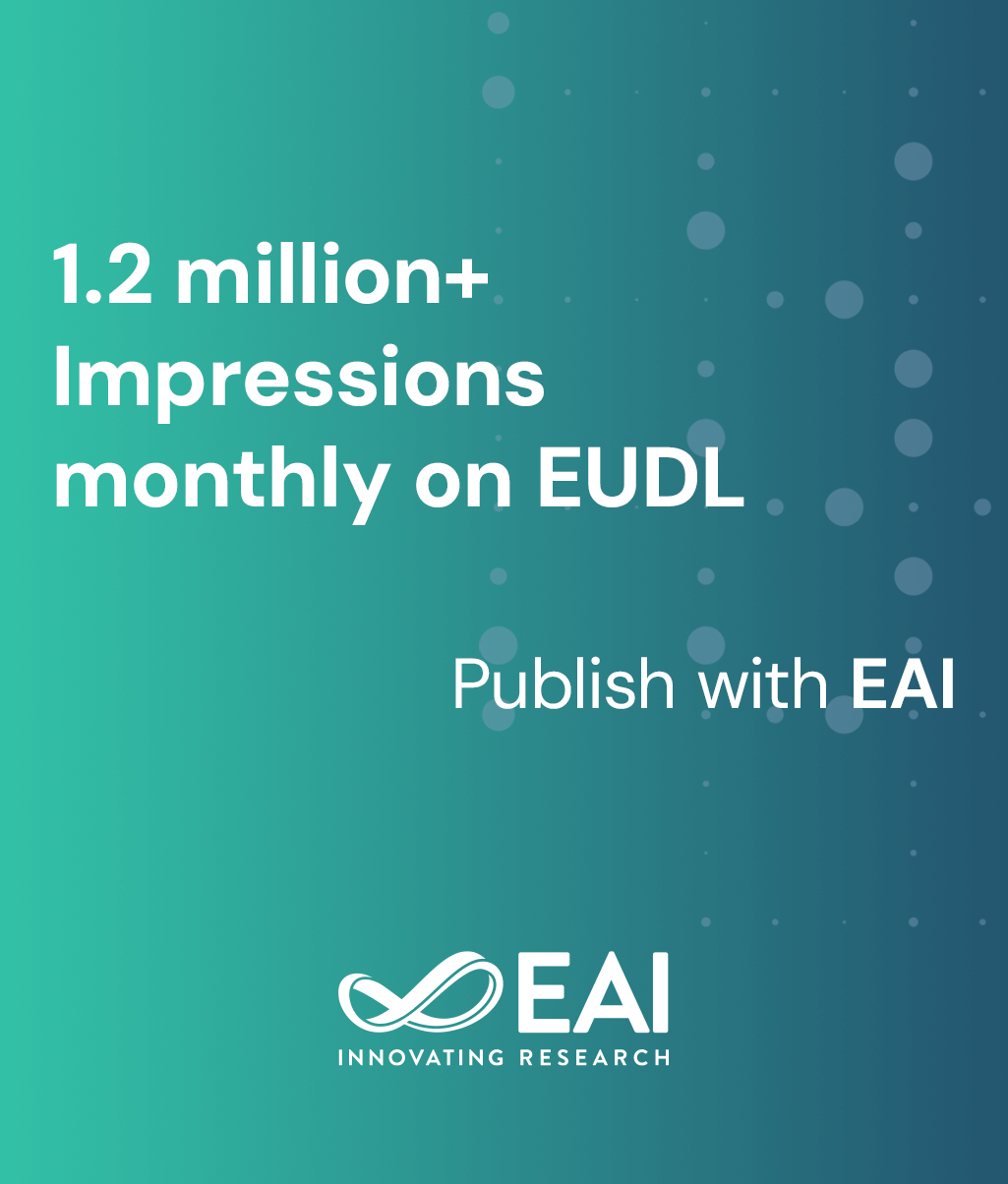
Research Article
Maximum Entropy Oriented Anonymization Algorithm for Privacy Preserving Data Mining
@INPROCEEDINGS{10.1007/978-3-642-33448-1_2, author={Stergios Tsiafoulis and Vasilios Zorkadis and Elias Pimenidis}, title={Maximum Entropy Oriented Anonymization Algorithm for Privacy Preserving Data Mining}, proceedings={Global Security, Safety and Sustainability \& e-Democracy. 7th International and 4th e-Democracy, Joint Conferences, ICGS3/e-Democracy 2011, Thessaloniki, Greece, August 24-26, 2011, Revised Selected Papers}, proceedings_a={ICGS3 \& E-DEMOCRACY}, year={2012}, month={10}, keywords={Privacy preservation maximum entropy anonymity -anonymity ℓ-diversity -closeness maximum entropy (SOMs) neural-network clustering}, doi={10.1007/978-3-642-33448-1_2} }
- Stergios Tsiafoulis
Vasilios Zorkadis
Elias Pimenidis
Year: 2012
Maximum Entropy Oriented Anonymization Algorithm for Privacy Preserving Data Mining
ICGS3 & E-DEMOCRACY
Springer
DOI: 10.1007/978-3-642-33448-1_2
Abstract
This work introduces a new concept that addresses the problem of preserving privacy when anonymising and publishing personal data collections. In particular, a maximum entropy oriented algorithm to protect sensitive data is proposed. As opposed to anonymity, ℓdiversity and closeness, the proposed algorithm builds equivalence classes with possibly uniformly distributed sensitive attribute values, probably by means of noise, and having as a lower limit the entropy of the distribution of the initial data collection, so that background information cannot be exploited to successfully attack the privacy of data subjects data refer to. Furthermore, existing privacy and information loss related metrics are presented, as well as the algorithm implementing the maximum entropy anonymity concept. From a privacy protection perspective, the achieved results are very promising, while the suffered information loss is limited.