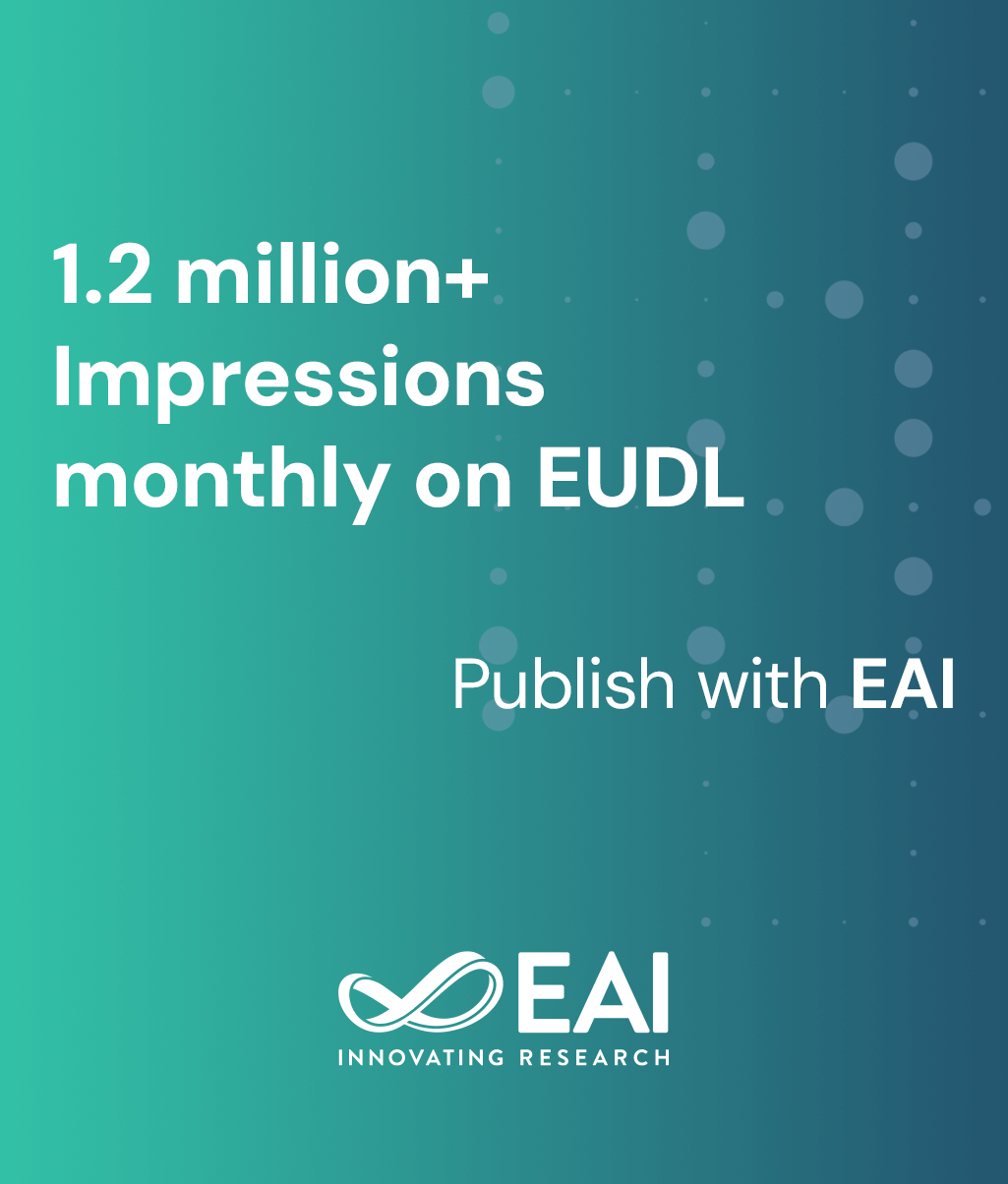
Research Article
Identifying Chronic Disease Complications Utilizing State of the Art Data Fusion Methodologies and Signal Processing Algorithms
@INPROCEEDINGS{10.1007/978-3-642-29734-2_35, author={John Gialelis and Petros Chondros and Dimitrios Karadimas and Sofia Dima and Dimitrios Serpanos}, title={Identifying Chronic Disease Complications Utilizing State of the Art Data Fusion Methodologies and Signal Processing Algorithms}, proceedings={Wireless Mobile Communication and Healthcare. Second International ICST Conference, MobiHealth 2011, Kos Island, Greece, October 5-7, 2011. Revised Selected Papers}, proceedings_a={MOBIHEALTH}, year={2012}, month={10}, keywords={patient monitoring algorithms data fusion}, doi={10.1007/978-3-642-29734-2_35} }
- John Gialelis
Petros Chondros
Dimitrios Karadimas
Sofia Dima
Dimitrios Serpanos
Year: 2012
Identifying Chronic Disease Complications Utilizing State of the Art Data Fusion Methodologies and Signal Processing Algorithms
MOBIHEALTH
Springer
DOI: 10.1007/978-3-642-29734-2_35
Abstract
In this paper a methodology for identifying patient’s chronic disease complications is proposed. This methodology consists of two steps: a. application of wavelet algorithms on ECG signal in order to extract specific features and b. fusion of the extracted information from the ECG signal with information from other sensors (i.e., body temperature, environment temperature, sweating index, etc.) in order to assess the health state of a monitoring patient. Therefore, the objective of this methodology is to derive semantically enriched information by discovering abnormalities at one hand detect associations and inter-dependencies among the signals at the other hand and finally highlight patterns and provide configuration rulesets for an intelligent local rule engine. The added value of the semantic enrichment process refers to the discovery of specific features and meaningful information with respect to the personalized needs of each patient.