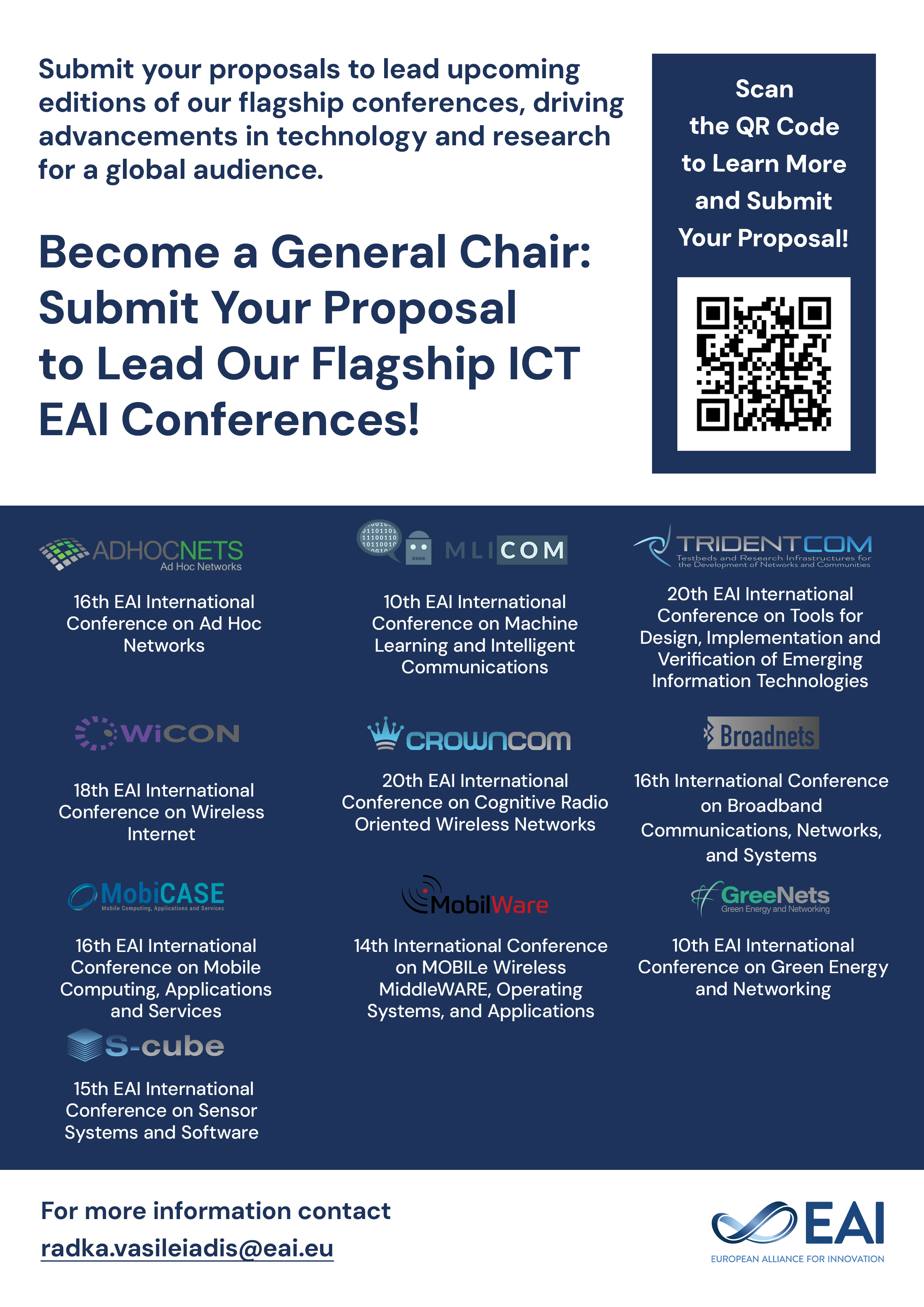
Research Article
Generative Models for Fingerprint Individuality using Ridge Types
@INPROCEEDINGS{10.1109/IAS.2007.32, author={Gang Fang and Sargur N. Srihari and Harish Srinivasan}, title={Generative Models for Fingerprint Individuality using Ridge Types}, proceedings={1st International ICST Workshop on Computational Forensics}, publisher={IEEE}, proceedings_a={IWCF}, year={2007}, month={9}, keywords={Fingerprint recognition Gaussian distribution Information analysis Information security Parameter estimation Partial response channels Pattern analysis Pattern recognition Spatial databases Text analysis}, doi={10.1109/IAS.2007.32} }
- Gang Fang
Sargur N. Srihari
Harish Srinivasan
Year: 2007
Generative Models for Fingerprint Individuality using Ridge Types
IWCF
IEEE
DOI: 10.1109/IAS.2007.32
Abstract
Generative models of pattern individuality attempt to represent the distribution of observed quantitative features, e.g., by learning parameters from a database, and then use such distributions to determine the probability of two random patterns being the same. Considering fingerprint patterns, Gaussian distributions have been previously used for minutiae location and von-Mises distributions for minutiae orientation so as to determine the probability of random correspondence (PRC) between two fingerprints. Motivated by the fact that ridges have not been modeled in generative models, and using representative ridge points in fingerprint matching, ridge information is incorporated into the generative model by using a third distribution for ridge types. The joint probability of minutiae location, minutiae orientation and ridge type is modeled as a mixture distribution. The proposed model offers a more accurate fingerprint representation from which more reliable PRCs can be computed. Based on parameters estimated from fingerprint databases, PRCs using ridge types are seen to be much smaller than PRCs computed with only minutiae