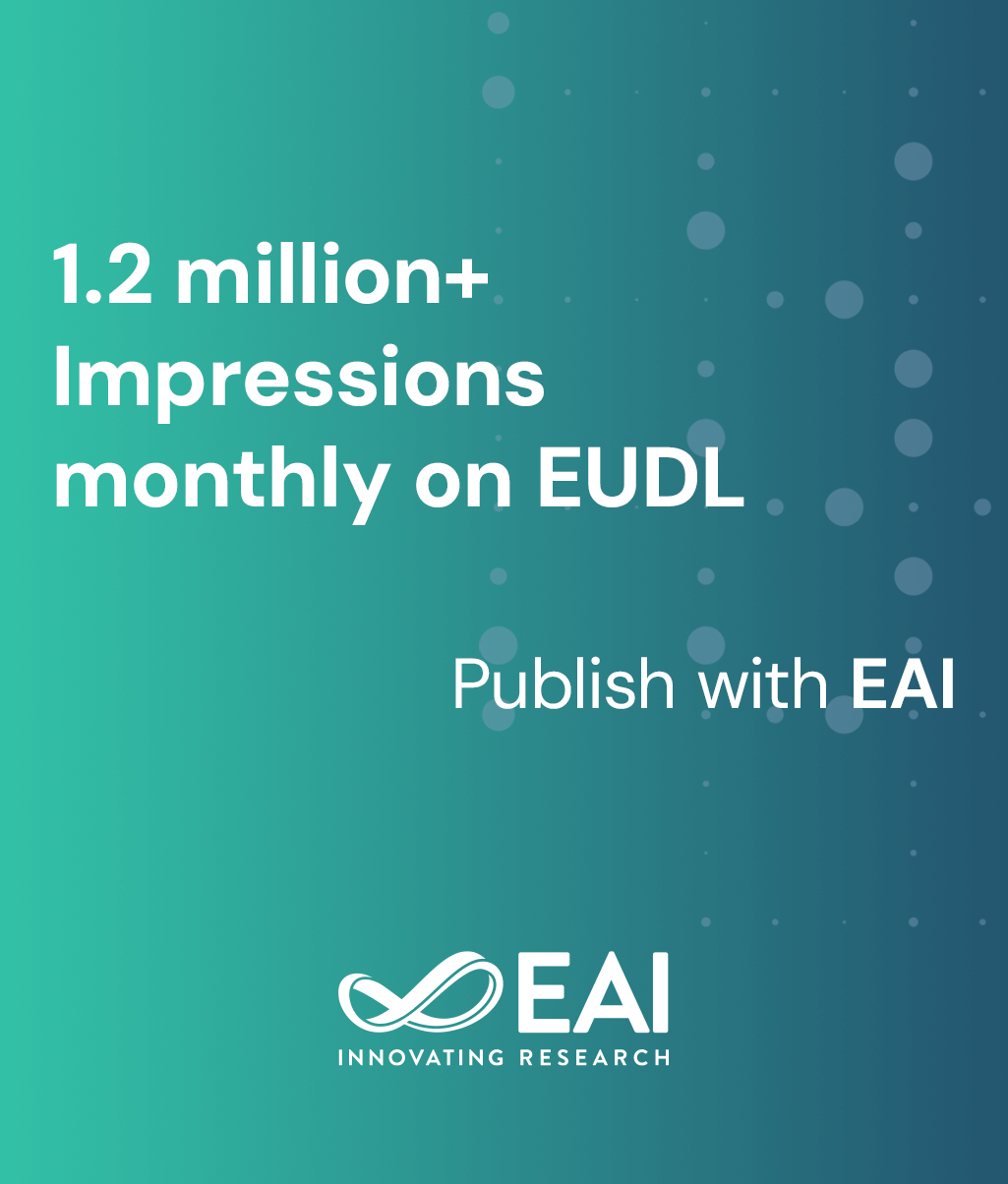
Research Article
Price-Based Spectrum Management in Cognitive Radio Networks
@INPROCEEDINGS{10.1109/CROWNCOM.2007.4549775, author={Fan Wang and Marwan Krunz and Shuguang Cui}, title={Price-Based Spectrum Management in Cognitive Radio Networks}, proceedings={2nd International ICST Conference on Cognitive Radio Oriented Wireless Networks and Communications}, publisher={IEEE}, proceedings_a={CROWNCOM}, year={2008}, month={6}, keywords={Ad hoc networks Chromium Cognitive radio Interference channels Iterative algorithms Nash equilibrium Pricing Radio spectrum management Sliding mode control System performance}, doi={10.1109/CROWNCOM.2007.4549775} }
- Fan Wang
Marwan Krunz
Shuguang Cui
Year: 2008
Price-Based Spectrum Management in Cognitive Radio Networks
CROWNCOM
IEEE
DOI: 10.1109/CROWNCOM.2007.4549775
Abstract
A key challenge in operating cognitive radios (CRs) in a self-organizing (ad hoc) network is how to adaptively and efficiently allocate transmission powers and spectrum among CRs according to the surrounding environment. Most previous works address this issue via heuristic approaches or using centralized solutions. In this paper, we present a novel joint power/channel allocation scheme that uses a distributed pricing strategy to improve the network’s performance. In this scheme, the spectrum allocation problem is modelled as a non-cooperative game. A price-based iterative water-filling (PIWF) algorithm is proposed, which allows users to converge to the Nash Equilibrium (NE). This PIWF algorithm can be implemented distributively with CRs repeatedly negotiating their best transmission powers and spectrum. Simulation results show that the social optimality of the NE solution is dramatically improved with our price-based strategy. Based on the orders by which CRs take actions, we study sequential and parallel versions of the algorithm. We show that the parallel version converges faster than the sequential version.