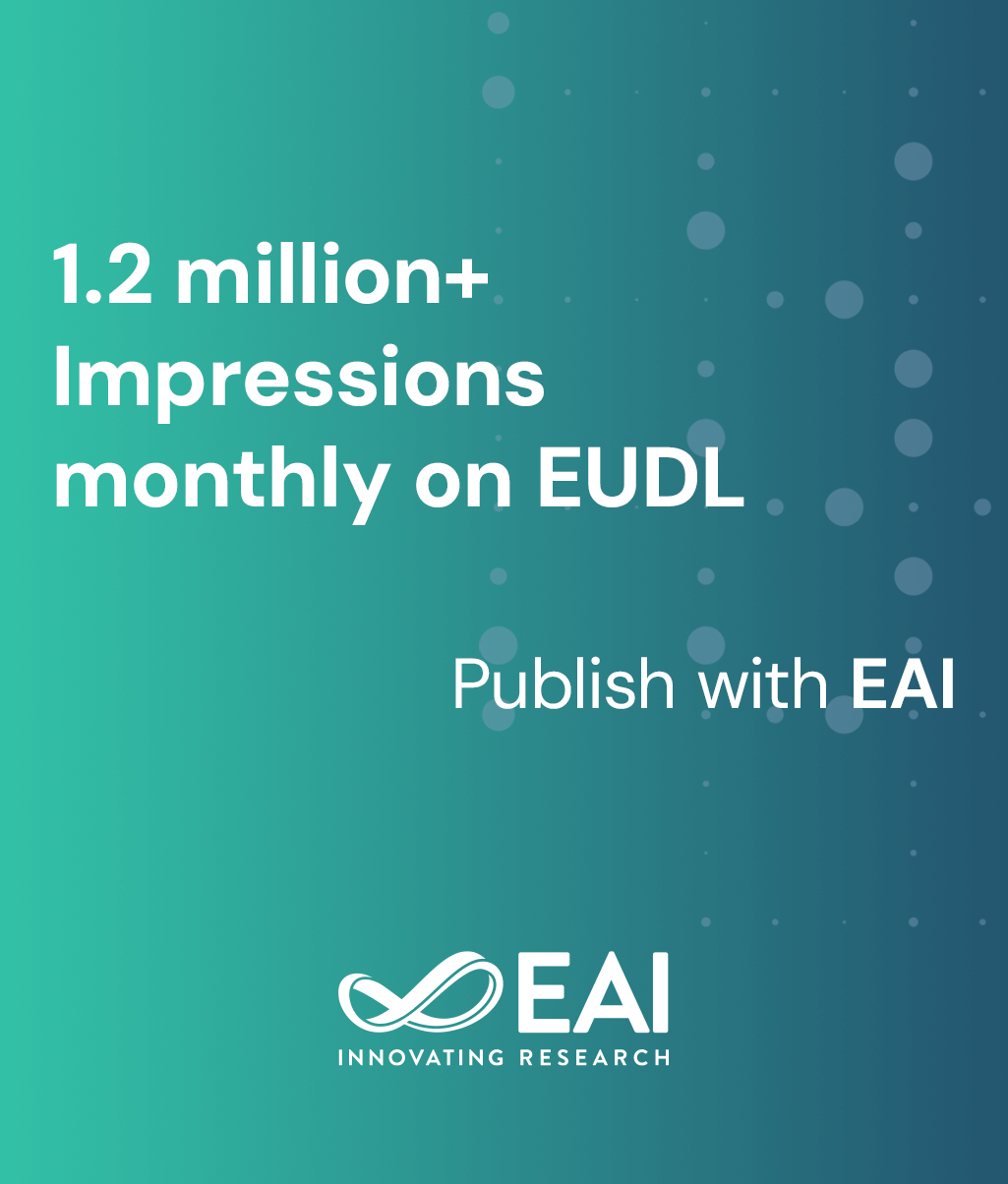
Research Article
Applications of Hidden Markov Model to Recognize Handwritten Tamil Characters
@INPROCEEDINGS{10.1007/978-3-642-35615-5_43, author={R. Kannan and R. Suresh and A. Selvakumar}, title={Applications of Hidden Markov Model to Recognize Handwritten Tamil Characters}, proceedings={Third International conference on advances in communication, network and computing}, proceedings_a={CNC}, year={2012}, month={12}, keywords={Optical Character Recognition Hidden Markov Model Preprocessing Binarization Segmentation Feature Extraction Preliminary Classification Training and Recognition}, doi={10.1007/978-3-642-35615-5_43} }
- R. Kannan
R. Suresh
A. Selvakumar
Year: 2012
Applications of Hidden Markov Model to Recognize Handwritten Tamil Characters
CNC
Springer
DOI: 10.1007/978-3-642-35615-5_43
Abstract
Optical Character Recognition (OCR) refers to the process of converting printed, hand printed and handwritten Tamil text documents into software translated Tamil Text. As part of the preprocessing phase the image file is checked for skewing. If the image is skewed, it is corrected by a simple rotation technique. Then the image is passed through a noise elimination phase and is binarized. The preprocessed image is segmented. Thus a database of character image glyphs is created out of the segmentation phase. Then all the image glyphs are considered for recognition. Each image glyph is passed through various routines which extract the features of the glyph. The glyphs are now set ready for classification and recognition based on the above said features. The extracted features are considered for recognition using Hidden Markov Model (HMM). The recognition rate achieved is 89%.