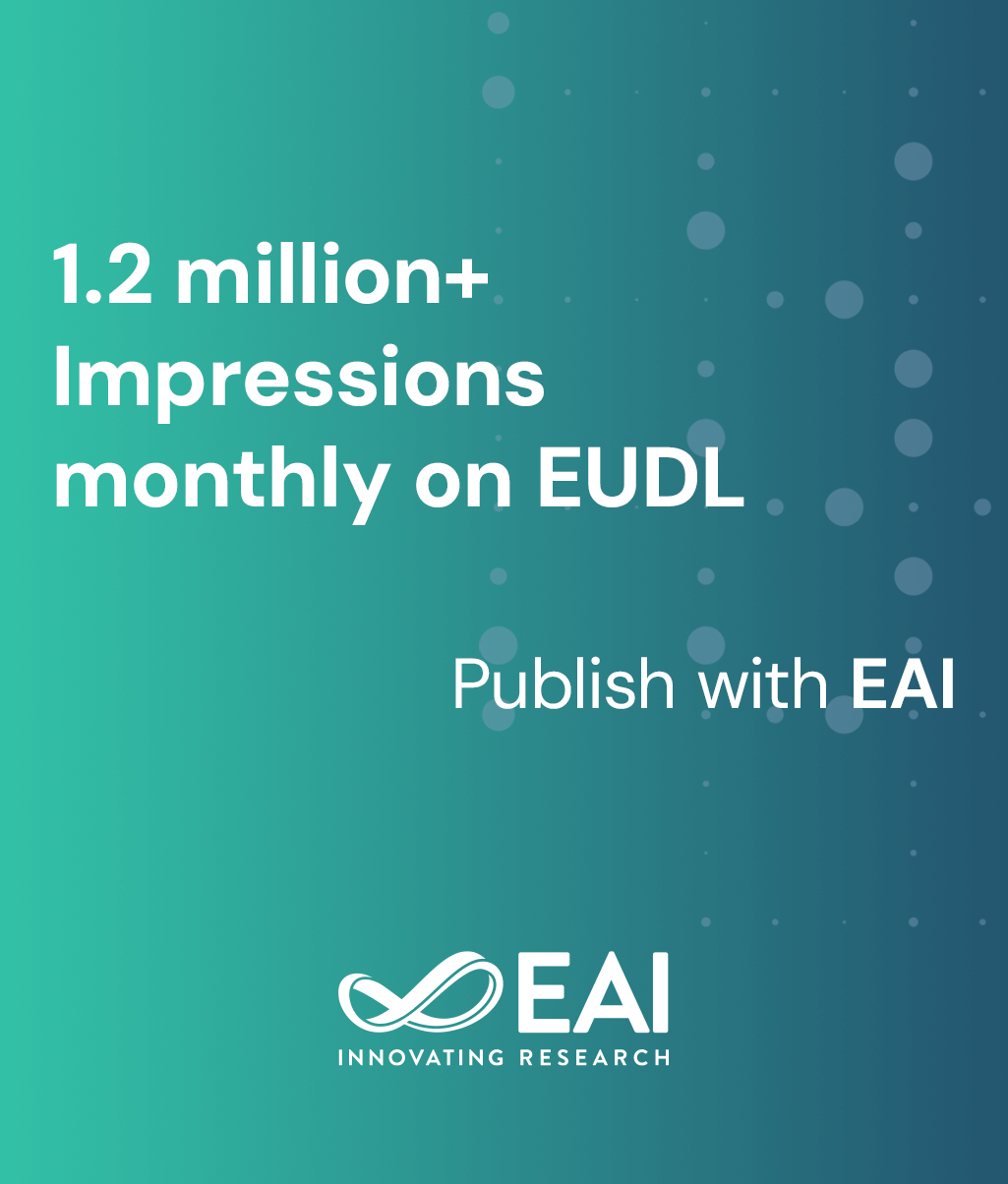
Research Article
Investigation on the Effects of ACO Parameters for Feature Selection and Classification
@INPROCEEDINGS{10.1007/978-3-642-35615-5_20, author={Shunmugapriya P. and Kanmani S. and Devipriya S. and Archana J. and Pushpa J.}, title={Investigation on the Effects of ACO Parameters for Feature Selection and Classification}, proceedings={Third International conference on advances in communication, network and computing}, proceedings_a={CNC}, year={2012}, month={12}, keywords={Optimization Ant Colony System Pheromone Pheromone Evaporation Classification Feature Selection ACO parameters}, doi={10.1007/978-3-642-35615-5_20} }
- Shunmugapriya P.
Kanmani S.
Devipriya S.
Archana J.
Pushpa J.
Year: 2012
Investigation on the Effects of ACO Parameters for Feature Selection and Classification
CNC
Springer
DOI: 10.1007/978-3-642-35615-5_20
Abstract
Ant Colony Optimization (ACO) is the most familiar meta-heuristic search algorithm and has been used in optimization of a number of feature selection (FS) problems. As a meta-heuristic search algorithm, ACO requires a set of parameters to solve the problem of feature selection. Pheromone Evaporation Rate (PER) is the most important among all these parameters. Setting up the values of these parameters is a big deal. Usually, these parameters are set up by experimenting through a number of values and finally selecting a set of values that seems to work well for feature selection. The change in optimal feature selection in accordance to different values of PER and other ACO parameters is discussed in this paper. ACO is applied for feature selection and classification of 10 datasets. From the experimental results, it can be seen that, the optimal value for the evaporation rate () lies around 0.7 leading to selection of best features and increase in classification accuracy.