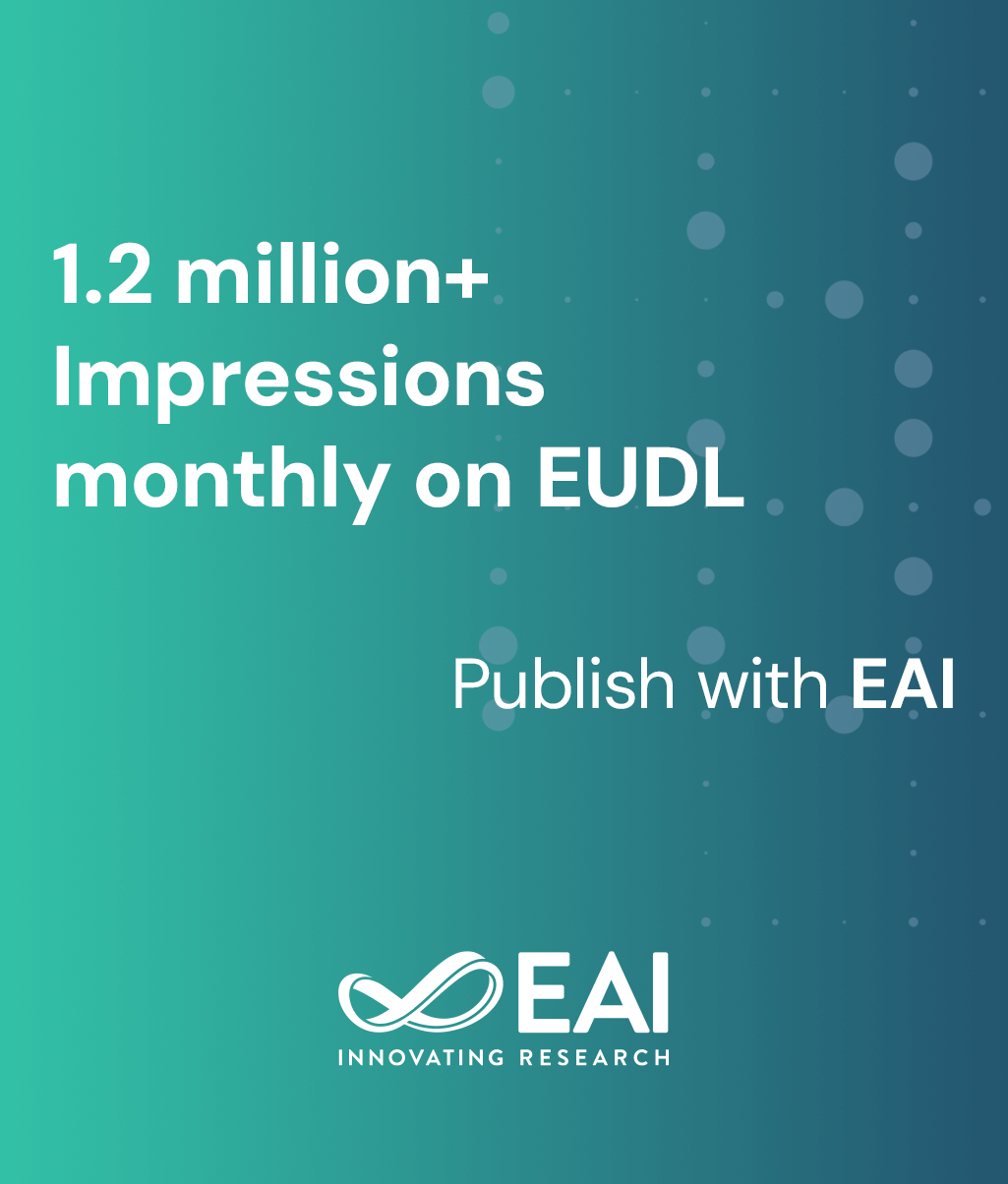
Research Article
An experimental evaluation of spam filter performance and robustness against attack
@INPROCEEDINGS{10.1109/COLCOM.2005.1651219, author={Steve Webb and Subramanyam Chitti and Calton Pu}, title={An experimental evaluation of spam filter performance and robustness against attack}, proceedings={1st International Conference on Collaborative Computing: Networking, Applications and Worksharing}, publisher={IEEE}, proceedings_a={COLLABORATECOM}, year={2006}, month={7}, keywords={Collaboration Degradation Educational institutions High performance computing Information filtering Information filters Large-scale systems Robustness Support vector machines Unsolicited electronic mail}, doi={10.1109/COLCOM.2005.1651219} }
- Steve Webb
Subramanyam Chitti
Calton Pu
Year: 2006
An experimental evaluation of spam filter performance and robustness against attack
COLLABORATECOM
IEEE
DOI: 10.1109/COLCOM.2005.1651219
Abstract
In this paper, we show experimentally that learning filters are able to classify large corpora of spam and legitimate email messages with a high degree of accuracy. The corpora in our experiments contain about half a million spam messages and a similar number of legitimate messages, making them two orders of magnitude larger than the corpora used in current research. The use of such large corpora represents a collaborative approach to spam filtering because the corpora combine spam and legitimate messages from many different sources. First, we show that this collaborative approach creates very accurate spam filters. Then, we introduce an effective attack against these filters which successfully degrades their ability to classify spam. Finally, we present an effective solution to the above attack which involves retraining the filters to accurately identify the attack messages.