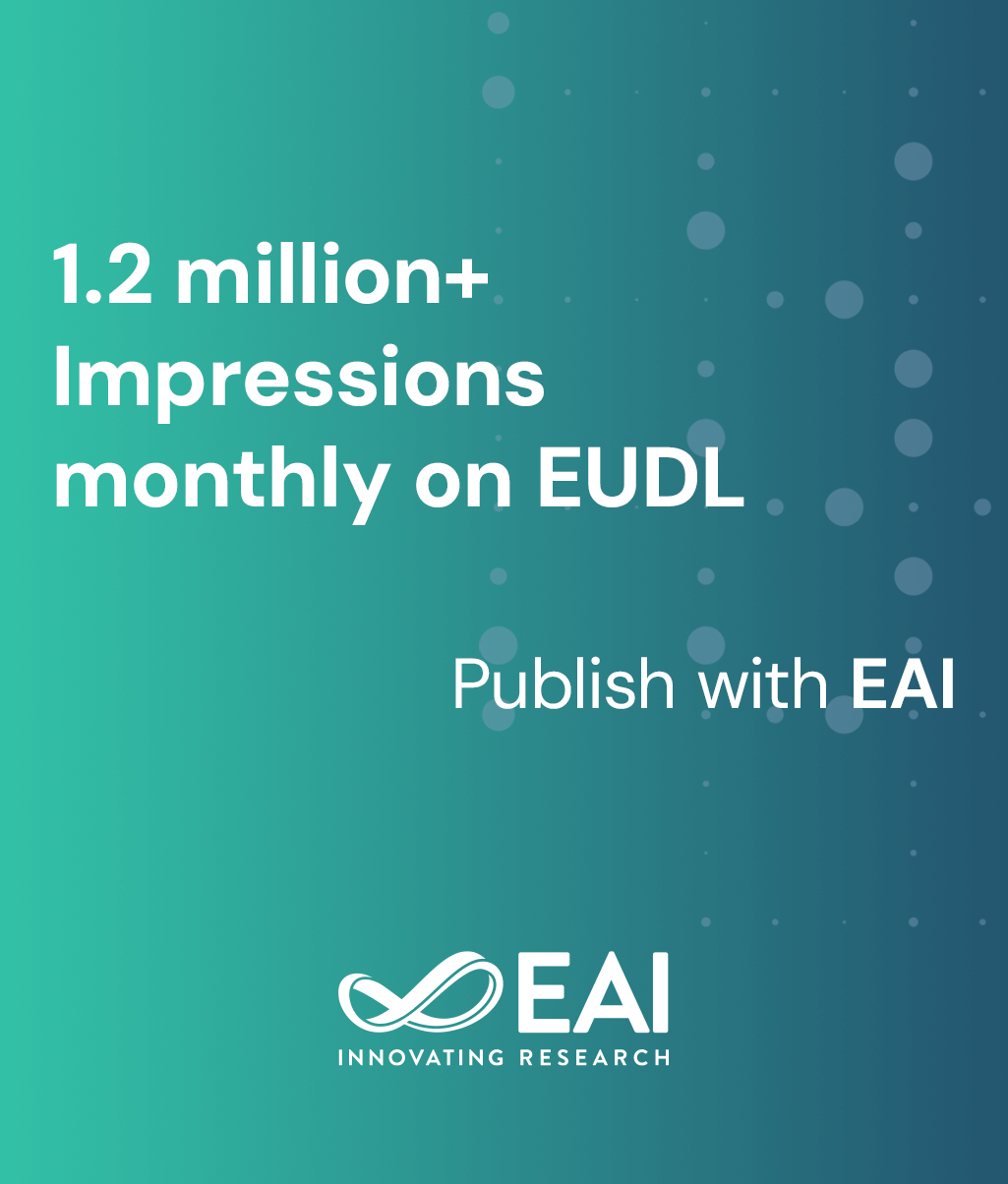
Research Article
A Fast Decoding Algorithm for Non-orthogonal Frequency Division Multiplexing Signals
@INPROCEEDINGS{10.1109/CHINACOM.2007.4469461, author={Xing Yang and Wenbao Ai and Tianping Shuai and Daoben Li}, title={A Fast Decoding Algorithm for Non-orthogonal Frequency Division Multiplexing Signals}, proceedings={2nd International ICST Conference on Communications and Networking in China}, publisher={IEEE}, proceedings_a={CHINACOM}, year={2008}, month={3}, keywords={AWGN Computational complexity Detectors Frequency division multiplexing Interference Maximum likelihood decoding Maximum likelihood detection OFDM Shape Signal detection}, doi={10.1109/CHINACOM.2007.4469461} }
- Xing Yang
Wenbao Ai
Tianping Shuai
Daoben Li
Year: 2008
A Fast Decoding Algorithm for Non-orthogonal Frequency Division Multiplexing Signals
CHINACOM
IEEE
DOI: 10.1109/CHINACOM.2007.4469461
Abstract
A fast decoding algorithm based on Semidefinite relaxation combined with cutting plane is proposed for the detection of Non-orthogonal frequency division multiplexing (NFDM) signals. It shows that maximum likelihood (ML) detection of NFDM signals can be transformed into a semidefinite programming problem by relaxing rank one constraint. In order to tighten the performance gap between the relaxed combinatorial optimization problem and the optimum ML detection one, adding cutting planes are necessary. Simulations show that the optimum ML performance can be approached by the proposed algorithm. The loss in signal-to-noise ratio (SNR) compared with the ML detector is within 1dB for the worst case. Moreover, the proposed algorithm’s computational complexity is only proportional to a polynomial of the number of subcarriers rather than an exponential one of the optimum ML algorithm.