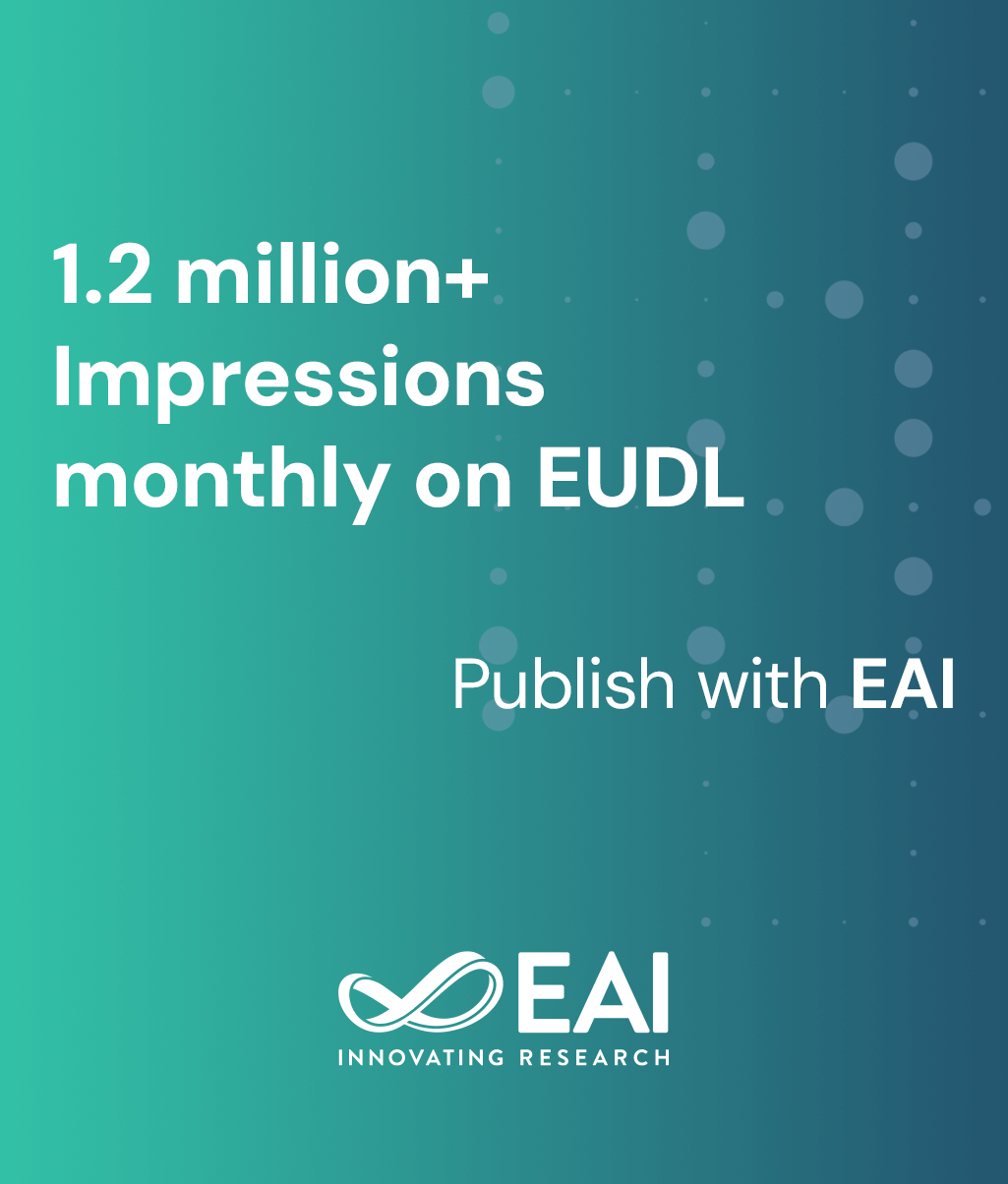
Research Article
Fast Fourier Transform based IP Traffic Classification System for SIPTO at H(e)NB
@INPROCEEDINGS{10.1109/ChinaCom.2012.6417521, author={Lin Han and Liusheng Huang and Qian Hu and Xue Han and Jinglin Shi}, title={Fast Fourier Transform based IP Traffic Classification System for SIPTO at H(e)NB}, proceedings={7th International Conference on Communications and Networking in China}, publisher={IEEE}, proceedings_a={CHINACOM}, year={2012}, month={10}, keywords={fft classification sipto h(e)nb}, doi={10.1109/ChinaCom.2012.6417521} }
- Lin Han
Liusheng Huang
Qian Hu
Xue Han
Jinglin Shi
Year: 2012
Fast Fourier Transform based IP Traffic Classification System for SIPTO at H(e)NB
CHINACOM
IEEE
DOI: 10.1109/ChinaCom.2012.6417521
Abstract
3GPP has recently introduced LIPA(Local IP Access) and SIPTO(Selected IP Traffic Offload) to offload traffic from the core network, which brings new challenge to on-line traffic classification, because of the large amount of data and the difference of mobile network from wired network, such as high bit error rates(BER) and temporary disconnections. Therefore, other proposed schemes which aim at ether LIPA at H(e)NB or SIPTO at macro network could not get high accuracy and high speed at the same time, and traffic classification methodologies in wired IP network are not applicable. This paper proposes a fast fourier transform(FFT) based IP traffic classification system for SIPTO at H(e)NB, which focuses on classifying each packet at H(e)NB by extracting the application layer payload pattern using FFT. Pattern extraction and classification using machine learning algorithms are simulated, and results show that our system outperforms existing methods by offering about 3%-6% improvement in classification accuracy with about 7% time. Simulation of SIPTO shows good reduction of press to the core network and low false rates.