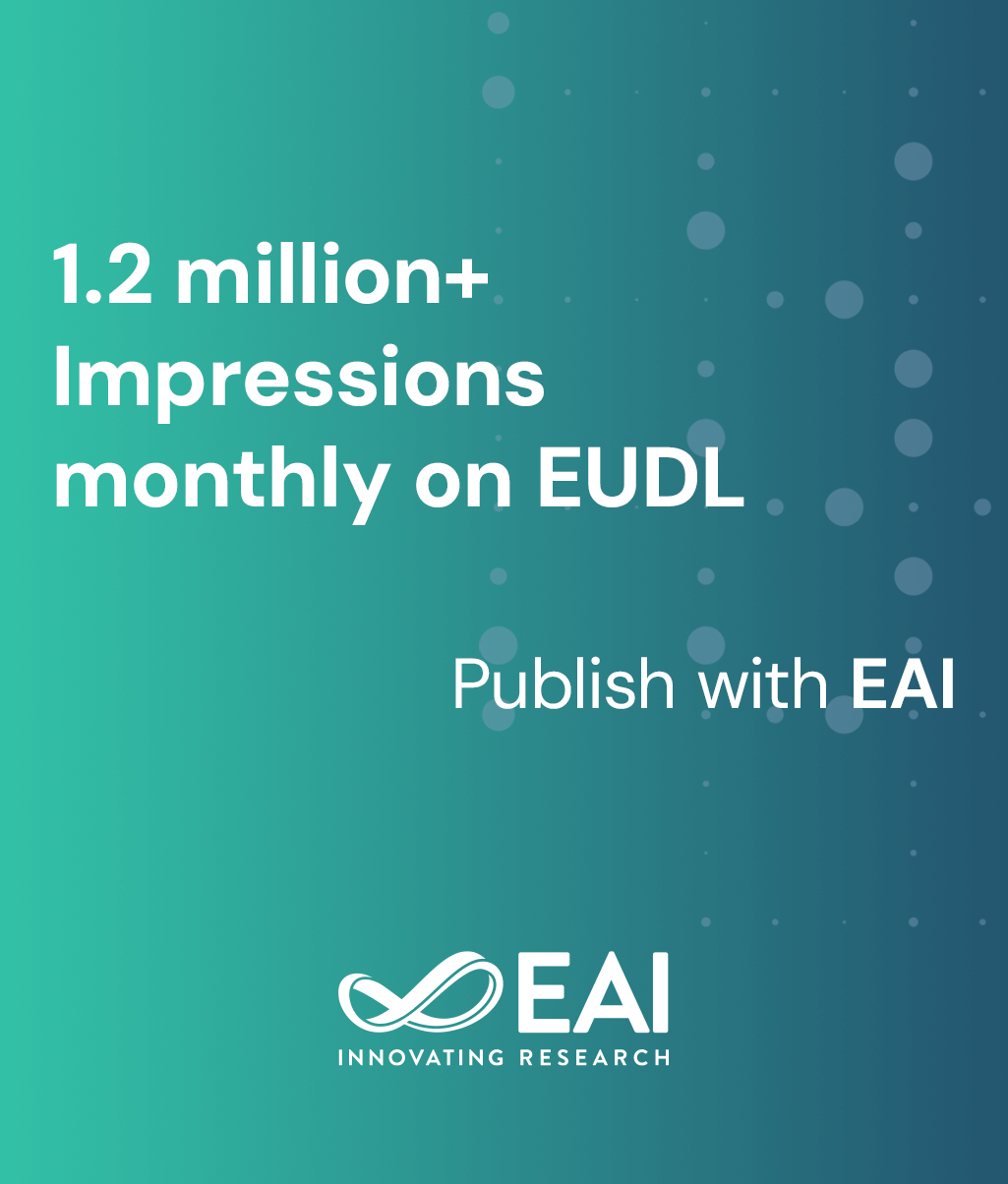
Research Article
Contribution of Spatio-temporal Intensity Variation to Bottom-Up Saliency
@INPROCEEDINGS{10.1007/978-3-642-32615-8_44, author={Eleonora Vig and Michael Dorr and Erhardt Barth}, title={Contribution of Spatio-temporal Intensity Variation to Bottom-Up Saliency}, proceedings={Bio-Inspired Models of Network, Information, and Computing Systems. 5th International ICST Conference, BIONETICS 2010, Boston, USA, December 1-3, 2010, Revised Selected Papers}, proceedings_a={BIONETICS}, year={2012}, month={10}, keywords={video saliency eye movements intrinsic dimension structure tensor natural dynamic scenes}, doi={10.1007/978-3-642-32615-8_44} }
- Eleonora Vig
Michael Dorr
Erhardt Barth
Year: 2012
Contribution of Spatio-temporal Intensity Variation to Bottom-Up Saliency
BIONETICS
Springer
DOI: 10.1007/978-3-642-32615-8_44
Abstract
We investigate the contribution of local spatio-temporal variation of image intensity to saliency. To measure different types of variation, we use the geometrical invariants of the structure tensor. With a video represented in spatial axes and and temporal axis , the -dimensional structure tensor can be evaluated for different combinations of axes (2D and 3D) and also for the (degenerate) case of only one axis. The resulting features are evaluated on several spatio-temporal scales in terms of how well they can predict eye movements on complex videos. We find that a 3D structure tensor is optimal: the most predictive regions of a movie are those where intensity changes along all spatial and temporal directions. Among two-dimensional variations, the axis pair , which is sensitive to horizontal translation, outperforms and by a large margin, and is even superior in prediction to two baseline models of bottom-up saliency.