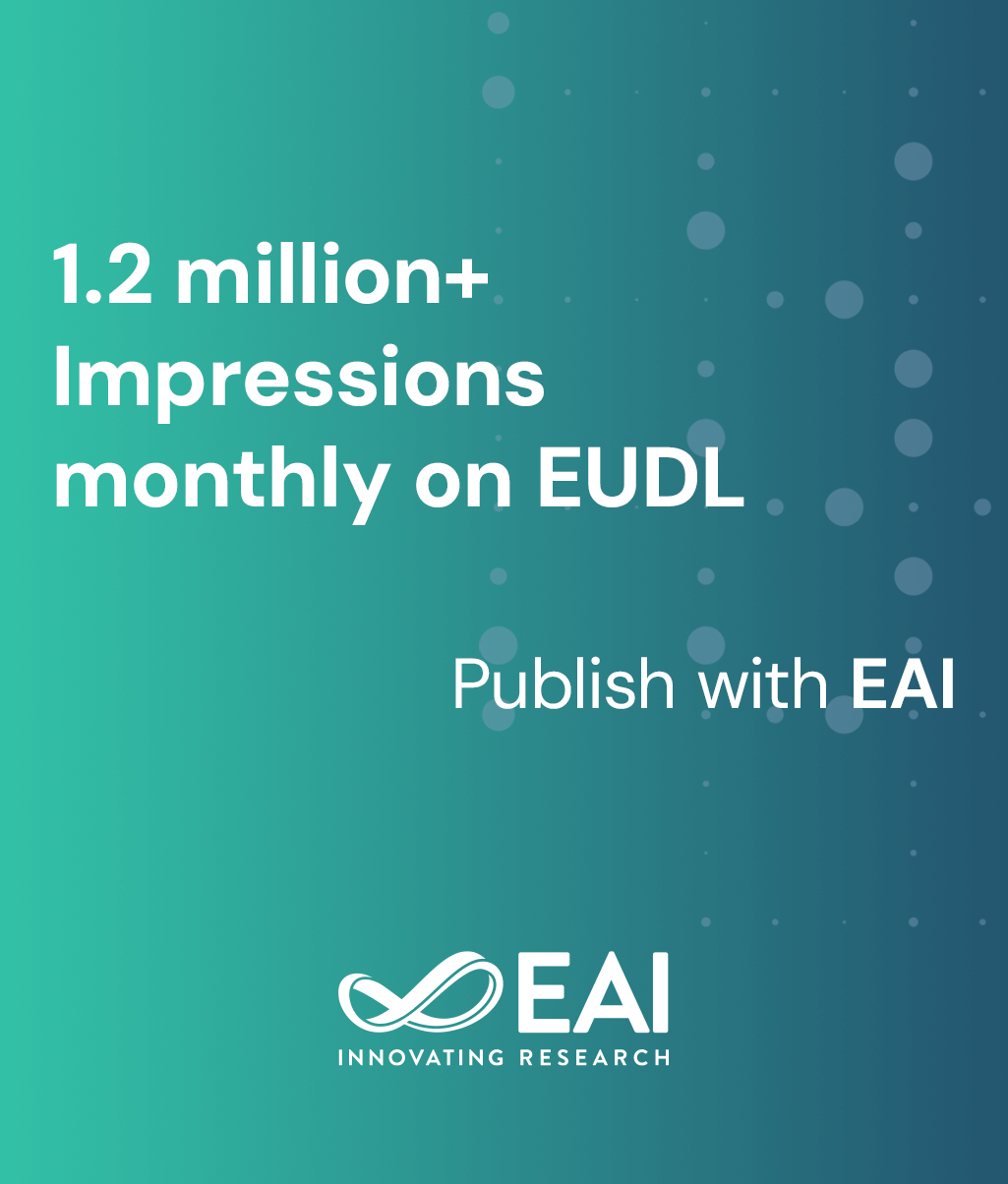
Research Article
Fast and Effective Spam Sender Detection with Granular SVM on Highly Imbalanced Mail Server Behavior Data
@INPROCEEDINGS{10.1109/COLCOM.2006.361856, author={Yuchun Tang and Sven Krasser and Paul Judge and Yan-Qing Zhang}, title={Fast and Effective Spam Sender Detection with Granular SVM on Highly Imbalanced Mail Server Behavior Data}, proceedings={2nd International ICST Conference on Collaborative Computing: Networking, Applications and Worksharing}, publisher={IEEE}, proceedings_a={COLLABORATECOM}, year={2007}, month={5}, keywords={spam filtering data mining class imbalance granular support vector machine}, doi={10.1109/COLCOM.2006.361856} }
- Yuchun Tang
Sven Krasser
Paul Judge
Yan-Qing Zhang
Year: 2007
Fast and Effective Spam Sender Detection with Granular SVM on Highly Imbalanced Mail Server Behavior Data
COLLABORATECOM
IEEE
DOI: 10.1109/COLCOM.2006.361856
Abstract
Unsolicited commercial or bulk emails or emails containing virus currently pose a great threat to the utility of email communications. A recent solution for filtering is reputation systems that can assign a value of trust to each IP address sending email messages. By analyzing the query patterns of each participating node, reputation systems can calculate a reputation score for each queried IP address and serve as a platform for global collaborative spam filtering for all participating nodes. In this research, we explore a behavioral classification approach based on spectral sender characteristics retrieved from such global messaging patterns. Due to the large amount of bad senders, this classification task has to cope with highly imbalanced data. In order to solve this challenging problem, a novel granular support vector machine - boundary alignment algorithm (GSVM-BA) is designed. GSVM-BA looks for the optima] decision boundary by repetitively removing positive support vectors from the training dataset and rebuilding another SVM. Compared to the original SVM algorithm with cost-sensitive learning, GSVM-BA demonstrates superior performance on spam IP detection, in terms of both effectiveness and efficiency.