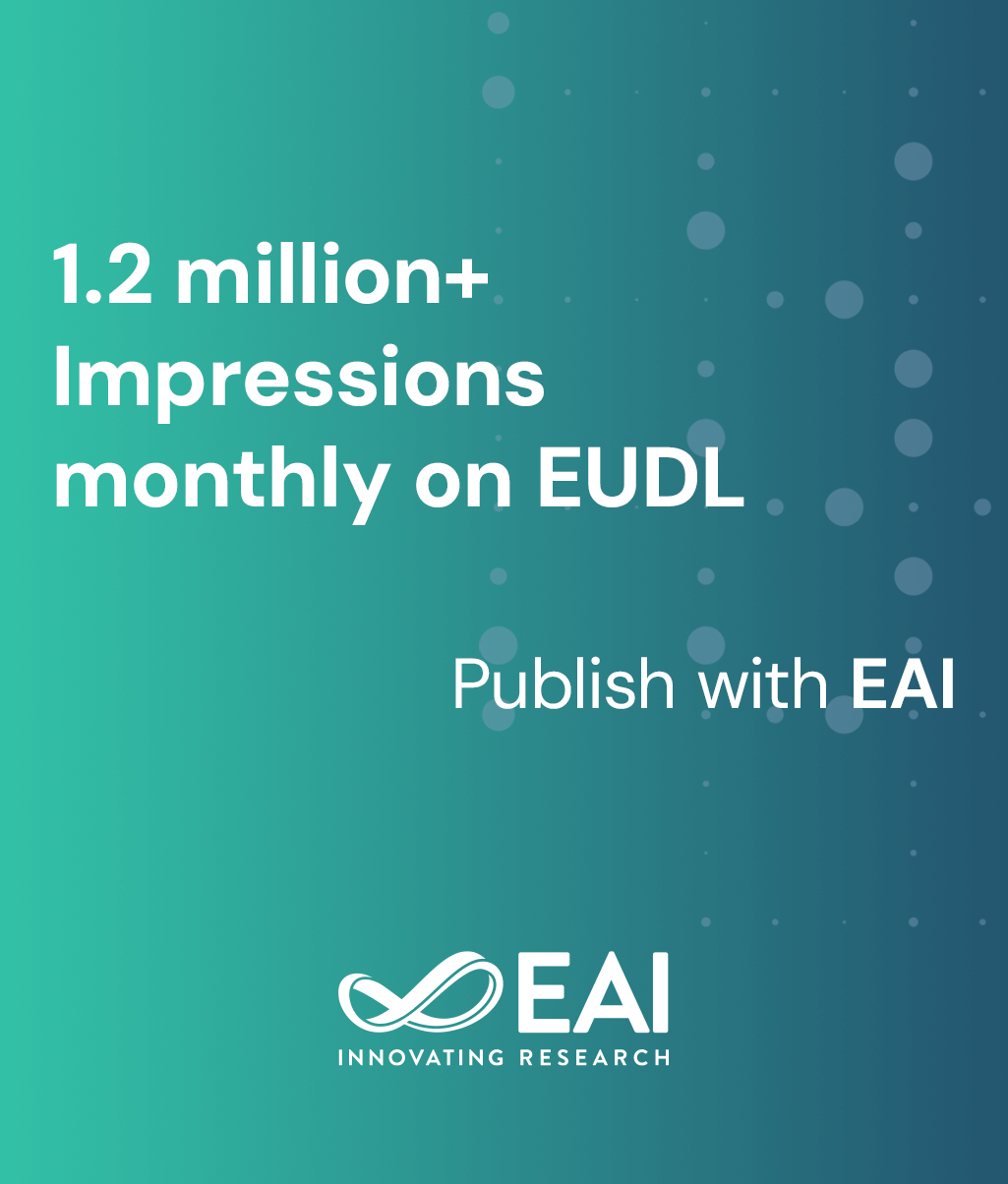
Research Article
An Unsupervised Anomaly Detection Approach using Subtractive Clustering and Hidden Markov Model
@INPROCEEDINGS{10.1109/CHINACOM.2007.4469390, author={Chun Yang and Feiqi Deng and Haidong Yang}, title={An Unsupervised Anomaly Detection Approach using Subtractive Clustering and Hidden Markov Model}, proceedings={2nd International ICST Conference on Communications and Networking in China}, publisher={IEEE}, proceedings_a={CHINACOM}, year={2008}, month={3}, keywords={Hidden Markov Model feature selection intrusion detection subtractive clustering}, doi={10.1109/CHINACOM.2007.4469390} }
- Chun Yang
Feiqi Deng
Haidong Yang
Year: 2008
An Unsupervised Anomaly Detection Approach using Subtractive Clustering and Hidden Markov Model
CHINACOM
IEEE
DOI: 10.1109/CHINACOM.2007.4469390
Abstract
Previous Research in network intrusion detection system (NIDS) has typically used misuse detection or supervised anomaly detection techniques. These techniques have difficulty in detecting new types of attacks or causing high false positives in real network environment. Unsupervised anomaly detection can overcome the drawbacks of misuse detection and supervised anomaly detection. In this paper, normal-anomaly patterns are built over the network traffic dataset that uses subtractive clustering, and at the same time the built Hidden Markov Model (HMM) correlates the observation sequences and state transitions to predict the most probable intrusion state sequences. The proposed unsupervised anomaly detection approach is capable of reducing false positives by classifying intrusion sequences into different emergency levels. The experimental results are also reported using the KDDCup’99 dataset and Matlab.