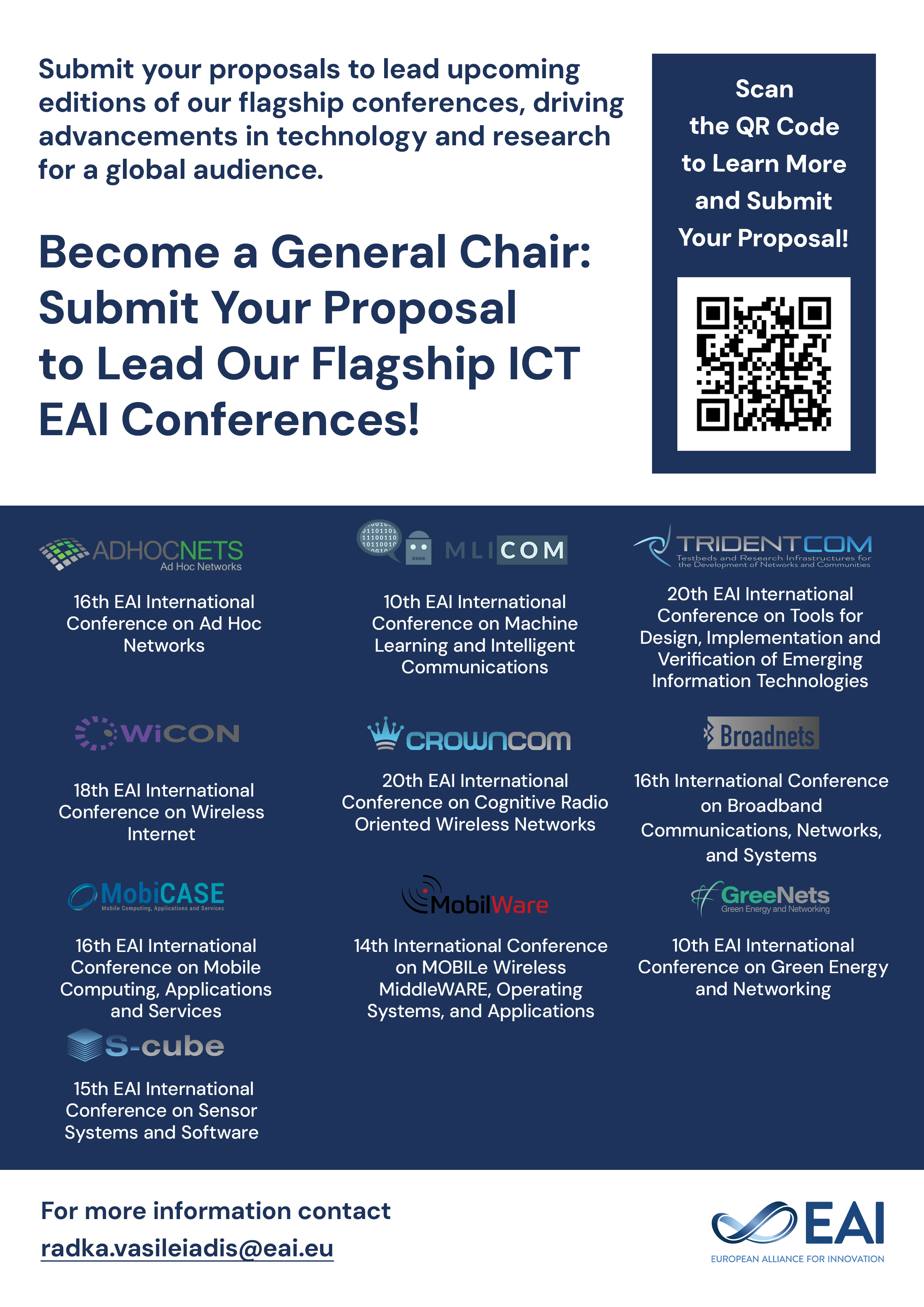
Research Article
Use of Novel Feature Extraction Technique with Subspace Classifiers for Speech Recognition
@INPROCEEDINGS{10.1109/PERSER.2007.4283894, author={Serkan Gunal and Rifat Edizkan}, title={Use of Novel Feature Extraction Technique with Subspace Classifiers for Speech Recognition}, proceedings={1st International IEEE Conference on Pervasive Services}, publisher={IEEE}, proceedings_a={ICPS}, year={2007}, month={8}, keywords={Continuous wavelet transforms Data engineering Feature extraction Fourier transforms Humans Linear predictive coding Mel frequency cepstral coefficient Speech recognition Wavelet analysis Wavelet transforms}, doi={10.1109/PERSER.2007.4283894} }
- Serkan Gunal
Rifat Edizkan
Year: 2007
Use of Novel Feature Extraction Technique with Subspace Classifiers for Speech Recognition
ICPS
IEEE
DOI: 10.1109/PERSER.2007.4283894
Abstract
Speech recognition is one of the fast moving research areas in pervasive services requiring human interaction. Like any type of pattern recognition system, selection of the feature extraction method and the classifier play a crucial role for speech recognition in terms of accuracy and speed. In this paper, an efficient wavelet based feature extraction method for speech data is presented. The feature vectors are then fed into three widely used linear subspace classifiers for recognition analysis. These classifiers are Class Featuring Information Compression (CLAFIC), Multiple Similarity Method (MSM) and Common Vector Approach (CVA). TI-DIGIT database is used to evaluate the performance of speaker independent isolated word recognition system designed. Experimental results indicate that the proposed feature extraction method together with the CLAFIC and CVA classifiers give considerably high recognition rates.