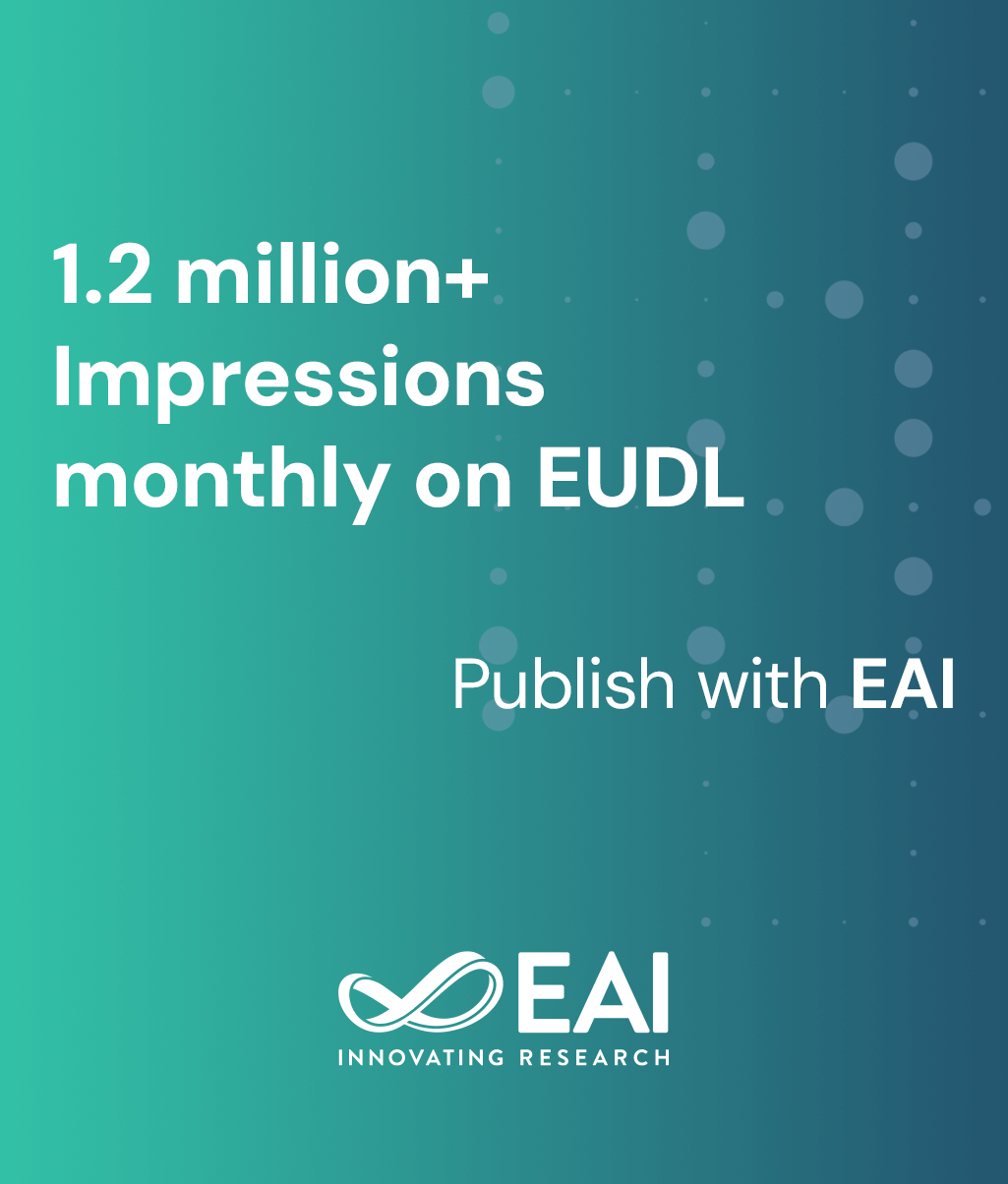
Research Article
Developing a framework for integrating prior problem solving and knowledge sharing histories of a group to predict future group performance
@INPROCEEDINGS{10.1109/COLCOM.2005.1651209, author={Ron Stevens and Amy Soller and Alessandra Giordani and Luca Gerosa and Melanie Cooper and Charlie Cox}, title={Developing a framework for integrating prior problem solving and knowledge sharing histories of a group to predict future group performance}, proceedings={1st International Conference on Collaborative Computing: Networking, Applications and Worksharing}, publisher={IEEE}, proceedings_a={COLLABORATECOM}, year={2006}, month={7}, keywords={Artificial neural networks Chemistry Cities and towns Collaborative work History Machine learning Online Communities/Technical Collaboration Predictive models Problem-solving Telecommunications}, doi={10.1109/COLCOM.2005.1651209} }
- Ron Stevens
Amy Soller
Alessandra Giordani
Luca Gerosa
Melanie Cooper
Charlie Cox
Year: 2006
Developing a framework for integrating prior problem solving and knowledge sharing histories of a group to predict future group performance
COLLABORATECOM
IEEE
DOI: 10.1109/COLCOM.2005.1651209
Abstract
Using a combination of machine learning probabilistic tools, we have shown that some chemistry students fail to develop productive problem solving strategies through practice alone and will require interventions to continue making strategic progress. One particularly useful form of intervention was face-to-face collaborative learning which increased the overall solution rate of the problem solving while also improving the strategies used. However, the collaborative intervention was not effective for all groups making complicated. To better model the effects of group composition we have developed a synchronous and symmetrical collaborative extension to the online IMMEX problem solving environment. This online collaborative environment appeared an accurate representation of the face-to-face collaboration episode in that both groupings showed similar gains in the problem solution frequency as well as in the differential use of particular strategies. We also noticed that some groups, like some individuals, rapidly developed and persisted with unproductive approaches highlighting the importance of identifying, and perhaps re-assembling such groups for subsequent problem solving. To support such decisions, we describe a causal model approach for integrating the performance and knowledge sharing histories of a group to help predict which groups should remain together.