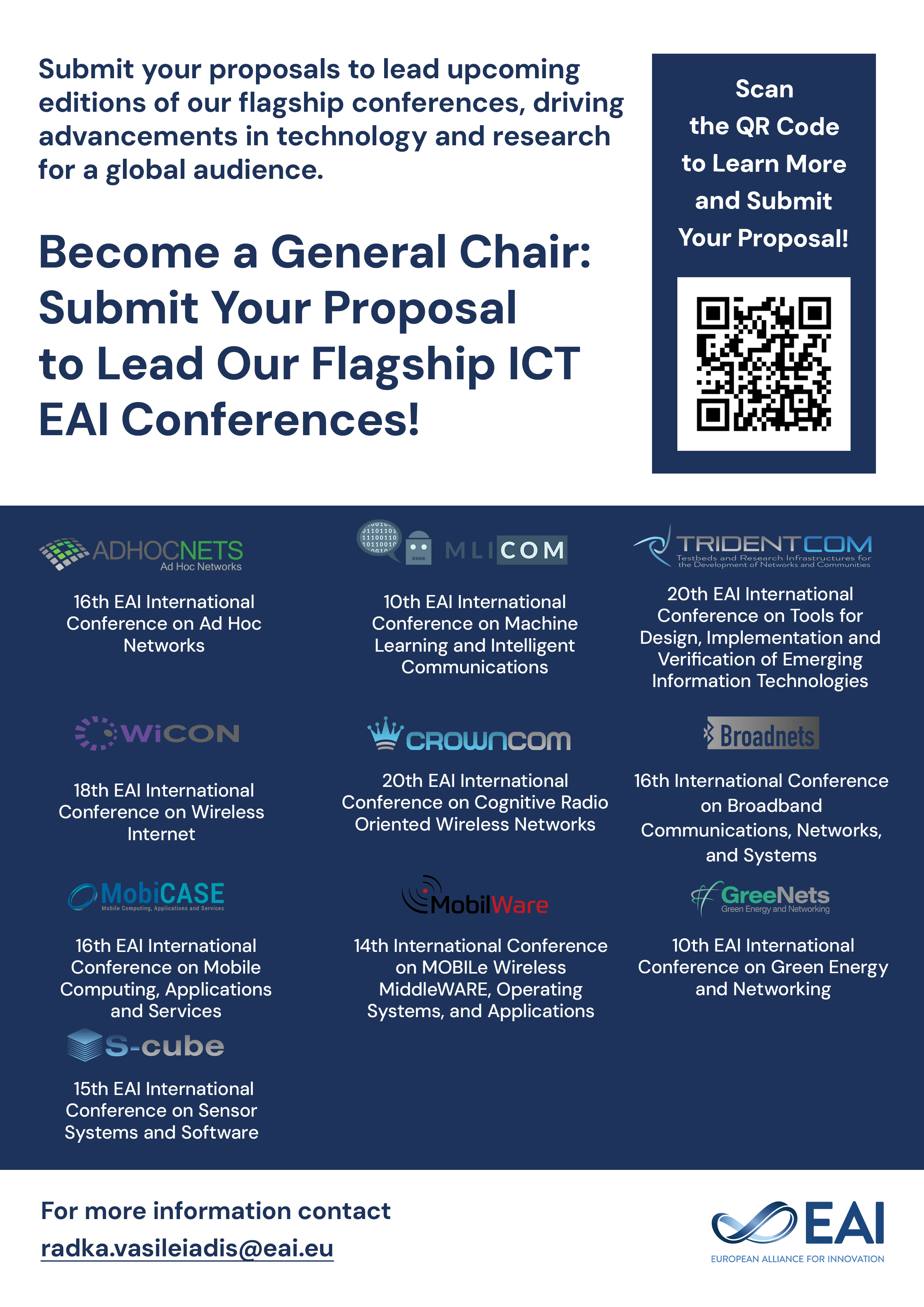
Research Article
Internet Service Fault Management using Active Probing in Uncertain and Noisy Environment
@INPROCEEDINGS{10.1109/CHINACOM.2009.5339962, author={L.W. Chu and S.H. Zou and S.D. Cheng and W.D. Wang and C.Q. Tian}, title={Internet Service Fault Management using Active Probing in Uncertain and Noisy Environment}, proceedings={ChinaCom2009-Advances in Internet Symposium}, publisher={IEEE}, proceedings_a={CHINACOM2009-AIS}, year={2009}, month={11}, keywords={service management; fault management; active probing; dependency model; bipartite Bayesian network.}, doi={10.1109/CHINACOM.2009.5339962} }
- L.W. Chu
S.H. Zou
S.D. Cheng
W.D. Wang
C.Q. Tian
Year: 2009
Internet Service Fault Management using Active Probing in Uncertain and Noisy Environment
CHINACOM2009-AIS
IEEE
DOI: 10.1109/CHINACOM.2009.5339962
Abstract
The great challenges of Internet service fault management are uncertainty and noise. To address these challenges, we model the service scenario through a multi-layer management model, and propose an approach using active probing to detect and diagnose faults. This approach uses bipartite Bayesian network as dependency model, binary symmetric channel as noise model, and is composed of two phases: fault detection and fault diagnosis. In first phase, we propose a greedy approximation probe selection algorithm (GAPSA), which selects a minimal set of probes while remaining a high probability of fault detection. In second phase, we propose a fault diagnosis probe selection algorithm (FDPSA), which selects probes to obtain more system information based on symptoms observed in previous phase. Simulation results prove the validity and efficiency of our approach..