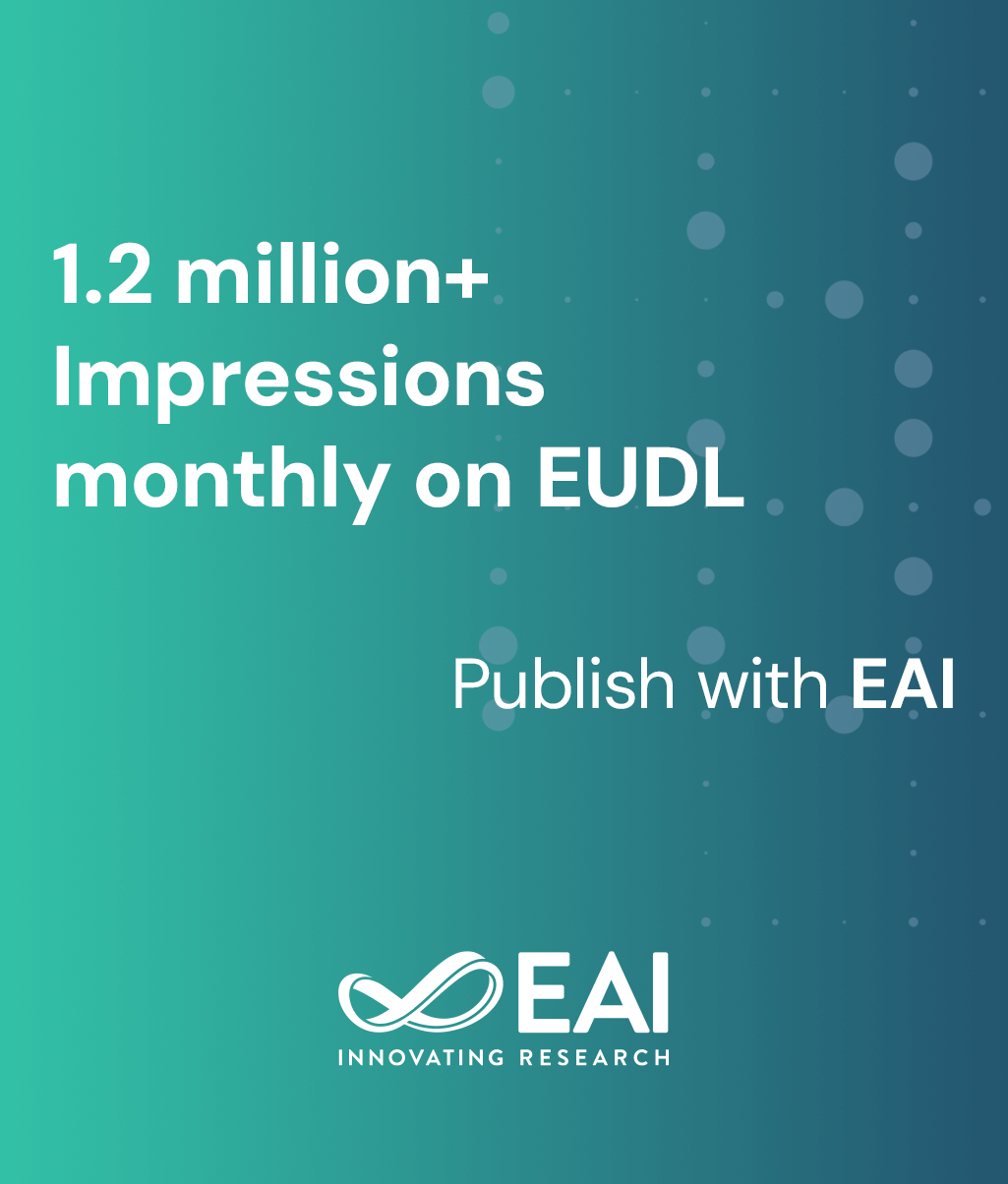
Research Article
Defense against Spectrum Sensing Data Falsification Attacks in Cognitive Radio Networks
@INPROCEEDINGS{10.1007/978-3-642-31909-9_9, author={Chowdhury Hyder and Brendan Grebur and Li Xiao}, title={Defense against Spectrum Sensing Data Falsification Attacks in Cognitive Radio Networks}, proceedings={Security and Privacy in Communication Networks. 7th International ICST Conference, SecureComm 2011, London, UK, September 7-9, 2011, Revised Selected Papers}, proceedings_a={SECURECOMM}, year={2012}, month={10}, keywords={Cognitive Radio Network SSDF attack 802.22}, doi={10.1007/978-3-642-31909-9_9} }
- Chowdhury Hyder
Brendan Grebur
Li Xiao
Year: 2012
Defense against Spectrum Sensing Data Falsification Attacks in Cognitive Radio Networks
SECURECOMM
Springer
DOI: 10.1007/978-3-642-31909-9_9
Abstract
IEEE 802.22 is the first standard based on the concept of cognitive radio. It recommends collaborative spectrum sensing to avoid the unreliability of individual spectrum sensing while detecting primary user signals. However, it opens an opportunity for attackers to exploit the decision making process by sending false reports. In this paper, we address security issues regarding distributed node sensing in the 802.22 standard and discuss how attackers can modify or manipulate their sensing result independently or collaboratively. This problem is commonly known as spectrum sensing data falsification (SSDF) attack or Byzantine attack. To counter the different attacking strategies, we propose a reputation based clustering algorithm that does not require prior knowledge of attacker distribution or complete identification of malicious users. We compare the performance of our algorithm against existing approaches across a wide range of attacking scenarios. Our proposed algorithm displays a significantly reduced error rate in decision making compared to current methods. It also identifies a large portion of the attacking nodes and greatly minimizes the false detection rate of honest nodes.