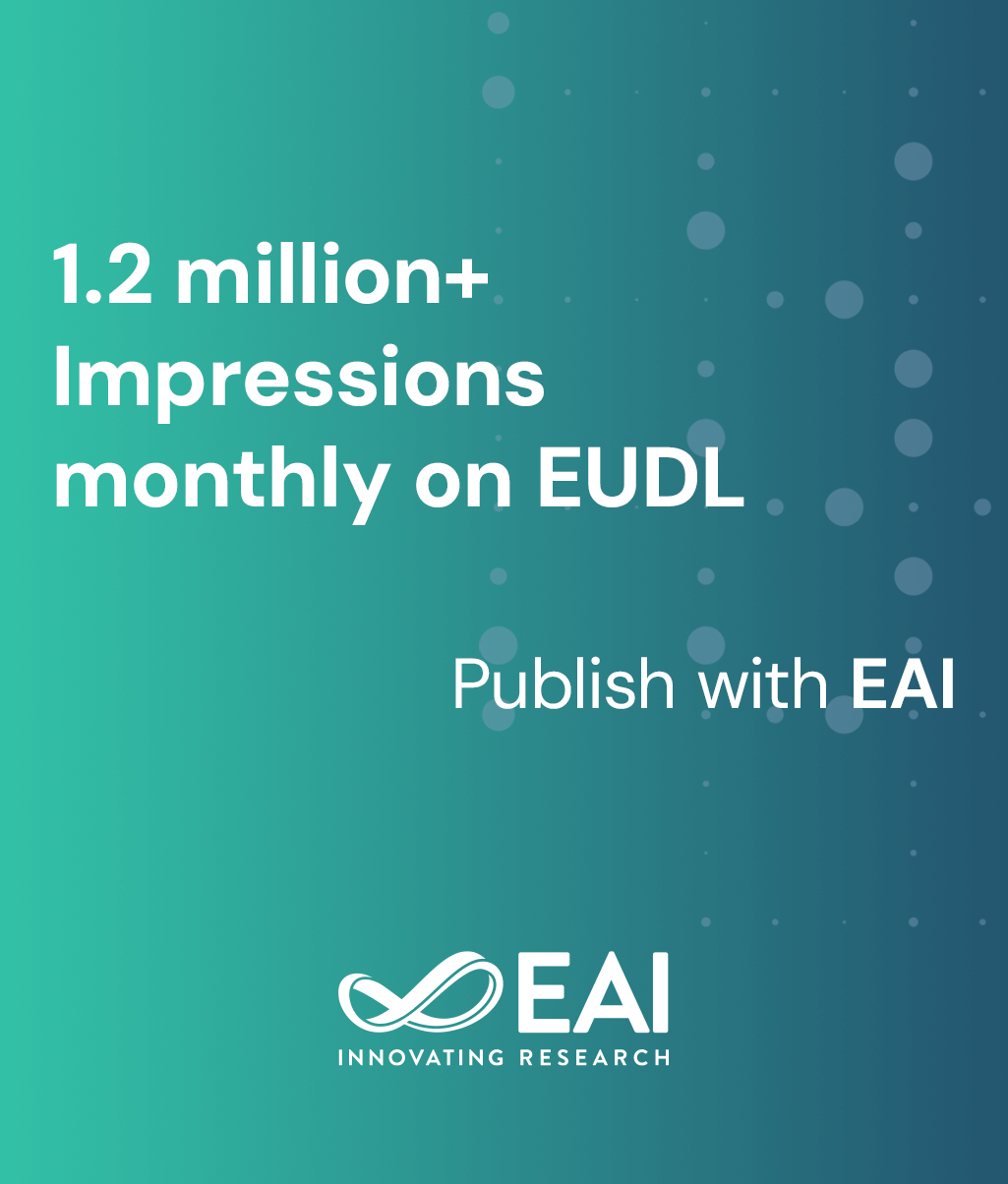
Research Article
Change Detection from Satellite Images Using PNN
@INPROCEEDINGS{10.1007/978-3-642-37949-9_29, author={Akansha Mehrotra and Krishna Singh and Kirat Pal and M. Nigam}, title={Change Detection from Satellite Images Using PNN}, proceedings={Quality, Reliability, Security and Robustness in Heterogeneous Networks. 9th International Conference, QShine 2013, Greader Noida, India, January 11-12, 2013, Revised Selected Papers}, proceedings_a={QSHINE}, year={2013}, month={7}, keywords={Change detection Probability density function Probabilistic neural network (PNN)}, doi={10.1007/978-3-642-37949-9_29} }
- Akansha Mehrotra
Krishna Singh
Kirat Pal
M. Nigam
Year: 2013
Change Detection from Satellite Images Using PNN
QSHINE
Springer
DOI: 10.1007/978-3-642-37949-9_29
Abstract
This paper presents a supervised change detection technique for satellite images using a probabilistic neural network (PNN). The proposed method works in two phases. In the first phase a difference image is computed. The most commonly used techniques for computing the difference image such as ratio images or log ratio images degrade the performance of the algorithm in the presence of speckle noise. To overcome the above mentioned limitations the difference image in this work is computed using normalized neighborhood ratio based method. In the next phase the PNN is used to detect efficiently any change between the two images. An estimator is used by the PNN to estimate the probability density function. The ratio of two conditional probability density functions, called the likelihood ratio is computed. Finally, the log likelihood ratio test is used to classify the pixels of the difference image into changed and unchanged classes to create a change map. The change map highlights the changes that have occurred between the two input images. The proposed method was compared quantatively as well as qualitatively with other existing state of the art methods. The results showed that the proposed method outperforms the other methods.