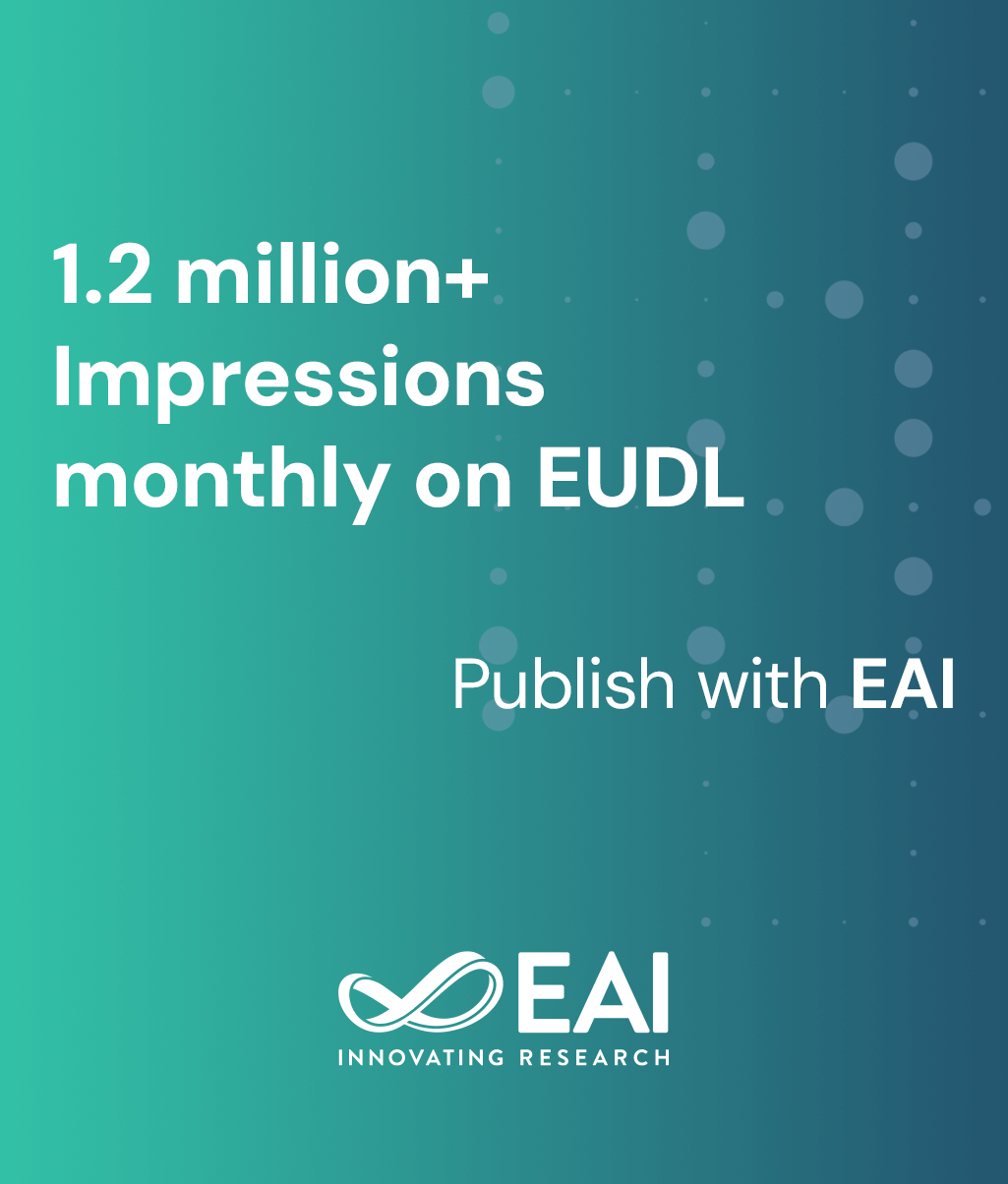
Research Article
Performance Evaluation of Evolutionary and Decision Tree Based Classifiers in Diversity of Datasets
@INPROCEEDINGS{10.1007/978-3-642-35615-5_47, author={Pardeep Kumar and Vivek Sehgal and Nitin and Durg Chauhan}, title={Performance Evaluation of Evolutionary and Decision Tree Based Classifiers in Diversity of Datasets}, proceedings={Third International conference on advances in communication, network and computing}, proceedings_a={CNC}, year={2012}, month={12}, keywords={Knowledge Discovery in Databases Evolutionary Computation Information Gain Classification}, doi={10.1007/978-3-642-35615-5_47} }
- Pardeep Kumar
Vivek Sehgal
Nitin
Durg Chauhan
Year: 2012
Performance Evaluation of Evolutionary and Decision Tree Based Classifiers in Diversity of Datasets
CNC
Springer
DOI: 10.1007/978-3-642-35615-5_47
Abstract
The large databases of digital information are ubiquitous. Data from the neighborhood store’s checkout register, your bank’s credit card authorization device, records in your doctor’s office, patterns in your telephone calls and many more applications generate streams of digital records archived in huge databases, sometimes in so-called data warehouses A new generation of computational techniques and tools is required to support the extraction of useful knowledge from the rapidly growing volumes of data. These techniques and tools are the subject of the emerging field of knowledge discovery in databases (KDD) and data mining. Data mining plays an important role to discover important information to help in decision making of a decision support system. It has been the active area of research in the last decade. The classification is one of the important tasks of data mining. Different kind of classifiers have been suggested and tested to predict the future events based on unseen data. This paper compares the performance evaluation of evolutionary based genetic algorithm and decision tree based classifiers in diversity of datasets. The performance evaluation metrics are predictive accuracy, training time and comprehensibility. Evolutionary based classifier shows better comprehensibility over decision tree based classifiers. These classifiers show almost same predictive accuracy. Experimental results demonstrate that evolutionary approach based classifiers are slower than decision tree based classifiers. This research is helpful for organizations to select the classifiers as information generator for their decision support systems to make future policies.