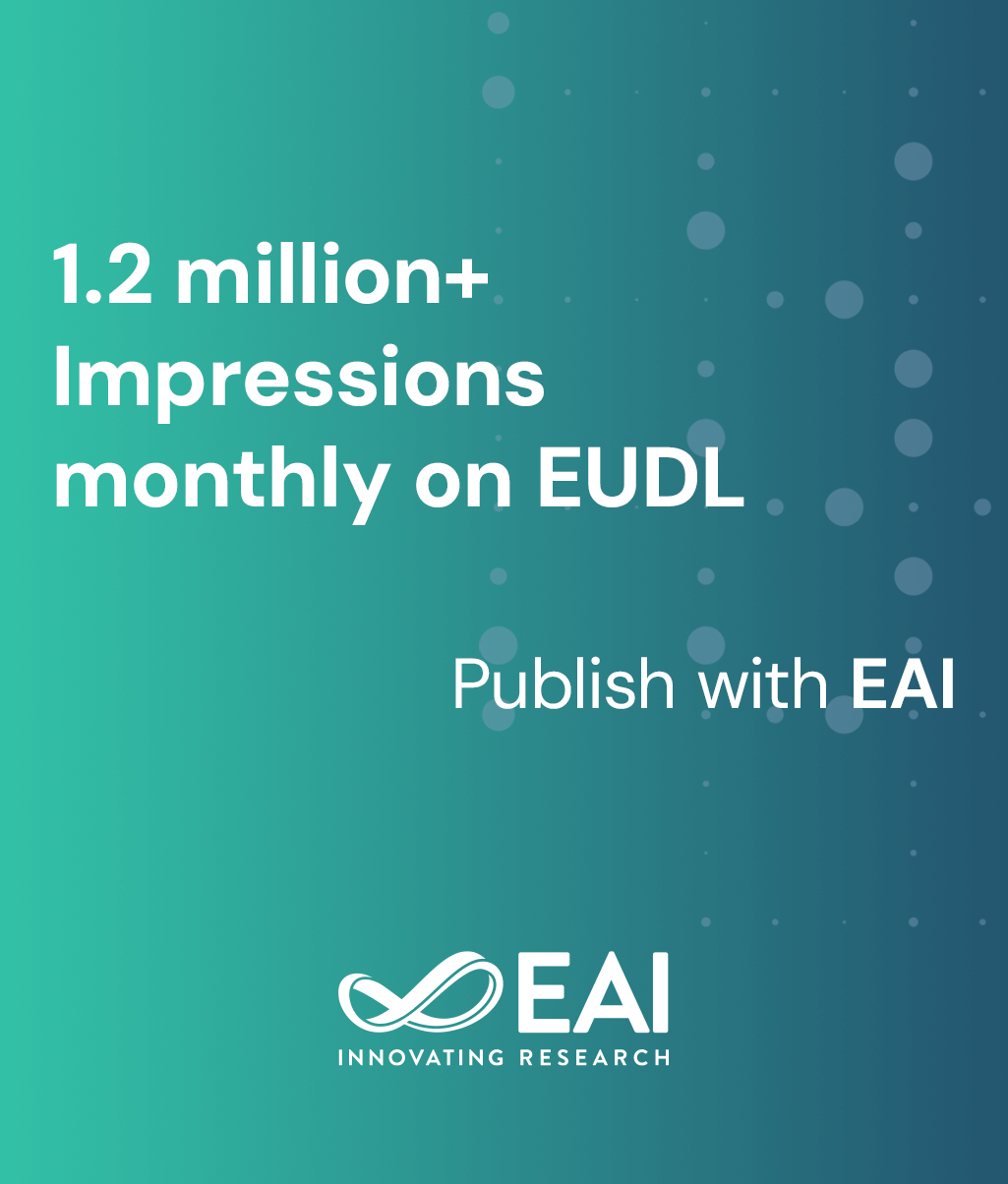
Research Article
Modeling Trust in Collaborative Information Systems
@INPROCEEDINGS{10.1109/COLCOM.2007.4553846, author={Sara Javanmardi and Cristina Videira Lopes}, title={Modeling Trust in Collaborative Information Systems}, proceedings={3rd International ICST Conference on Collaborative Computing: Networking, Applications and Worksharin}, publisher={IEEE}, proceedings_a={COLLABORATECOM}, year={2008}, month={6}, keywords={Collaboration Collaborative software Collaborative tools Data mining Hidden Markov models Information resources Information security Information systems Protection Wikipedia}, doi={10.1109/COLCOM.2007.4553846} }
- Sara Javanmardi
Cristina Videira Lopes
Year: 2008
Modeling Trust in Collaborative Information Systems
COLLABORATECOM
IEEE
DOI: 10.1109/COLCOM.2007.4553846
Abstract
Collaborative systems available on the Web allow millions of users to share information through a growing collection of tools and platforms such as wikis, blogs and shared forums. All of these systems contain information and resources with different degrees of sensitivity. However, the open nature of such infrastructures makes it difficult for users to determine the reliability of the available information and trustworthiness of information providers. Hence, integrating trust management systems to open collaborative systems can play a crucial role in the growth and popularity of open information repositories. In this paper, we present a trust model for collaborative systems, namely for platforms based on wiki technology. This model, based on Hidden Markov Models, estimates the reputation of the contributors and the reliability of the content dynamically. The focus of this paper is on reputation estimation. Evaluation results based on a subset of Wikipedia shows that the model can effectively be used for identifying vandals, and users with high quality contributions.