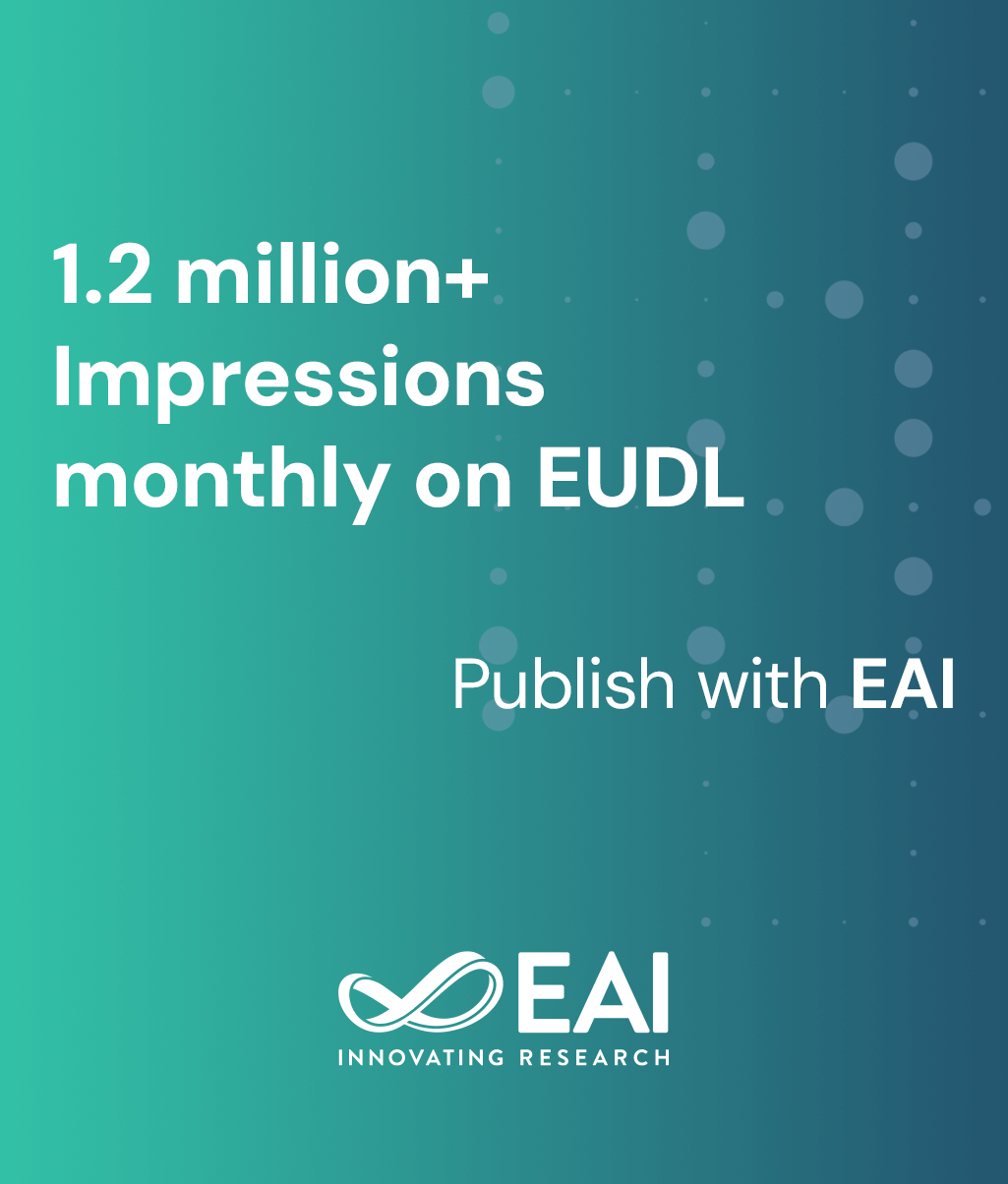
Research Article
SensOrchestra: Collaborative Sensing for Symbolic Location Recognition
@INPROCEEDINGS{10.1007/978-3-642-29336-8_11, author={Heng-Tze Cheng and Feng-Tso Sun and Senaka Buthpitiya and Martin Griss}, title={SensOrchestra: Collaborative Sensing for Symbolic Location Recognition}, proceedings={Mobile Computing, Applications, and Services. Second International ICST Conference, MobiCASE 2010, Santa Clara, CA, USA, October 25-28, 2010, Revised Selected Papers}, proceedings_a={MOBICASE}, year={2012}, month={10}, keywords={Collaborative sensing mobile phone sensing localization context-awareness context-based advertising}, doi={10.1007/978-3-642-29336-8_11} }
- Heng-Tze Cheng
Feng-Tso Sun
Senaka Buthpitiya
Martin Griss
Year: 2012
SensOrchestra: Collaborative Sensing for Symbolic Location Recognition
MOBICASE
Springer
DOI: 10.1007/978-3-642-29336-8_11
Abstract
Symbolic location of a user, like a store name in a mall, is essential for context-based mobile advertising. Existing fingerprint-based localization using only a single phone is susceptible to noise, and has a major limitation in that the phone has to be held in the hand at all times. In this paper, we present SensOrchestra, a collaborative sensing framework for symbolic location recognition that groups nearby phones to recognize ambient sounds and images of a location collaboratively. We investigated audio and image features, and designed a classifier fusion model to integrate estimates from different phones. We also evaluated the energy consumption, bandwidth, and response time of the system. Experimental results show that SensOrchestra achieved 87.7% recognition accuracy, which reduces the error rate of single-phone approach by 2X, and eliminates the limitations on how users carry their phones. We believe general location or activity recognition systems can all benefit from this collaborative framework.