBIQ.2007.4451054
Abstract
The exponential growth in wireless network faults, vulnerabilities, and attacks make the wireless local area network (WLAN) security management a challenging research area. Newer network cards implemented more security measures according to the IEEE recommendations [14]; but the wireless network is still vulnerable to denial of service attacks or to other traditional attacks due to existing wide deployment of network cards with well-known security vulnerabilities. The effectiveness of a wireless intrusion detection system (WIDS) relies on updating its security rules; many current WIDSs use static security rule settings based on expert knowledge. However, updating those security rules can be time-consuming and expensive. In this paper, we present a novel approach based on multi-channel monitoring and anomaly analysis of station localization, packet analysis, and state tracking to detect wireless attacks; we use adaptive machine learning and genetic search to dynamically set optimal anomaly thresholds and select the proper set of features necessary to efficiently detect network attacks. We present a self-protection system that has the following salient features: monitor the wireless network, generate network features, track wireless network state machine violations, generate wireless flow keys (WFK), and use the dynamically updated anomaly and misuse rules to detect complex known and unknown wireless attacks. To quantify the attack impact, we use the abnormality distance from the trained norm and multivariate analysis to correlate multiple selected features contributing to the final decision. We validate our wireless self protection system (WSPS) approach by experimenting with more than 20 different types of wireless attacks. Our experimental results show that the WSPS approach can protect from wireless network attacks with a false positive rate of 0.1209% and more than 99% detection rate.