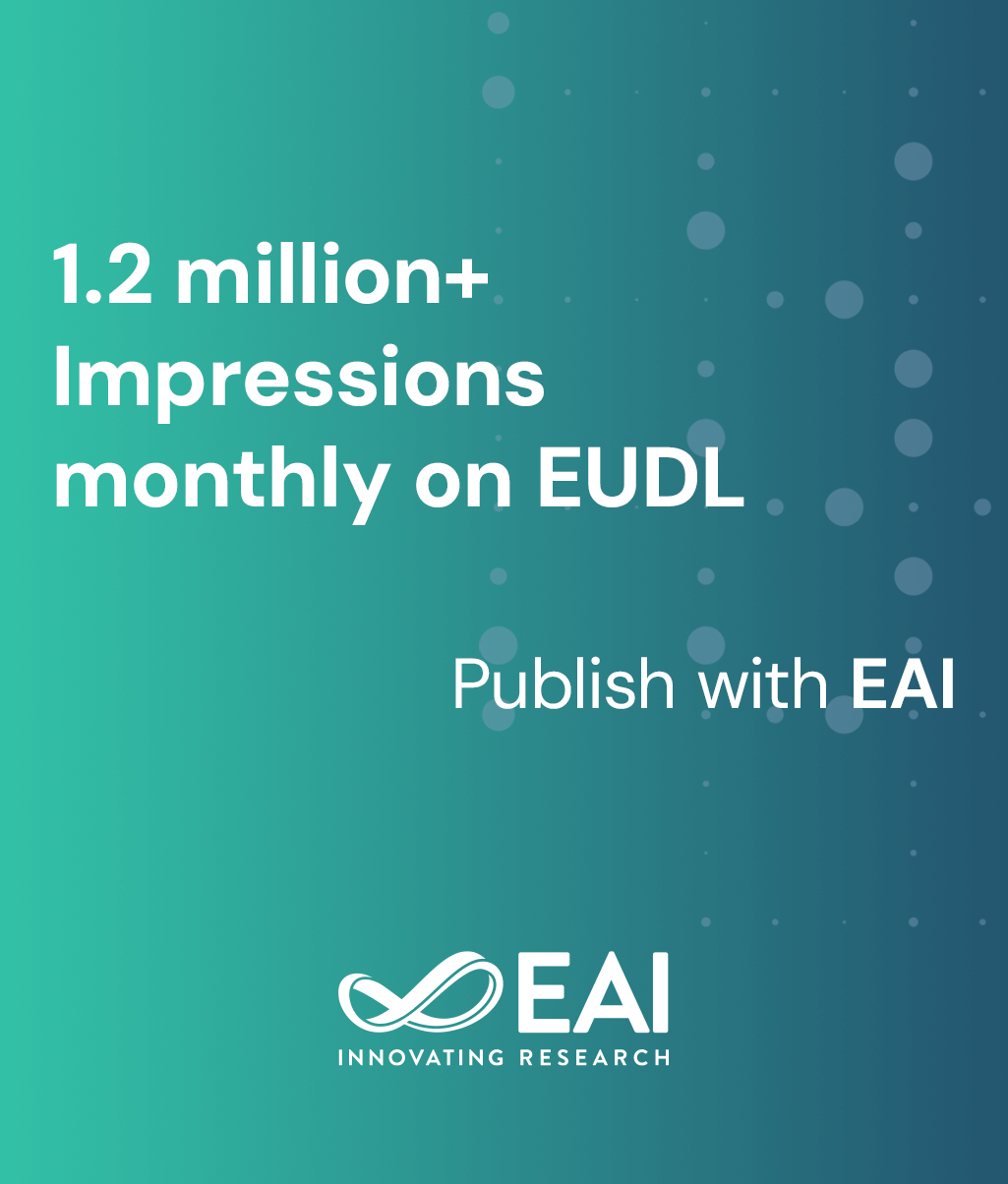
Research Article
Effects of Agendas on Model-based Intention Inference of Cooperative Teams
@INPROCEEDINGS{10.1109/COLCOM.2007.4553875, author={Martin Giersich and Thomas Kirste}, title={Effects of Agendas on Model-based Intention Inference of Cooperative Teams}, proceedings={3rd International ICST Conference on Collaborative Computing: Networking, Applications and Worksharin}, publisher={IEEE}, proceedings_a={COLLABORATECOM}, year={2008}, month={6}, keywords={Accuracy Bayesian methods Computational modeling Computer science Filtering Hidden Markov models Intelligent sensors Particle filters Predictive models Working environment noise}, doi={10.1109/COLCOM.2007.4553875} }
- Martin Giersich
Thomas Kirste
Year: 2008
Effects of Agendas on Model-based Intention Inference of Cooperative Teams
COLLABORATECOM
IEEE
DOI: 10.1109/COLCOM.2007.4553875
Abstract
Ubiquitous computing aims for the realization of environments that assist users autonomously and proactively. Therefore smart environment infrastructures need to be able to identify users needs (intention recognition) and to plan an appropriate assisting strategy. Both is matter for research. In our approach we address inferring the intention of a team within a smart meeting environment. This becomes a central challenge, especially if multiple users are observed by noisy heterogeneous sensors. We propose a team behavior model based on hierarchical dynamic Bayesian network (DBN) for inferring the current task and activity of a team of users online. Given (noisy and intermittent) sensor readings of the team members’ positions in a meeting room, we are interested in inferring the team’s current objective. We implemented the model using particle filters for inference and demonstrate that by adding knowledge about the meeting agenda prediction accuracy and speed is improved. Evaluation of simulation data answers the question, how precise agenda knowledge must be to predict team behavior optimally.